
DOI:
https://doi.org/10.14483/23448393.21091Publicado:
2024-11-27Número:
Vol. 29 Núm. 3 (2024): Septiembre-diciembreSección:
Inteligencia ComputacionalHand Tremor Characterization from a Spatiotemporal Convolutional Representation
Caracterización del temblor de manos a partir de una representación espaciotemporal de carácter convolucional
Palabras clave:
Tremor, Explainability maps, Volumetric convolution, Resting tremor, Postural tremor (en).Palabras clave:
Temblor, Mapas de explicabilidad, Convolución volumétrica, Temblor en reposo, Temblor postural (es).Descargas
Referencias
Z. Ou, J. Pan, S. Tang, D. Duan, D. Yu, H. Nong, and Z. Wang, "Global trends in the incidence, prevalence, and years lived with disability of parkinson's disease in 204 countries/territories from 1990 to 2019," Front. Public Health, vol. 9, p. 776847, 2021.
https://doi.org/10.3389/fpubh.2021.776847
A. Castro Toro and O. F. Buritic'a, "Parkinson's disease: Diagnostic criteria, risk factors and progression, and assessment scales clinical stage," Acta. Neurol. Colomb., vol. 30, no. 4, pp. 300-306, 2014.
J. Pasquini, G. Deuschl, A. Pecori, S. Salvadori, R. Ceravolo, and N. Pavese, "The clinical profile of tremor in parkinson's disease," Mov. Disord. Clin. Pract., vol. 10, no. 10, pp. 1496-1506, 2023. https://doi.org/10.1002/mdc3.13845
B. Kilinc, N. Cetisli-Korkmaz, L. S. Bir, A. D. Marangoz, and H. Senol, "The quality of life in individuals with parkinson's disease: Is it related to functionality and tremor severity? A cross-sectional study," Physiother. Theory. Pract., pp. 1-10, 2023.
https://doi.org/10.1080/09593985.2023.2236691
K. P. Bhatia et al., "Consensus statement on the classification of tremors from the task force on tremor of the international parkinson and movement disorder society," Mov. Disord., vol. 33, no. 1, pp. 75-87, 2018. https://doi.org/10.1002/mds.27121
L. P. S'anchez-Fern'andez, L. A. S'anchez-P'erez, P. D. Concha-G'omez, and A. Shaout, "Kinetic tremor analysis using wearable sensors and fuzzy inference systems in parkinson's disease," Biomed. Signal Process Control, vol. 84, p. 104748, 2023.
https://doi.org/10.1016/j.bspc.2023.104748
C.-H. Lin, J.-X.Wu, J.-C. Hsu, P.-Y. Chen, N.-S. Pai, and H.-Y. Lai, "Tremor class scaling for parkinson disease patients using an array x-band microwave doppler-based upper limb movement quantizer," IEEE Sens. J., vol. 21, no. 19, pp. 21 473-21 485, 2021. https://doi.org/10.1109/JSEN.2021.3103803
X. Zheng, A. Vieira, S. L. Marcos, Y. Aladro, and J. Ordieres-Mer'e, "Activity-aware essential tremor evaluation using deep learning method based on acceleration data," Parkinsonism. Relat. Disord., vol. 58, pp. 17-22, 2019. https://doi.org/10.1016/j.parkreldis.2018.08.001
S.-H. Lee, D. Lee, J. Park, J.-M. Shim, and B. Kim, "Quantification of tremor dynamics via video-based analysis," Multimed. Tools Appl., pp. 1-19, 2024. https://doi.org/10.1007/s11042-024-18438-y
M. U. Friedrich et al., "Validation and application of computer vision algorithms for video-based tremor analysis," NPJ Digit. Med., vol. 7, no. 1, p. 165, 2024. https://doi.org/10.1038/s41746-024-01153-1
H. B. Kim et al., "Wrist sensor-based tremor severity quantification in parkinson's disease using convolutional neural network," Comput. Biol. Med., vol. 95, pp. 140-146, 2018. https://doi.org/10.1016/j.compbiomed.2018.02.007
H.-Y. Wu, M. Rubinstein, E. Shih, J. Guttag, F. Durand, and W. T. Freeman, "Eulerian video magnification for revealing subtle changes in the world," ACM Trans. Graph. (Proc. SIGGRAPH 2012), vol. 31, no. 4, 2012. https://doi.org/10.1145/2185520.2335416
A. Gironell, B. Pascual-Sedano, I. Aracil, J. Mar'ın-Lahoz, J. Pagonabarraga, and J. Kulisevsky, "Tremor types in parkinson disease: a descriptive study using a new classification," Parkinson's Dis., vol. 2018, no. 1, p. 4327597, 2018. https://doi.org/10.1155/2018/4327597
E. D. Louis, "Tremor," Continuum (Minneap. Minn.), vol. 25, no. 4, pp. 959-975, 2019. https://doi.org/10.1212/CON.0000000000000748
