DOI:
https://doi.org/10.14483/2256201X.20898Publicado:
01-01-2024Número:
Vol. 27 Núm. 1 (2024): Enero-junioSección:
Artículos de investigación científica y tecnológicaBiomass Storage Potential and Improvement in Soil Properties under Different Bamboo Plantations in the Terai Region of Central Himalaya
Almacenamiento de biomasa y mejora del suelo en plantaciones de bambú en la región de Terai en el Himalaya central
Palabras clave:
Bamboo species, nutrient uptake, aboveground biomass, soil microbial population, soil properties, Terai region (en).Palabras clave:
Especies de bambú, absorción de nutrientes, biomasa aérea, población microbiana del suelo, propiedades del suelo, región de Terai (es).Descargas
Referencias
Akinlabi, E. T., Anane-Fenin, K., & Akwada, D. R. (2017). Bamboo: The multipurpose plant. Springer. DOI: https://doi.org/10.1007/978-3-319-56808-9
Alemayehu, A., Mulatu, Y., Eshete, N., & Terefe, M. (2015). Growth performance and biomass accumulation of four different introduced bamboo species in South-Western Ethiopia. Growth, 5(3), 5-10.
Arce, J. J. C. (2019). Forests, inclusive and sustainable economic growth and employment. United Nations.
Arunachalam, A., & Arunachalam, K. (2002). Evaluation of bamboos in eco-restoration of ‘jhum’fallows in Arunachal Pradesh: Ground vegetation, soil and microbial biomass. Forest Ecology and Management, 159(3), 231-239. https://doi.org/10.1016/S0378-1127(01)00435-2 DOI: https://doi.org/10.1016/S0378-1127(01)00435-2
Bai, W., Kong, L., & Guo, A. (2013). Effects of physical properties on electrical conductivity of compacted lateritic soil. Journal of Rock Mechanics and Geotechnical Engineering, 5(5), 406-411. https://doi.org/10.1016/j.jrmge.2013.07.003 DOI: https://doi.org/10.1016/j.jrmge.2013.07.003
Benbi, D. K., Brar, K., Toor, A. S., & Singh P. (2015). Total and labile pools of soil organic carbon in cultivated and undisturbed soils in northern India. Geoderma, 237, 149-158. https://doi.org/10.1016/j.geoderma.2014.09.002 DOI: https://doi.org/10.1016/j.geoderma.2014.09.002
Blake, G. R., & Hartge, K. H. (1986). Methods of soil analysis. American Society of Agronomy.
Bower, C. A., & Wilcox, L. V. (1965). Methods of soil analysis. American Society of Agronomy.
Cao, S. X., Chen, L., Shankman, D., Wang, C. M., Wang, X. B., & Zhang, H. (2011) Excessive reliance on afforestationin China’s arid and semi-arid regions, Lessons in ecological restoration. Earth Science Review, 104, 240-245. https://doi.org/10.1016/j.earscirev.2010.11.002 DOI: https://doi.org/10.1016/j.earscirev.2010.11.002
Chang, E. H., Tian, G., Shiau, Y. J., Chen, T. H., & Chiu, C. Y. (2019). Influence of thorny bamboo plantations on soil microbial biomass and community structure in subtropical badland soils. Forests, 10(10), 854. https://doi.org/10.3390/f10100854 DOI: https://doi.org/10.3390/f10100854
Chen, X. (2021). Variations in patterns of internode and branch lengths for several bamboo species. Plant Biosystems, 155(6), 1088-1099. https://doi.org/10.1080/11263504.2020.1829729 DOI: https://doi.org/10.1080/11263504.2020.1829729
Chen, M., Lin, G., Ramakrishnan, M., Fei, Z., Kunnummal, K. V., Yulong, D., & Chen, J. (2022). Rapid growth of Moso bamboo (Phyllostachys edulis): Cellular roadmaps, transcriptome dynamics, and environmental factors. The Plant Cell, 34(10), 3577-3610. https://doi.org/ 10.1093/plcell/koac193 DOI: https://doi.org/10.1093/plcell/koac193
Chhonkar, P. K., Bhadraray, S., Patra, A. K., & Purakayastha T. J. (2007). Experiments in soil biology and biochemistry. Westville Publishing House.
Danielson, R. E., & Southerland, P. L. (1986). Porosity. American Society of Agronomy, Soil Science Society of America. DOI: https://doi.org/10.2136/sssabookser5.1.2ed.c18
Dong, L., & Kou, M. (2022). Soil Aggregate stability and carbon density in three plantations in the Loess Plateau, China. Forests, 13(7), 1096. https://doi.org/10.3390/f13071096 DOI: https://doi.org/10.3390/f13071096
El-Amin, E. A., Diab, I. E., & Ibrahim, S. (2001). Influence of Eucalyptus cover on some physical and chemical properties of a soil in Sudan. Communications in Soil Science and Plant Analysis, 32(13-14), 2267-2278. https://doi.org/10.1081/CSS-120000282 DOI: https://doi.org/10.1081/CSS-120000282
Farooq, T. H., Shakoor, A., Wu, X., Li, Y., Rashid, M. H. U., Zhang, X., & Yan, W. (2021). Perspectives of plantation forests in the sustainable forest development of China. Forest-Biogeosciences and Forestry, 14(2), 166-174. https://doi.org/10.3832/ifor3551-014 DOI: https://doi.org/10.3832/ifor3551-014
Franklin, D. C., Prior, L. D., Hogarth, N. J., & McMahon, C. R. (2010). Bamboo, fire and flood: Consequences of disturbance for the vegetative growth of a clumping, clonal plant. Plant Ecology, 208(2), 319-332. https://doi.org/10.1007/s11258-009-9709-x DOI: https://doi.org/10.1007/s11258-009-9709-x
Fu, Y., Horton, R., & Heitman J. (2021). Estimation of soil water retention curves from soil bulk electrical conductivity and water content measurements. Soil and Tillage Research, 209, 104948. https://doi.org/10.1016/j.still.2021.104948 DOI: https://doi.org/10.1016/j.still.2021.104948
Gaikwad, A. S., Thite, M., & Rananavare, S. I. A. (2021). Effect of different bamboo species on growth attributing characters grown on Entisol of semi-arid climate. The Pharma Innovation Journal, 10(12), 1980-1985. https://doi.org/10.22271/tpi.2021.v10.i12Saa.9910 DOI: https://doi.org/10.22271/tpi.2021.v10.i12Saa.9910
Goh, Y., Yap, S. P., & Tong, T. Y. (2020). Bamboo: The emerging renewable material for sustainable construction. Encyclopedia of Renewable and Sustainable Materials, 2, 365-376. https://doi:org/10.1016/B978-0-12-803581-8.10748-9 DOI: https://doi.org/10.1016/B978-0-12-803581-8.10748-9
Guo, Z., Zhuang, M., Yang, L., Li, Y., Wu, S., & Chen, S. (2021). Differentiated mineral nutrient management in two bamboo species under elevated CO2 environment. Journal of Environmental Management, 279, 111600. https://doi.org/10.1016/j.jenvman.2020.111600 DOI: https://doi.org/10.1016/j.jenvman.2020.111600
Hao, M., Zhang, J., Meng, M., Chen, H. Y., Guo, X., Liu, S., & Ye, L. (2019). Impacts of changes in vegetation on saturated hydraulic conductivity of soil in subtropical forests. Scientific Reports, 9(1), 8372. https://doi.org/10.1038/s41598-019-44921-w DOI: https://doi.org/10.1038/s41598-019-44921-w
Hassan, N. H. M., Abdullah, N., Kelanda, D. N. A., & Perumal, M. (2022). Early field growth performance of ten selected bamboo taxa: The case study of Sabal bamboo pilot project in Sarawak, Malaysia. Biodiversitas, 23(6), 2882-2892. https://doi.org/10.13057/biodiv/d230614 DOI: https://doi.org/10.13057/biodiv/d230614
Iyer, R. (2019). Sustainability-based evaluation of bamboo harvest in Pachgaon [Doctoral dissertation, Department of Biology, IISRE]. http://dr.iiserpune.ac.in:8080/xmlui/bitstream/handle/123456789/3018/Rahul%20_Iyer_20141154_Thesis_final.pdf?sequence=1&isAllowed=y
Jackson, M. L. (1958). Soil chemical analysis. Asia Publishing House.