H. Zach, "Parkinson's tremor: Effects of dopamine and cognitive load," Ph.D. dissertation, Radboud Univ., 2023.
H. Zach, M. Dirkx, B. R. Bloem, and R. C. Helmich, "The clinical evaluation of parkinson's tremor," J. Parkinson's Dis., vol. 5, no. 3, pp. 471-474, 2015. https://doi.org/10.3233/JPD-150650
Cómo citar
APA
ACM
ACS
ABNT
Chicago
Harvard
IEEE
MLA
Turabian
Vancouver
Descargar cita
Recibido: 31 de julio de 2023; Aceptado: 5 de agosto de 2024
Abstract
Context:
Parkinson’s Disease (PD) is a neurodegenerative disorder related to dopamine deficiency that mainly entails motor conditions such as slowness of movement, postural instability, limb tremor, rigidity, and a decreased range of motion. Tremor, defined as a rhythmic and uncontrolled movement of limbs, is the most prevalent symptom in PD. In the clinical routine, tremors are assessed and quantified by observing the hands following postural and resting patterns. These configurations include voluntary muscular contractions and tremor perception reduction, which leads to noisy signals. The assessments are also subjective and depend on the expertise of professionals to determine whether the tremor is associated with PD.
Method:
This work introduces a deep volumetric representation that characterizes PD tremor patterns in resting and postural recording conditions. The strategy includes a convolutional architecture that extracts spatiotemporal patterns correlated with tremor, propagated through different layers until dis- crimination between PD and control subjects is achieved. Moreover, a set of explainability maps is computed by backpropagating output gradients into convolutionally learned spatio-temporal maps.
Results:
The method was evaluated on 80 videos (five PD patients and five control subjects), reporting an average accuracy of 92.5 % and a perfect sensitivity score in the postural configuration. As for the resting scheme, the proposed method obtained an average accuracy of 90 % and sensitivity of 80 %.
Conclusions:
This approach showed efficacy regarding the localization of tremor patterns, recovering movement information while preserving the spatial and temporal representation. The strategy allows visualizing movement patterns from explainability maps of control subjects and PD patients.
Keywords:
tremor, explainability maps, volumetric convolution, resting tremor, postural tremor.Resumen
Contexto:
La enfermedad de Parkinson (EP) es un trastorno neurodegenerativo relacionado con la deficiencia de dopamina que conlleva principalmente afecciones motoras como lentitud de movimientos, inestabilidad postural, temblor de las extremidades, rigidez y disminución del rango de movimiento. El temblor, definido como un movimiento rítmico e incontrolado de las extremidades, es el síntoma más prevalente de la EP. En la rutina clínica, los temblores se evalúan y cuantifican observando las manos siguiendo patrones posturales y de reposo. Estas configuraciones incluyen contracciones musculares voluntarias y reducción de la percepción del temblor, lo que conduce a señales ruidosas. Las evaluaciones también son subjetivas y dependen de la experiencia de los profesionales para determinar si el temblor está asociado a la EP.
Método:
Este trabajo introduce una representación volumétrica profunda que caracteriza los patrones de temblor en la EP en condiciones de registro en reposo y posturales. La estrategia incluye una arquitectura convolucional que extrae patrones espaciotemporales correlacionados con el temblor, los cuales se propagan a través de diferentes capas hasta lograr la discriminación entre sujetos con EP y sujetos control. Además, se calcula un conjunto de mapas de explicabilidad retropropagando los gradientes de salida hacia los mapas espaciotemporales aprendidos de forma convolucional.
Resultados:
El método fue evaluado en 80 videos (cinco pacientes con EP y cinco sujetos control), reportando una precisión promedio del 92.5 % y una puntuación de sensibilidad perfecta en la configuración postural. En cuanto al esquema en reposo, el método propuesto obtuvo una precisión promedio del 90 % y una sensibilidad del 80 %.
Conclusiones:
Este enfoque mostró eficacia en la localización de los patrones de temblor, recuperando información de movimiento mientras preservaba la representación espacial y temporal. La estrategia permite visualizar los patrones de movimiento a partir de mapas de explicabilidad tanto de sujetos control como de pacientes con EP.