Jackson, M. L. (1967). Soil chemical analysis. Prentice Hall.
Kanwal, M. S. (2011). Influence of different bamboo species on growth, carbon sequestration potential and soil properties in tarai region of northern india [Master’s thesis, G. B. Pant University of Agriculture and Technology, Department of Agronomy.
Kaushal, R., Singh, I., Thapliyal, S. D., Gupta, A. K., Mandal, D., Tomar, J. M. S., & Durai, J. (2020 a). Rooting behaviour and soil properties in different bamboo species of Western Himalayan Foothills, India. Scientific Reports, 10(1), 4966. https://doi.org/10.1038/s41598-020-61418-z DOI: https://doi.org/10.1038/s41598-020-61418-z
Kaushal, R., Tewari, S., Banik, R. L., Thapliyal, S. D., Singh, I., Reza, S., & Durai, J. (2020 b). Root distribution and soil properties under 12-year old sympodial bamboo plantation in Central Himalayan Tarai Region, India. Agroforestry Systems, 94, 917-932. https://doi.org/10.1007/s10457-019-00459-4 DOI: https://doi.org/10.1007/s10457-019-00459-4
Kaushal, R., Islam, S., Tewari, S., Tomar, J. M. S., Thapliyal, S., Madhu, M., & Durai, J. (2022). An allometric model-based approach for estimating biomass in seven Indian bamboo species in western Himalayan foothills, India. Scientific Reports, 12(1), 7527. https://doi.org/10.1038/s41598-022-11394-3 DOI: https://doi.org/10.1038/s41598-022-11394-3
Kim, C., Baek, G., Yoo, B. O., Jung, S. Y., & Lee, K. S. (2018). Regular fertilization effects on the nutrient distribution of bamboo components in a moso bamboo (Phyllostachys pubescens (Mazel) Ohwi) stand in South Korea. Forests, 9(11), 671. https://doi.org/10.3390/f9110671 DOI: https://doi.org/10.3390/f9110671
Kittur, B. H., Sudhakara, K., Mohan Kumar, B., Kunhamu, T. K., & Sureshkumar, P. (2016). Bamboo based agroforestry systems in Kerala, India: performance of turmeric (Curcuma longa L.) in the subcanopy of differentially spaced seven year-old bamboo stand. Agroforestry Systems, 90, 237-250. https://doi.org/ 10.1007/s10457-015-9849-z DOI: https://doi.org/10.1007/s10457-015-9849-z
Kodešová, R., Kodeš, V., Žigová, A., & Šimůnek, J. (2006). Impact of plant roots and soil organisms on soil micromorphology and hydraulic properties. Biologica, 61(19), 339-343. https://doi.org/10.2478/s11756-006-0185-7 DOI: https://doi.org/10.2478/s11756-006-0185-7
Kumar, B. M., Rajesh, G., & Sudheesh, K. G. (2006). Aboveground biomass production and nutrient uptake of thorny bamboo [Bambusa bambos (L.) Voss] in the homegardens of Thrissur, Kerala. Journal of Tropical Agriculture, 43, 51-56.
Kumar, R., Thangaraju, M. M., Kumar, M., Thul, S. T., Pandey, V. C., Yadav, S., & Kumar, S. (2021). Ecological restoration of coal fly ash–dumped area through bamboo plantation. Environmental Science and Pollution Research, 28, 33416-33432. https://doi.org/ 10.1007/s11356-021-12995-7 DOI: https://doi.org/10.1007/s11356-021-12995-7
Kumar, P. S., Shukla, G., Nath, A. J., & Chakravarty, S. (2022). Soil properties, litter dynamics and biomass carbon storage in three-bamboo species of Sub-Himalayan region of Eastern India. Water, Air, & Soil Pollution, 233(1), 12. https://doi.org/10.1007/s11270-021-05477-6 DOI: https://doi.org/10.1007/s11270-021-05477-6
Kumari, Y., & Bhardwaj, D. R. (2017). Distribution of nutrition elements in different bamboos in bamboo based agroforestry plantation: A comparative study. Journal of Pharmacognosy and Phytochemistry, 6(3), 556-561.
Kumari, Y., & Bhardwaj, D. R. (2019). Bioproduction and nutrient cycling in seven bamboo species in subtropical Indian Himalayas. Journal of Tree Sciences, 38(2), 1-12. http://dx.doi.org/10.5958/2455-7129.2019.00012.8 DOI: https://doi.org/10.5958/2455-7129.2019.00012.8
Lei, Z., Yu, D., Zhou, F., Zhang, Y., Yu, D., Zhou, Y., & Han, Y. (2019). Changes in soil organic carbon and its influencing factors in the growth of Pinus sylvestris var. mongolica plantation in Horqin Sandy Land, Northeast China. Scientific Reports, 9(1), 16453. https://doi.org/10.1038/s41598-019-52945-5 DOI: https://doi.org/10.1038/s41598-019-52945-5
Li, C., Cai, Y., Xiao, L., Gao, X., Shi, Y., Zhou, Y., & Zhou, G. (2021). Rhizome extension characteristics, structure and carbon storage relationships with culms in a 10-year moso bamboo reforestation period. Forest Ecology and Management, 498, 119556. https://doi.org/10.1016/j.foreco.2021.119556 DOI: https://doi.org/10.1016/j.foreco.2021.119556
Liu, R., Yang, X., Gao, R., Hou, X., Huo, L., Huang, Z., & Cornelissen, J. H. (2021). Allometry rather than abiotic drivers explains biomass allocation among leaves, stems and roots of Artemisia across a large environmental gradient in China. Journal of Ecology, 109(2), 1026-1040. https://doi.org/10.1111/1365-2745.13532 DOI: https://doi.org/10.1111/1365-2745.13532
Lobovikov, M., Paudel, S., Ball, L., Piazza, M., Guardia, M., Wu, J., & Ren, H. (2007). World bamboo resources: A thematic study prepared in the framework of the global forest resources assessment 2005 (No. 18). Food & Agriculture Organization.
Luo, X., Keenan, T. F., Chen, J. M., Croft, H., Colin Prentice, I., Smith, N. G., & Zhang, Y. (2021). Global variation in the fraction of leaf nitrogen allocated to photosynthesis. Nature Communications, 12(1), 4866. https://doi.org/10.1038/s41467-021-25163-9. DOI: https://doi.org/10.1038/s41467-021-25163-9
Mensah, S., Kakaï, R. G., & Seifert, T. (2016). Patterns of biomass allocation between foliage and woody structure: the effects of tree size and specific functional traits. Annals of Forest Research, 59(1), 49-60. https://doi.org/ 10.15287/afr.2016.458 DOI: https://doi.org/10.15287/afr.2016.458
Nawaz, M. F., Bourrie, G., & Trolard, F. (2013). Soil compaction impact and modelling. A review. Agronomy for Sustainable Development, 33, 291-309. https://doi.org/10.1007/s13593-011-0071-8 DOI: https://doi.org/10.1007/s13593-011-0071-8
Noguchi, M., & Yoshida, T. (2005). Factors influencing the distribution of two co-occurring dwarf bamboo species (Sasa kurilensis and S. senanensis) in a conifer-broadleaved mixed stand in northern Hokkaido. Ecological Research, 20, 25-30. https://doi.org/10.1007/s11284-004-0009-6. DOI: https://doi.org/10.1007/s11284-004-0009-6
Oli, B. N., & Kandel, C. M. (2005). Biomass estimation of Bambusa nutans subspecies cupulata grown at Eastern Terai, Nepal. Banko Janakari, 15(2), 34-37. https://doi.org/10.3126/banko.v15i2.349 DOI: https://doi.org/10.3126/banko.v15i2.349
Ovington, J. D. (1965). Organic production, turnover and mineral cycling in woodlands. Biological Reviews, 40(3), 295-336. DOI: https://doi.org/10.1111/j.1469-185X.1965.tb00805.x
Pandey, C. S. (2019). Influence of tree cropping systems on physical properties of salt affected soil. International Journal of Current Microbiology and Applied Science, 8(4), 739-747. https://doi.org/10.20546/ijcmas.2019.804.080 DOI: https://doi.org/10.20546/ijcmas.2019.804.080
Pattanaik, S., & Hall, J. B. (2014). Patterns of morphometric variability in Dendrocalamus hamiltonii Munro populations across East Khasi Hills, Northeast India. Indian Forester, 140(9), 868-874.
Patra, S., Kaushal, R., Singh, D., Kumar, R., Gadedjisso-Tossou, A., & Durai, J. (2022). Surface soil hydraulic conductivity and macro-pore characteristics as affected by four bamboo species in North-Western Himalaya, India. Ecohydrology & Hydrobiology, 22(1), 188-196. https://doi.org/10.1016/j.ecohyd.2021.08.012 DOI: https://doi.org/10.1016/j.ecohyd.2021.08.012
Puangchit, L., Hnin, S. M., & Sungkaew, S. (2019). Allometric equations for estimating the aboveground biomass of a 14-Year-old bamboo plantation at moeswe research station, Myanmar. Journal of Tropical Forest Research, 3(1), 1-19.
Qian, Z., Sun, X., Gao, J., & Zhuang, S. (2021). Effects of bamboo (Phyllostachys praecox) cultivation on soil nitrogen fractions and mineralization. Forests, 12(8), 1109. https://doi.org/10.3390/f12081109 DOI: https://doi.org/10.3390/f12081109
Qiao, Y., Miao, S., Yue, S., Wu, H., & Han, X. (2016). How 23-year continuous soybean cultivation led to more SOC and thermal energy stored in Mollisol micro-aggregates. Polish Journal of Environmental Studies, 25(3), 1215-1221. https://doi.org/10.15244/pjoes/61628 DOI: https://doi.org/10.15244/pjoes/61628
Robinson, D. A., Thomas, A., Reinsch, S., Lebron, I., Feeney, C. J., Maskell, L. C., & Cosby, B. J. (2022). Analytical modelling of soil porosity and bulk density across the soil organic matter and land-use continuum. Scientific Reports, 12(1), 7085. https://doi.org/10.1038/s41598-022-11099-7 DOI: https://doi.org/10.1038/s41598-022-11099-7
Seobi, T., Anderson, S. H., Udawatta, R. P., & Gantzer, C. J. (2005). Influence of grass and agroforestry buffer strips on soil hydraulic properties for an Albaqualf. Soil Science Society of America Journal, 69(3), 893-901.https://doi.org/10.2136/sssaj2004.0280 DOI: https://doi.org/10.2136/sssaj2004.0280
Selassie, Y. G., & Ayanna, G. (2013). Effects of different land use systems on selected physico-chemical properties of soils in Northwestern Ethiopia. Journal of Agricultural Science, 5(4), 112. https://doi.org/10.5539/jas.v5n4p112 DOI: https://doi.org/10.5539/jas.v5n4p112
Shanmughavel, P., & Francis, K. (2003). Biomass accumulation and nutrient distribution in Dendrocalamus hamiltonii [Conference presentation]. XII World Forestry Congress, Quebec, Canada.
Sharma, M. L., & Nirmala, C. (2015). Bamboo diversity of India: An update [Conference presentation]. 10th World Bamboo Congress, Seoul, South Korea.
Shiau, Y. J., Wang, H. C., Chen, T. H., Jien, S. H., Tian, G., & Chiu, C. Y. (2017). Improvement in the biochemical and chemical properties of badland soils by thorny bamboo. Scientific Reports, 7(1), 40561. https://doi.org/10.1038/srep40561. DOI: https://doi.org/10.1038/srep40561
Silva, L. N., Freer-Smith, P., & Madsen, P. (2019). Production, restoration, mitigation: a new generation of plantations. New Forests, 50(2), 153-168. https://doi.org/10.1007/s11056-018-9644-6 DOI: https://doi.org/10.1007/s11056-018-9644-6
Singh, J., Sharma, R., Dhakad, A. K., & Chauhan, S. K. (2018). Defining growth, quality and biomass production of different bamboo species in central plains of Punjab. Journal of Pharmacognosy and Phytochemistry, 7(5), 1328-1332.
Singh, K. A., & Kochhar, S. K. (2005). Effect of clump density/spacing on the productivity and nutrient uptake in Bambusa pallida and the changes in soil properties. Journal of Bamboo Rattan, 4(4), 323-334. DOI: https://doi.org/10.1163/156915905775008354
Singh, A. N., & Singh, J. S. (2006). Experiments on ecological restoration of coal mine spoil using native trees in a dry tropical environment, India: A synthesis. New Forests, 31, 25-39. https://doi.org/10.1007/s11056-004-6795-4 DOI: https://doi.org/10.1007/s11056-004-6795-4
Singh, K. A., & Rai, A. K. (2012). Nutrient accumulation, distribution and use efficiency in different bamboo plant species in north-eastern hills region of India. Indian Journal of Agricultural Sciences, 82(2), 134. DOI: https://doi.org/10.56093/ijas.v82i2.15288
Subbaiah, B. V., & Asija, G. L. (1956). A rapid method for estimation of available N in soil. Current Science, 25, 259-260.
Tian, G., Justicia, R., Coleman, D. C., & Carroll, C. R. (2007). Assessment of soil and plant carbon levels in two ecosystems (woody bamboo and pasture) in montane Ecuador. Soil Science, 172(6), 459-468. https://doi.org/10.1097/ss.0b013e31804fa21a DOI: https://doi.org/10.1097/ss.0b013e31804fa21a
Trivedi, P., Leach, J. E., Tringe, S. G., Sa, T., & Singh, B. K. (2020). Plant-microbiome interactions: From community assembly to plant health. Nature Reviews Microbiology, 18(11), 607-621. https://doi.org/10.1038/s41579-020-0412-1 DOI: https://doi.org/10.1038/s41579-020-0412-1
Tu, Z., Chen, L., Yu, X., & Zheng, Y. (2013). Effect of bamboo plantation on rhizosphere soil enzyme and microbial activities in coastal ecosystem. Journal of Food, Agriculture and Environment, 2(3), 2333-2338.