Palabras clave:
temblores, mapas de explicabilidad, convolución volumétrica, temblor en reposo, temblor postural.Introduction
Parkinson’s disease (PD) is the second most prevalent neurodegenerative disorder, marked by a deficit in dopamine and impacting about 1 % of individuals aged 60 and older 1. In Colombia, PD has an estimated prevalence of 4.7 per 1000 inhabitants, being more frequent in people over 60 years old 2. Physiologically, PD is associated with a progressive loss of dopamine, a neurotransmitter responsible for optimal and synchronized locomotion processes. Due to this dopamine deficit, motor alterations affect coordinated movements and balance. For instance, postural instability, muscle rigidity, slowness of movement, tremors, and voice involvement constitute the typical symptoms of PD. Tremor is a dominant symptom of this disease. It is a rhythmic and involuntary movement caused by a muscle’s reciprocal innervations, which vary in intensity and frequency 3. In approximately 50 % of patients, the cardinal motor is tremor, and only 10 % do not have this symptom. Tremor patterns also vary in frequency and amplitude. Consequently, they can be identified depending on the posture of the limbs and the presence or absence of force. Hence, the detection and characterization of tremors are key to establishing complementary mechanisms to the standardized protocols that allow diagnosing PD.
In clinical practice, resting and postural tremors are commonly evaluated, primarily focusing on amplitude and persistence 4. In the resting configuration, the arms are supported by the muscles or a stable surface, and the tremor is observed during relaxation. Alternatively, the arms are held against gravity at a 90° angle from the body in the postural configuration. In this sense, the physical effort caused by maintaining the arms in this unnatural posture guarantees motion exaggeration. The unnatural position of the arms causes this overload; therefore, these schemes include noisy signals limiting the adequate quantification of hand tremor patterns. Every kind of tremor shows a different range of motion frequency, with resting tremors usually between 4 and 6 Hertz. Meanwhile, postural tremors correspond to the broader range, i.e., between 5 and 12 Hertz 5. Additionally, the movement frequency can be augmented for stress or anxiety and can reduce involuntary movements.
The quantification of tremors is usually performed using technological tools that integrate electronic devices and portable systems (inertial-type sensors), such as accelerometers, gyroscopes, and magnetometers 6. For instance, systems based on inertial sensors placed on the hands and arms quantify velocity and acceleration rates in postural and tremor configurations. Similarly, inertial sensors based on kinematic measures can separate Parkinsonian patterns from control subjects. Complex systems based on the Doppler effect can measure upper limb movements
showing frequencies, tremors, and the direction of signal tremors in the range of 0-8 Hz 7. In addition, 8 quantified the acceleration data into a deep learning scheme to quantify the tremor severity. However, these systems require fine calibration to reduce the signal noise, but, in some cases, motion pens reduce the movement amplitude and can be challenging to detect slight motions.
Some works have exploited video techniques to quantify tremor features through non-invasive strategies. Specifically, video has been used indirectly to measure tremors. In this kind of method, the participant holds the camera with their hands in a postural position and places it in front of a common background, which helps to characterize kinematic trajectories 9. However, this method has several drawbacks: it is highly dependent on maintaining a static position of the arm extended at 90 degrees relative to the trunk, which is challenging for healthy participants, and even more so for patients. These additional movements not associated with tremors make it difficult to distinguish between patients and control subjects.
Another example of video techniques involves recording the hands and identifying landmarks, which allows quantifying kinematic characteristics related to amplitude and frequency 10. However, this approach is quite sensitive to noise, as the location of points of interest between frames can vary throughout the video without being associated with tremors. These slight errors add noise to subtle tremors. Additionally, the number of landmarks may lead to a simplification of tremor; a reduced number of landmarks can affect the identification of tremor-associated patterns.
Interestingly, 11 proposed a convolutional neural network (CNN) scheme to codify the tremor severity through a wearable wrist device equipped with an accelerometer and a gyroscope. Here, the collected signals are transformed into the frequency domain and then mapped to the deep net. Furthermore, 8 demonstrated the feasibility of using acceleration data in three directions to quantify tremor severity. This approach measured the arm movements of 20 people performing daily actions like drinking, extending their arms, touching their noses, stacking glasses, drawing, and writing. All the captured signals were then filtered in order to identify voluntary human movements and the severity of tremor patterns through activity classification models (ACM) and tremor assessment models (TEM). Despite these advantages, all these strategies still rely on physical devices that might introduce difficulties associated with sophisticated calibration processes and external noise sources that may bias the recorded measures. Moreover, sensibility is also compromised due to the anti-natural movement caused by the invasive devices.
This work introduces a convolutional spatiotemporal representation able to encode hand tremor patterns associated with PD, which allows distinguishing between Parkinsonian and control patients. This distinction was made separately for both resting and postural configurations. To this effect, hand video sequences were magnified to highlight associated tremors. The hand tremor patterns were then encoded via a hierarchical architecture involving 3D convolutions and embedded dense vectors with motion information. The results showcase the capabilities of this architecture, which provides indices correlated with PD. In addition, explainability maps were computed to support clinical decisions, enabling the visualization of the regions that, according to the network, are associated with a specific prediction.
Proposed approach
This work quantifies the spatiotemporal information through a 3D convolution strategy to classify Parkinsonian hand tremors in video sequences. Furthermore, as previously mentioned, explainability maps were computed as a visual tool to support clinical decisions within the deep representation strategy. Fig. 1 illustrates the proposed methodology.