Udawatta, R. P., Kremer, R. J., Garrett, H. E., & Anderson, S. H. (2009). Soil enzyme activities and physical properties in a watershed managed under agroforestry and row-crop systems. Agriculture, Ecosystems & Environment, 131(1-2), 98-104. https://doi.org/10.1016/j.agee.2008.06.001 DOI: https://doi.org/10.1016/j.agee.2008.06.001
Walkley, A., & Black, I. A. (1934). An examination of the Degtjareff method for determining soil organic matter, and a proposed modification of the chromic acid titration method. Soil Science, 37(1), 29-38. DOI: https://doi.org/10.1097/00010694-193401000-00003
Wang, Y., Qiao, G., Xu, J., Jin, K., Fan, M., Ding, Y., & Zhuo, R. (2022). Anatomical characteristics and variation mechanisms on the thick-walled and dwarfed culm of Shidu bamboo (Phyllostachys nidularia f. farcta). Frontiers in Plant Science, 13, 1-11. https://doi.org/10.3389/fpls.2022.876658 DOI: https://doi.org/10.3389/fpls.2022.876658
Watanabe, F. S., & Olsen, S. R. (1965). Test of an ascorbic acid method for determining phosphorus in water and NaHCO3 extracts from soil. Soil Science Society of America Journal, 29(6), 677-678. DOI: https://doi.org/10.2136/sssaj1965.03615995002900060025x
Wu, J., Xu, Q., Jiang, P., & Cao, Z. (2009). Dynamics and distribution of nutrition elements in bamboos. Journal of Plant Nutrition, 32(3), 489-501. https://doi.org/10.1080/01904160802679958 DOI: https://doi.org/10.1080/01904160802679958
Xie, J., Huang, X., Qi, J., Hse, C. Y., & Shupe, T. F. (2014). Effect of anatomical characteristics and chemical components on microwave-assisted liquefaction of bamboo wastes. BioResources, 9(1), 1-9. DOI: https://doi.org/10.15376/biores.9.1.231-240
Xue, L., Ren, H., Li, S., Leng, X., & Yao, X. (2017). Soil bacterial community structure and co-occurrence pattern during vegetation restoration in karst rocky desertification area. Frontiers in Microbiology, 8, 2377.https://doi.org/10.3389/fmicb.2017.02377. DOI: https://doi.org/10.3389/fmicb.2017.02377
Zhan, H., Zhang, L. Y., Niu, Z. H., Wang, C. M., & Wang, S. G. (2016). Chemical properties and fiber morphology of Dendrocalamus hamiltonii as potential pulp material. European Journal of Wood and Wood Products, 74, 273-276. https://doi.org/10.1007/s00107-015-0993-y DOI: https://doi.org/10.1007/s00107-015-0993-y
Zhang, Y. M., Yu, Y. L., & Ji, Y. W. (2013). Effect of thermal treatment on the physical and mechanical properties of Phyllostachys pubescen bamboo. European Journal of Wood and Wood Products, 71(1), 61-67. https://doi.org/ 10.1007/s00107-012-0643-6. DOI: https://doi.org/10.1007/s00107-012-0643-6
Zhang, M. M., Fan, S. H., Guan, F. Y., Yan, X. R., & Yin, Z. X. (2020). Soil bacterial community structure of mixed bamboo and broad-leaved forest based on tree crown width ratio. Scientific Reports, 10(1), 6522. https://doi.org/10.1038/s41598-020-63547-x DOI: https://doi.org/10.1038/s41598-020-63547-x
Zhao-Hua, L. I., Denich, M., & Borsch, T. (2005). Growth behavior of Phyllostachys nigra var. henonis (Bambusoideae) in Central China. Journal of Forestry Research, 16, 163-168.https://doi.org/10.1007/BF02856808. DOI: https://doi.org/10.1007/BF02856808
Zheng, Y., & Lin, X. (2020). Niche specialization and functional overlap of bamboo leaf and root microbiota. Frontiers in Microbiology, 11, 571159. https://doi.org/10.3389/fmicb.2020.571159 DOI: https://doi.org/10.3389/fmicb.2020.571159
Zhou, Z., & Shangguan, Z. (2007). Vertical distribution of fine roots in relation to soil factors in Pinus tabulaeformis Carr. forest of the Loess Plateau of China. Plant and Soil, 291, 119-129. https://doi.org/10.1007/s11104-006-9179-z DOI: https://doi.org/10.1007/s11104-006-9179-z
Cómo citar
APA
ACM
ACS
ABNT
Chicago
Harvard
IEEE
MLA
Turabian
Vancouver
Descargar cita
Visitas
Dimensions
PlumX
Descargas
Recibido: 25 de mayo de 2023; Aceptado: 20 de noviembre de 2023
Abstract
This study evaluates six bamboo species, i.e., Dendrocalamus hamiltonii, Bambusa nutans, Dendrocalamus asper, Bambusa bambos, Bambusa balcooa, and Dendrocalamus strictus, regarding their growth behavior, nutrient uptake, and effect on the soil properties of the Terai region. Various aboveground growth parameters exhibited significant variations with the maximum clump girth (8.60 m) and internodal length (35.37 cm) of B. nutans, which was also reported by the culm diameter (5.70 cm) of D. hamiltonii and the number of culms per clump (65) of D. strictus. The total aboveground biomass ranged from 51.14 Mg.ha-1 in D. asper to 362.56 Mg.ha-1 in D. hamiltonii. The most significant variation in soil properties was observed in the 0-40 cm soil layer. Under D. hamiltonii, the lowest soil bulk density (1.07 g.cm3; 1.21 g.cm3) and the maximum soil porosity (59.00%; 56.40%), soil organic carbon (1.54%; 0.72%), and available soil nitrogen (228.29 kg.ha-1; 173.73 kg.ha-1) were found the 0-40 and 40-80 cm soil layers. Furthermore, significant enhancements in soil microbial population were recorded. Thus, bamboo plantations have great potential to enhance the biomass generation and fertility quotient of fallow lands.
Keywords:
aboveground biomass, bamboo species, nutrient uptake, soil microbial population, soil properties, Terai region.Resumen
Este estudio evalúa seis especies de bambú, i.e., Dendrocalamus hamiltonii, Bambusa nutans, Dendrocalamus asper, Bambusa bambos, Bambusa balcooa y Dendrocalamus strictus, respecto a su comportamiento de crecimiento, absorción de nutrientes y efecto sobre las propiedades del suelo de la región de Terai. Varios parámetros de crecimiento sobre el suelo mostraron variaciones significativas con la circunferencia máxima del macizo (8.60 m) y la longitud internodal (35.37 cm) de B. nutans, lo cual también se reportó para diámetro del culmo (5.70 cm) de D. hamiltonii y el número de culmos por macizo (65) de D. strictus. La biomasa aérea total varió de 51.14 Mg.ha-1 en D. asper a 362.56 Mg.ha-1 en D. hamiltonii. La variación más significativa en las propiedades del suelo se observó en la capa de suelo de 0-40 cm. Bajo D. hamiltonii, la densidad aparente del suelo más baja (1.07 g.cm3; 1,21 g.cm3) y los máximos valores de porosidad del suelo (59 %; 56.40 %), carbono orgánico del suelo (1.54 %; 0.72%) y nitrógeno disponible del suelo (228.29 kg.ha-1; 173.73 kg.ha-1) se encontraron en las capas de 0-40 y 40-80 cm de profundidad. Además, se registró una mejora significativa en la población microbiana del suelo. Por lo tanto, las plantaciones de bambú tienen un gran potencial para mejorar la generación de biomasa y el cociente de fertilidad de las tierras en barbecho.
Palabras clave:
absorción de nutrientes, especies de bambú, biomasa aérea, población microbiana del suelo, propiedades del suelo, región de Terai.INTRODUCTION
The establishment of fast-growing plantations with short-rotation woody crops in marginal and degraded lands is globally prevalent and has been the focus of research at every national and international level (Silva et al., 2019). However, such plantations are not only emerging as the primary source for meeting the ever-increasing need for wood, bioenergy, and raw materials for a variety of forest-based industries (Arce, 2019), but also hold great potential to restore the productivity and biodiversity of the degraded ecosystems (Silva et al., 2019). Apart from bolstering the ecological functions of degraded lands, they also help to preserve dwindling natural forests (Farooq et al., 2021), reduce atmospheric CO2, and improve the socioeconomic status of local communities.
At a global level, bamboo is regarded as the most versatile, sustainable, and replenishable plant resource, with enormous economic, social, and environmental benefits and covering an area of around 37 million ha (Goh et al., 2020). Bamboo has multifaceted uses like food, fuel, fiber, and construction material, playing an integral role in the sustainable development of equatorial countries (Akinlabi et al., 2017). For a few decades, bamboo planting has become more popular around the world, with Asia accounting for 30% of all bamboo resources planted (Lobovikov et al., 2007). In India alone, around 136 bamboo species in 29 genera are prevalent, both in cultivated plantations as well as forest ecosystems, with maximum species diversity in the forest subgroups: 2B, 3B, and 3C (Sharma & Nirmala, 2015).
Different bamboo species have distinctive growth rates and biomass allocation patterns, and they require various environmental conditions to proliferate. The assessment and evaluation of the growth performance of bamboo species are integral to calculating their adaptive capacity, production potential, and nutrient transport during harvest (Alemayehu et al., 2015). Comparative studies on the growth behavior of the various bamboo species are integral for the selection for commercial plantations, agroforestry/social forestry programs, and plantations for ecosystem conservation activities, among other things.
Bamboo plantations play many ecologically beneficial and environmentally sustainable roles, i.e., in controlling soil erosion depletion, reclaiming degraded lands, water resource preservation, and climate change mitigation (Tu et al., 2013). Bamboo’s fast growth with thick foliage and dense fibrous interconnected root systems, which results in a thick litter layer on the soil, helps to conserve soil moisture, improve soil fertility, and act as a barrier to soil nutrient loss. The impact of bamboo on soil is species-specific and has a varied effect on soil physicochemical properties, with most species tending to improve soil aeration, bulk density, and soil microbial biomass due to the large root surface area and the secretion of root exudes in the rhizosphere (Liu et al., 2021). Therefore, the goal of this study is to understand the growth patterns, nutrient uptake, and effects on soil qualities of several bamboo species. We hypothesized that the aboveground growth parameters, nutrient allocation, and soil properties of bamboo are species-specific.
MATERIALS AND METHODS
Study area
This study was conducted in a 14-year-old bamboo plantation at the Agroforestry Research Center, G. B. Pant University of Agriculture and Technology, Pantnagar, Uttarakhand (29°03′73′′ N latitude, 79°45′25′′ E longitude, and 236 m altitude) during the 2021-2022 period. This area is well known as the Tarai region, a narrow strip located in the foothills of the Shivalik range of the Himalaya. The climate of the experimentation site is humid semi-tropical, with chilled winters and hot dry summers, falling in the Cwa category of the Koppen-Geiger system. During the research period, the mean maximum temperature reached 45 °C in May, and the average lowest temperature was 0.5 °C in January. Around 80-90% of the rainfall is received from the south-west monsoon in July-September, with a mean annual rainfall of 1405 mm. Alluvial materials with medium to moderately coarse textures make up soils of the Terai region, which are mostly influenced by tall vegetation and moderate to well-drained conditions. The soils are referred to as mollisols because they have mollic horizons and weakly developed mollic epipedons.
Experimental design
The plantation was established in 2005 with six bamboo species: Dendrocalamus hamiltonii, Bambusa nutans, Dendrocalamus asper, Bambusa bambos, Bambusa balcooa, and Dendrocalamus strictus. The bamboo stand was established with a 5 m × 5 m clump spacing in a randomized block statistical design with four replications. For each bamboo species, four plants were planted in each plot (block plantation), occupying an area of 10 m2. In total, there were 24 plots, covering a total area of 240 m2.
METHODOLOGY
Biomass estimation
A complete enumeration was carried out, counting the number of culms per clump with the help of the cross-stick method. Then, the circumference of the bamboo clump (i.e., clump girth) was determined at breast height (DBH at 1.37 m) using measuring tape. Three culms of different ages (1, 2, or 3 years old or above) from each replication (12 for each species) were selected based on their physiological appearance, i.e., the color of the culm, the presence of culm sheath. The length, diameter, number of nodes, and internodal length of selected culms were measured, which were destructively felled from ground level during December. To determine the fresh weight, branches were parted, and leaves were removed from the felled culms of each bamboo species. To calculate the culm, branch, and leaf biomass of various bamboo species, representative samples were taken from each bamboo species and then oven dried. The oven dry weight of the representative sample was then multiplied by the total number of culms. The total aboveground biomass was calculated by adding the leaf, branch, and culm biomass for each bamboo species.
Nutrient uptake
To estimate the nutrient uptake of the six bamboo species under study, the samples of culm, branches, and leaves from specimens of different ages (1, 2, or 3 years old or above) from each replication were collected and kept under sun, left to dry for 2-3 days. The samples were further dried for two days in a hot air oven at 65±5ο C and then ground to fine powder. After grinding, the samples passed through 0.5 mm sieve and were chemically analyzed for nitrogen (Jackson, 1967, i.e., modified KEL PLUS auto N-analyzer), phosphorus (vanado-molybdate method), and potassium (flame emission spectrophotometer). The following formulas were used to determine the nutrient (NPK) uptake by the culm, branch, and leaves of six bamboo species (Singh & Rai, 2012):
Soil physicochemical properties and microbial activities
The soil samples were collected randomly from between the rows of bamboo and from the plot devoid of bamboo (control plot) at three levels of soil depth, i.e., 0-40, 40-80, and 80-120 cm. A composite sample was then prepared for each soil depth, combining soils from three sampling sites and producing one sample per level from each plot. Following collection, the samples were air dried, ground, and sieved. The bulk density was estimated by using the core sampler technique (Blake & Hartge, 1986), and a steel measuring cylinder was used to gauge the particle density. Soil porosity was calculated via the method described by Danielson & Southerland (1986). The soil pH was measured with a 1:2.5 soil: water suspension ratio (Jackson, 1967), and the electrical conductivity of the soil was measured using a digital microprocessor-based electrical conductivity meter (Bower & Wilcox, 1965). The quick titration method was used to determine the amount of organic carbon in the soil (Walkley & Black, 1934). The alkaline potassium permanganate method was used to determine the available soil nitrogen (Subbaiah & Asija, 1956), the available phosphorus was estimated via Bray no. 1 extraction followed by the reduced molybdate blue color estimation method (Watanabe & Olsen, 1965), and the available potassium was determined by means of neutral normal ammonium acetate solution extraction followed by flame photometry (Jackson, 1958). Regarding the soil microbial population, the soil samples were collected from each plot at a depth of up to 20 cm and air dried, ground, and sieved. The serial dilution plate technique to soil samples from each plot (Chhonkar et al., 2007). As for fungi, Martin's Rose-Bengal medium was used, Thornton's medium for bacteria, and Ken-Knight and Munaier’s medium for actinomycetes.
Statistical analysis
A thorough statistical study was carried out, and the range of deviation was calculated using the standard error of the mean. A randomized complete block design with four replications was used to compare the treatment effect (bamboo species) on aboveground growth parameters, nutrient uptake, and soil physicochemical properties at different soil depths for the same bamboo species and at the same depth for different bamboo species and soil microbial population. A one-way analysis of variance (ANOVA) was performed using R (version 4.2.1) for Windows. To identify significant differences in the treatment means, a post hoc test, i.e., Tukey’s honest significant difference (HSD), was used at a 0.05 level of significance.
Figure 1: Layout of the experimental plot
RESULTS
Growth parameters and biomass
The post hoc analysis via Tukey’s HSD test (Figures 2 and 3) revealed that all the growth parameters of six bamboo species were significantly different (p<0.05), i.e., the clump girth (CG), culm length (CuL), culm diameter (CuD), number of culms per clump (NCu/Cl), number of nodes (NN), and internodal length (IL).
Figure 2: Variations in growth parameters: a) clump girth (m), b) number of culms, c) culm length (m), d) culm diameter (cm), e) number of nodes, and f) internode length (cm) for six bamboo species. Means followed by different letters are significantly different (p<0.05). The error bar signifies the mean standard deviation
Figure 3: Variations in above ground biomass a) leaf biomass, b) branch biomass, c) culm biomass, and d) total aboveground biomass for six bamboo species. Means followed by different letters are significantlydifferent (p<0.05). The error bar signifies the mean standard deviation
The highest CG (8.60 m), CuL (15.68 m), NN (50), and IL (35.37 cm) were observed in B. nutans. However, the CuL (15.39 m) and NN (48) of D. hamiltonii and the NN of B. balcooa (49) exhibited insignificant differences with the CuL and NN of B. nutans. Among all bamboo species, the highest CuD (5.70 cm) was recorded in D. hamiltonii, followed by B. nutans (4.70 cm). D. strictus exhibited the highest NCu/Cl (65), followed by B. balcooa (51), and the lowest value was recorded in B. bambos (35). Likewise, for the six bamboo species, Tukey’s HSD test revealed significant variations in different components of the biomass (Figures 4a-d), i.e, leaf (LB), branch (BB), culm (CuB), and total aboveground biomass (TAGB) (p<0.05). D. hamiltonii reported the highest LB (29.54 Mg.ha-1), CuB (296.12 Mg.ha-1), and TAGB (362.56 Mg.ha-1), followed by B. nutans (LB: 27.18 Mg.ha-1, CuB: 198.48 Mg.ha-1, TAGB: 277.50 Mg.ha-1). B. nutans showed the highest BB (51.84 Mg.ha-1), followed by D. hamiltonii (36.90 Mg.ha-1). However, D. asper revealed minimal BB (7.54 Mg.ha-1), CuB (38.08 Mg.ha-1), and TAGB (51.14 Mg.ha-1), as well as no significant differences with the TAGB of B. bambos(63.14 Mg.ha-1).
Figure 4: Variations in total nutrient uptake by a) leaves, b) branches, c) culms, and d) aboveground biomass for six bamboo species. Means followed by different letters are significantly different (p<0.05). The error bar signifies the mean standard deviation
Nutrient uptake
In this research, the nutrient uptake, i.e., nitrogen (N), phosphorus (P), and potassium (K) by various growth components (Figure 3) of the six bamboo species exhibited significant differences (p<0.05). Regarding the nutrient uptake by leaves, Tukey’s HSD test revealed that the highest N (0.25 Mg.ha-1), P (0.07 Mg.ha-1), and K (0.36 Mg.ha-1) uptake was recorded by D. hamiltonii, which remained statistically on par with the N (0.24 Mg.ha-1) and P (0.06 Mg.ha-1) uptake by leaves of B. nutans. Significant variations in the nutrient uptake by branches were also observed in bamboo species, with the highest N (0.42 Mg.ha-1), P (0.07 Mg.ha-1), and K (0.46 Mg.ha-1) uptake being recorded by B. nutans, followed by D. hamiltonii ( N: 0.28 Mg.ha-1, P: 0.06 Mg.ha-1, K: 0.34 Mg.ha-1), whereas the lowest N (0.06 Mg.ha-1), P (0.01 Mg.ha-1), and K (0.06 Mg.ha-1) uptake was recorded by D. asper. As for the nutrient uptake by culms, D. hamiltonii showed the highest N (1.78 Mg.ha-1), P (0.35 Mg.ha-1), and K (2.34 Mg.ha-1) uptake values, followed by the N (1.34 Mg.ha-1), P (0.25 Mg.ha-1), and K (1.57 Mg.ha-1) culm uptake of B. nuatns. The lowest N, P, and K uptake values reported by culms correspond to D. asper (0.22, 0.04, and 0.28 Mg.ha-1) which was insignificantly different from the N (0.23 Mg.ha-1), P (0.05 Mg.ha-1), and K (0. Mg.ha-1) culm uptake of B. bambos. Among the bamboo species, the overall total aboveground nutrient uptake also varied significantly (p<0.05). The highest total aboveground N, P, and K uptake (2.31, 0.47, and 3.04 Mg.ha-1) was observed in D. hamiltonii, followed by B. nutans (2.00, 0.38, and 2.36 Mg.ha-1).
Soil physicochemical properties
In the bamboo plantations, there was a significant reduction regarding soil properties (Table 1), i.e., soil porosity (POR), soil electrical conductivity (EC), soil organic carbon (SOC), available soil nitrogen (ASN), available soil phosphorus (ASP), and available soil potassium (ASK), as well as an increase in soil parameters like bulk density (BD), particle density (PD), and pH, as the soil depth increased (p<0.05).
Table 1: Soil physicochemical properties of six bamboo species in 14-year-old plantation and a control plot in the Terai region of the Central Himalayas
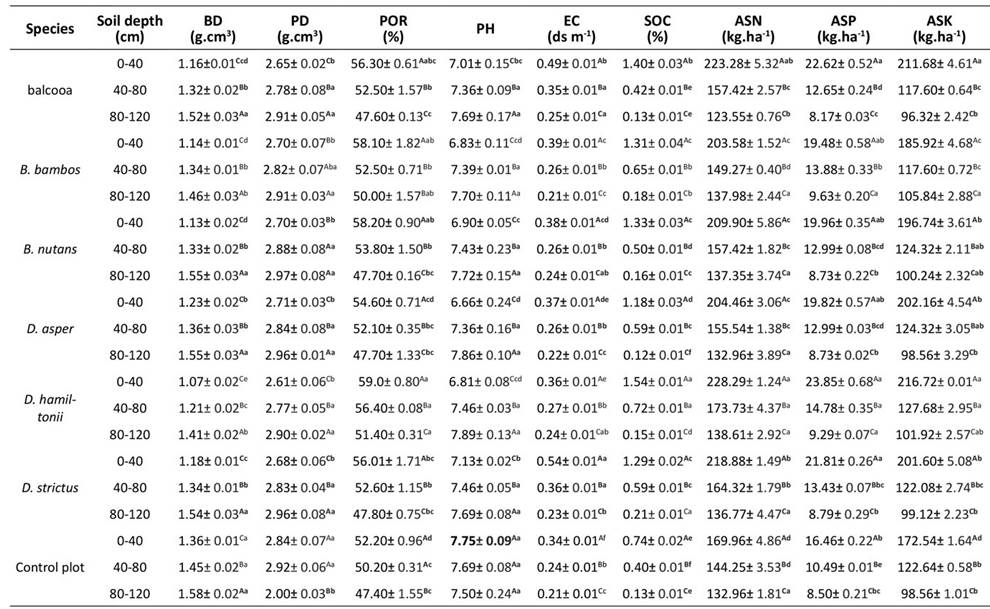
These values correspond to the mean ± standard deviation (n = 4). Different lower-case letters indicate significant differences in the soil characteristics of bamboo species and the control plot in the same soil layer. Different capital letters indicate significant differences in the soil characteristics of different soil layers for the same treatment (ANOVA and LSD test, p<0.05). BD: bulk density; PD: particle density; EC: electrical conductivity; POR: soil porosity; ASN: available soil nitrogen; ASP: available soil phosphorous; and ASK: available soil potassium
In the soil layer (0-40 cm), the highest BD (1.36 g.cm3) was observed in the control plots, followed by D. asper (1.23 g.cm3), whereas the lowest value was observed in D. hamiltonii (1.07 g.cm3). Similarly, the open plots exhibited the highest PD (2.84 g.cm3), but no significant difference was observed in the different bamboo species. The soil pH of the control plots was found to be slightly alkaline while also exhibiting the highest value (7.75), whereas the lowest soil pH was recorded in D. asper (6.66), on par with B. bambos (6.83) and D. hamiltonii (6.81). The highest soil POR was recorded in D. hamiltonii (59.00%), close to those of B. nutans (58.20 %), B. bambos (58.10 %), and B. balcooa (56.30 %). The highest soil EC was observed in D. strictus (0.54 ds m-1), followed by B. balcooa (0.49 ds m-1). The highest SOC (1.54 %) was recorded in D. hamiltonii, also followed by B. balcooa (1.40 %). The open plots had the lowest soil POR (52.20 %), EC (0.34 ds m-1), and SOC (0.74 %). Among the bamboo species, the highest ASN (228.29 kg.ha-1) and ASK (216.72 kg.ha-1) were recorded in D. hamiltonii, on par with the ASN (223.28 kg.ha-1) and ASK (211.68 kg.ha-1) of B. balcooa. In contrast, the open plots had the lowest ASN (169.96 kg.ha-1) and ASK (172.54 kg.ha-1). Regarding ASP, there was no significant variation between the bamboo species and the open plot.
Tukey’s HSD test also revealed that, in the 40-80 cm soil layer, the open plots had the highest soil BD (1.45 g.cm3), and D. hamiltonii showed the lowest value (1.21 g.cm3). As for the soil POR, D. hamiltonii exhibited the highest value (56.40 %), and the lowest was recorded in the open plots (50.20 %) and D. asper (52.10 %), which exhibited no significant difference. The highest soil EC (0.36 ds m-1) was observed in D. strictus, on par with B. balcooa (0.35 ds m-1). Among the bamboo species, D. hamiltonii exhibited the highest SOC (0.72 %), followed by B. bambos (0.65 %), the highest ASN (173.73 kg.ha-1), followed by D. strictus (164.32 kg.ha-1), and the highest ASP (14.78 kg.ha-1) in the 40-80 cm soil layer, while the open plots had the lowest SOC (0.40 %), ASN (144.25 kg.ha-1), and ASP (10.49 kg.ha-1). However, there was no significant difference in the soil PD, pH, and ASK between the bamboo species and the open plots.
In the 80-120 cm soil layer, the variations in soil BD, PD, pH, EC, ASN, ASP, and ASK were not statistically significant, except for SOC, whose highest value was recorded in D. strictus (0.21 %), followed by B. bambos (0.18%), whereas the lowest was reported by D. asper (0.12 %) (Tukey’s HSD test).
Soil microbial population
The soil microbial population exhibited significant variations between the bamboo species and the open plot (p<0.05). The highest fungi count was observed in B. nutans (101.20 ×104 cfu.g-1), which did not differ significantly from that of D. hamiltonii (100.47 ×104 cfu.g-1), followed by D. strictus (97.14 ×104 cfu.g-1) and B. bambos (95.61 ×104 cfu.g-1). The open plot had the lowest fungi count (54.40 ×104 cfu.g-1). Among the different bamboo species, the highest actinomycetes count was reported by D. hamiltonii (54.16 ×105cfu.g-1), followed by B. nutans (51.33 ×105cfu.g-1), and the lowest value was recorded in the open plot (30.45 ×105cfu.g-1). Similarly, the bacterial count was significantly higher in D. hamiltonii (68.30 ×106cfu.g-1), followed by B. nutans (65.11×106cfu.g-1), and it was minimal in open plots (36.22 ×106cfu.g-1).
DISCUSSION
Growth parameters and biomass
In this research, the variations in the growth parameters of the six different bamboo species, which grew under similar environmental conditions, may be indicative of their unique genetic makeup (Kaushal et al., 2020b), as well as due to internal factors such as the plant's origin, species type, and resilient system. All bamboo species in the plantations are sympodial and are prone to growing centrifugally, i.e., growing from the center outwards. Due to the varying adaptive potentials of these bamboo species with regard to their surroundings and environment, numerous external factors may have an impact on the growth rate (Hassan et al., 2022).
The variation in the clump girth, i.e., the measurement of the enclosing boundary of the cluster of bamboo poles, depends on the mortality and recruitment of new culms in the bamboo clumps (Franklin et al., 2010; Iyer, 2019). Chen et al. (2022) argued that internodal length is affected by the growth of fiber and parenchyma cells in the bamboo culm. Similarly, Chen (2021) suggested that this aspect is dependent on the function of intercalary meristem present at the base of the internode, which increases the internodal length by elongation of the meristematic cells. This could be the case of B. nutans in this research. Similar findings were reported by Singh et al. (2018) and Gaikwad et al. (2021), who recorded the highest clump girth and internodal length of B.nutans in bamboo plantations. Likewise, Kaushal et al. (2020b) reported the highest clump girth for B. nutans (15.40 m) and the lowest one for D. asper (5.10 m) in 12-year-old bamboo plantations, which is consistent with our findings.
The culm length growth of the bamboo species, which shows a substantial association with the aboveground biomass according to the adaptive potential regarding the given environmental conditions (Noguchi & Yoshida, 2005), corroborates the highest culm length reported by B. nutans, which was followed by D. hamiltonii. Similarly, Singh et al. (2018) recorded the significant growth (8.70 m) of B. nutans in the central plains of India, which was lower than that reported in this study. Likewise, the study by Kanwal (2011) attributed the highest culm height to B. nutans over eight other bamboo species in the central Himalayan zone of India.
Large-diameter bamboo culms are increasingly in demand as sustainable raw materials for the wood industries (Zhang et al., 2013), which depends on the growth of ground and vascular tissue in the intermediate layer of bamboo culms (Xie et al., 2014; Wang et al., 2022). This might be the reason for the higher culm diameter growth of D. hamiltonii in our study. Similar findings were published by Pattanaik and Hall (2014) and Zhan et al. (2016), who observed a greater diameter growth in D. hamiltonni in the east Khasi hills of Meghalaya, India (7.16 cm) and in the Yunnan province, China (10-18.5 cm). These values are higher than ours. Likewise, Kaushal et al. (2020b) reported a great culm diameter growth for D. hamiltonii over other bamboo species in the central Himalayan region.
In the bamboo species, the aggregation of culms per clump was dependent on the activity of the adventitious buds present on the rhizome, which ultimately develop into new culm recruits (Zhao-Hua et al., 2005). Likewise, Li et al. (2021) found that the number of culms per clump is positively correlated with the vitality and growth of the belowground rhizome, which varies from species to species and is determined by capacity of a bamboo species to adapt to a given environment, which might be the reason for the higher culm density per clump in D. strictus. Similarly, Kittur et al. (2016) recorded 75 culms per clump of D. strictus in a seven-year-old bamboo plantation in the humid climate of India, which is comparable to our findings.
The variations in the aboveground growth parameters, i.e., the leaves, branches, and culm of the bamboo species, is dependent on species’ characteristics and their ability to compete for limited resources. Similarly, different bamboo species have distinct phylogenetic, morphological, and physiological features, as well as distinct biomass allocation strategies to optimize resource uptake (Mensah et al., 2016). Oli & Kandel (2005) and Puangchit et al. (2019) recorded the branch biomass (12.24 t. ha-1; 10 t. ha-1) of B. nutans in the eastern Terai region of Nepal and in the reserve forest of Myanmar, with values lower than those of our findings. Similarly, Kaushal et al. (2022) observed the highest branch biomass in B. nutans in the foothills of western Himalaya. Likewise, in comparison with our study, Shanmughavel & Francis (2003) recorded higher values for the culm (431.66 Mg. ha-1) and the total aboveground biomass (623.05 t. ha-1) of D. hamiltonii in wastelands of Coimbatore, India.
Nutrient uptake
Various factors such as species, age, developmental phase, soil fertility, climatic factors, and other variables have a general impact on the uptake, accumulation, and distribution of nutrients throughout the various plant parts (Ovington, 1965). According to Kim et al. (2018), the variations in the nutrient uptake by different biomass components of bamboo species can be linked to their response to soil nutrition and their propensity to accumulate various amounts of dry matter in different parts. Similarly, resource allocation and their utilization by various structural and functional organs for habitat adaptation might be another feasible explanation for the observed variations in nutrient uptake (Guo et al., 2021).
Many researchers (Singh & Kochhar, 2005; Singh & Rai, 2012; Kumar et al., 2006) have documented the nutrient uptake by different biomass components of various bamboo species. Like any other plant species, the primary organ for organic matter production is the leaf, while branches and stems serve as storage organs, particularly in older bamboos (Wu et al., 2009). The differences in nutrient uptake by the leaves of the bamboo species could be ascribed to their specific photosynthesis strategy, which is influenced by genetic variability (Luo et al., 2021). Our findings are consistent with those of Gaikwad et al. (2021), who recorded the highest N uptake by leaves and the highest P and K uptake by branches in a two-year-old B. nutans plantation in semi-arid tracts of Madhya Pradesh, India. Likewise, Kumari & Bharadwaj (2017) recorded the N and P uptake of D. hamitonii leaves (0.19 Mg. ha-1-; 0.02 Mg. ha-1) in the subtropical climate of Himachal Pradesh, values lower than those of our findings.
The maximal nutrient uptake by culms is attributed to bamboo species with a high biomass production and nutrient export potential via culm harvesting (Singh & Rai, 2012), which corroborates the high nutrient uptake by the culms of D. hamiltonii in our plantation. Similar findings were published by Kumari & Bharadwaj (2019) who reported the total N (0.21 t. ha-1), P (0.03 t. ha-1), and K (0.19 t. ha-1) uptake by D. hamiltonii in ten-year-old bamboo plantations, with values lower than those of our findings. Likewise, Gaikwad et al. (2021) observed lower values of total N (0.45 t. ha-1), P (0.04 t. ha-1), and K (0.37 t. ha-1) uptake by B. nutans in comparison with our study.
Soil physicochemical properties
There are significant differences regarding the impact of different bamboo species on soil physicochemical properties and the microbial population. The soil BD, which has a substantial impact on soil fertility, water flow, and gas penetration, is impacted by a variety of factors, including the aboveground biomass, compaction, and the plant species' rooting system, among others (Nawaz et al., 2013). The reduced soil BD in the 0-40 cm soil layer of our bamboo plantations is most likely due to an increased root dispersion, which can loosen the soil (Zhou & Shangguan, 2007). The rooting systems of different bamboo species may be the reason for the differences in soil BD (Cao et al., 2011). In the 0-40 and 40-80 cm soil layers, D. hamiltonii reported the lowest soil BD, which could be attributed to higher production of fine roots and aboveground biomass, litterfall rate, and decomposition (Seobi et al., 2005; Udawatta et al., 2009). Kaushal et al. (2020a) reported higher fine root production and litterfall as well as a lower soil BD under D. hamiltonii in comparison to other bamboo species. The slight decrease in soil particle density when compared to the control plot might be due to maximal root spread in the upper soil layer, which generates channels in the soil and might have accelerated the downward movement of finer soil particles (silt and clay), thus reducing soil particle density as suggested by El-amin et al. (2001). Similarly, Selassie & Ayanna (2013) and Pandey (2019) observed a decline in soil particle density with the addition of soil organic matter in bamboo plantations.
Soil porosity is the proportion of pore space in a given volume of soil, which is determined by the soil BD, organic matter, and particle shape and size, among others (Robinson et al., 2022). The smaller pore space in the 40-80 and 80-120 cm soil layers when compared to the 0-40 cm level of the bamboo plantations could be attributed to reductions in root distribution, biomass, total root length, and the root area per unit of soil volume (Kodešová et al., 2006; Hao et al., 2019). Arunachalam & Arunachalam (2002) and Kumar et al. (2021) estimated the soil pore space under different bamboo species. Similarly to our study, Patra et al. (2022) observed maximum values for soil porosity under D. hamiltonii in the northwestern Himalayan region. The slightly more acidic nature of the 0-40 cm soil layer in comparison with the 40-80 and 80-120 cm levels may be explained by the presence of litterfall, which causes more humus to accumulate. The decomposition of this humus may have resulted in organic acids, causing a decrease in the soil pH (Kumar et al., 2022; Qian et al. 2021)
The electrical conductivity of the soil is determined by its particle size, dispersion, porosity, water retention, and temperature (Bai et al., 2013). The enhanced electrical conductivity of the 0-40 cm soil layer under the bamboo plantations might be due to a better water retention, as a result of the larger pore space (Bai et al., 2013; Fu et al., 2021). Likewise, the decomposition of litterfall, which leads to the leaching of bases under the bamboo species, could be a plausible explanation for the increased soil electrical conductivity (Kim et al., 2018; Gaikwad et al., 2021; Kumar et al., 2022). The higher soil organic carbon in the 0-40 cm soil layer under the bamboo plantations could be due to the species' rapid growth rate, resulting in the continuous addition of litterfall to the soil (Lei et al., 2019; Kaushal et al., 2020b; Benbi et al., 2015). Similarly, the dense mat-like root systems of rhizomatous bamboo species, along with their small and finer roots in the upper soil layer, may contribute to more stable soil aggregates, less soil erosion, and an increase in the organic carbon content of the soil (Qiao et al., 2016; Dong & Kou, 2022). Likewise, variations in above- and belowground biomass production, as well as the disintegration rate, could be valid explanation for the high soil organic carbon content under D. hamiltonii.
The addition of soil organic matter and nutrient return through litterfall, as well as the extensive fibrous interconnected root structure of bamboo plantations, have the potential to reduce the surface water flow velocity and increase the retention of more nutrients in the soil, which would otherwise be lost due to erosion (Shiau et al., 2017). This could be the reason for the greater availability of soil N, P, and K under the bamboo plantations in comparison to the control plot. Singh and Singh (2006) posited that bamboo species that allocate more biomass to the foliage are likely to have more nutrient-rich litterfall and enhanced soil fertility, which may be the reason for the higher soil nutrient availability under D. hamiltonii. Similarly to our study, Kanwal et al. (2011) reported the ASP (24.84 kg.ha-1) and ASK (191 kg.ha-1) values of the 0-40 cm soil layer in a six-year-old D. hamiltonii plantation in the Central Himalayas.
Soil microbial population
The greater microbial populations found under the bamboo plantations in comparison with the control plot could be attributed to litterfall, which is a carbon and nitrogen source for the soil. This would boost soil enzymatic activities and soil organic carbon, consequently increasing microorganism diversity in the soil (Tian et al., 2007; Chang et al., 2019). Researchers such as Xue et al. (2017) and Zhang et al. (2020) have suggested a positive relationship between the ASN, the SOC, and the diversity of soil microorganisms. Likewise, bamboo roots exude various metabolic products, which attract diverse soil microflora into the rhizosphere (Trivedi et al., 2020; Zheng & Lin, 2020), which might be a plausible explanation for the higher microbial population under the bamboo plantations. Similarly to our study, Kaushal et al. (2020b) revealed that D. hamiltonii had the highest actinomycetes count (8.4 ×105cfu. g-1) compared to other species in a ten-year-old bamboo plantation. On contrary, Arunachalam & Arunachalam (2002) reported the lowest bacterial (7.080 ×105cfu. g-1) and fungal (22.53 ×103 cfu. g-1) soil count under D. hamiltonii among other bamboo species in the humid tropics of India.
CONCLUSIONS
For homogeneous climate conditions, this study revealed that the aboveground growth characteristics of several bamboo species can vary, indicating their adaptive and production potential, which is an important indicator for species selection in plantations. B. nutans and D. hamiltonii, due to their larger and thicker culm walls, can act as excellent sustainable building materials, as they can support great loads. These species also accumulated the highest amount of total aboveground biomass, making them excellent raw materials for bio-energy, pulp, paper, and paperboard production, as well as a more effective terrestrial carbon sink for climate change mitigation. The assessment of nutrient uptake by bamboo species is critical for optimizing soil nutrient management in order to enchance the plantation output potential. D. hamiltonii performed best in improving the soil physicochemical properties and enhancing the soil microbial population. Thus, it is suitable for the restoration of fallow lands in the Terai region of the Central Himalayas.
Acknowledgements
ACKNOWLEDGEMENTS
The authors gratefully acknowledge Dr. D. K. Dwiedi from the G. B. Pant University of Agriculture and Technology, Pantnagar, for proofreading the article. We would also like to extend our appreciation to Dr. José Gutiérrez, the style editor of Colombia Forestal, for all of his hard work in making the manuscript accurate.
Referencias
Licencia
Derechos de autor 2024 Colombia forestal

Esta obra está bajo una licencia internacional Creative Commons Atribución-CompartirIgual 4.0.
Colombia Forestal conserva los derechos patrimoniales (copyright) de las obras publicadas, y favorece y permite la reutilización de las mismas bajo la licencia Creative Commons Atribución-CompartirIgual 4.0 Internacional por lo cual se pueden copiar, usar, difundir, transmitir y exponer públicamente, siempre que:
Se reconozcan los créditos de la obra de la manera especificada por el autor o el licenciante (pero no de una manera que sugiera que tiene su apoyo o que apoyan el uso que hace de su obra).