Figure 1: Representation of the proposed methodology. The upper part shows the 3D convolutional architecture, where each row represents different inputs in the network, and the bottom part presents the feature maps of the model
Deep convolutional 3D architecture
The proposed architecture considers spatial and temporal convolutions to identify spatiotemporal features from video sequences and capture tremor patterns in postural and resting configurations. Specifically, for each layer L, linear transformations are progressively computed, followed by contractive nonlinearities that project the information onto a set of q learned filters expressed as
In this model, I(x)
t
represents a video sequence projected to the first layer by Ψ1. Then, a representation
is obtained, with Ψ
g
denoting independently learned convolutional filters. The resulting representation is successively convolved with the filters of the next N − 1 layers,
The convolutional representation
is given by the representations of each layer:
. The responses of the first layers yield volumetric low-level features, such as edges and textures. These low-level features build more complex representations in the last convolutional layers. The input to this convolutional network corresponds to video sequences of hand tremors that are densely correlated in successive layers, allowing for the robust modeling of motion patterns. Fig. 1 illustrates the proposed convolutional scheme.
Explanability maps
Predictive capabilities in clinical settings face challenges associated with the poor interpretability of output predictions. To overcome such limitation, this work implemented a spatio-temporal gradient-weighted class activation mapping (Grad-CAM) approximation as an alternative visualization tool to support PD predictions (Fig. 2). Particularly, Grad-CAM maps were adapted to the volumetric responses of the different 3D convolutional layers in the proposed architecture. This strategy generates a heat map, highlighting the critical regions in a specific network input by means of the gradient information that flows from a certain output probability to a specific convolutional layer in the proposed CNN. To obtain the discriminant location map regarding the class c and an input video, the gradient of the probability score of that class (y
c
) is first computed with respect to the A
k
feature maps of a convolutional layer. These backward-flowing gradients are spatially averaged to obtain the weights
Where
is the global average pooling, and
denotes the gradients via backpropagation. After calculating the weights for the target class c, a weighted combination of activation maps is followed by a rectified linear unit (ReLU). The ReLU is applied to the linear combination since only the features that positively influence the class are of interest, and it highlights the associated regions as follows:
where
is the linear combination. Finally, an upsampling guarantees the same resolution as the original image.
Figure 2: Sum-pooling strategy for feature maps in a convolutional layer: a) input image, b) feature maps of a given convolutional layer, c) sum-pooling
Experimental setup
Data
This study included a total of ten participants: five control subjects (average age: 72,2 ± 8 years) and five patients with PD (average age: 72,3 ± 9 years). The patients had been diagnosed at the second stage of the disease by a physician who used the standard protocols of the Hoehn-Yahr scale. The Ethics Committee of Universidad Industrial de Santander approved this study, and a written informed consent was obtained from all the participants. Data recording was made possible thanks to Fundación del Adulto Mayor y Parkinson en Santander (FAMPAS) and the Biomedical Imaging, Vision, and Learning Laboratory (BIVL2ab research group). The protocols followed for the tremor configuration are detailed below.
A camera was placed on a tripod at a 45° angle, and a green background was used to highlight the hands. Additionally, a semi-controlled environment was used to avoid external brightness artifacts. The participant was seated in a comfortable position to prevent any additional movements that were not associated with tremors. The test configurations are detailed below.
In the resting configuration, the hands were supported by a table with a green background, and the palms were relaxed in an upward position. During the recording, some questions were asked to the participants, diverting their focus from tensing their hands to prevent the tremor.
In the postural configuration, the hands were held above the surface without touching it, with the palms facing downward.
Parameter tuning
The proposed approach was adjusted at different stages in order to optimize the representation with regard to the description and quantification of PD patterns. According to each stage, the following parameters were set:
Temporal Sequences: Different temporal sequence lengths (F) were considered, where F = {8,12,16,24} frames per video. Considering that each video lasts between 12 and 15 seconds, the number of frames taken represents different frequencies associated with the motion.
Spatio-temporal network: The spatio-temporal contribution was considered with three and five 3D convolutional layers. Additionally, the performance of one or two dense layers was considered. The fully connected layers were ReLU-activated. A dropout rate of 0.5 was established, which is an effective mechanism to avoid over-fitting.
Training configuration: The model was trained using the Adam optimizer, with an initial learning rate of 0.001. A scheduler was employed to reduce the learning rate by a factor of 0.1 if the validation losses did not improve for five consecutive epochs. The loss function used was the categorical cross-entropy, with L2 regularization to prevent over-fitting. The training lasted 20 epochs, with early stopping based on validation loss, applying a patience of eight epochs. A batch size with the same number of frames per video was selected (i.e., 8,12,16,24).
Experimental configuration
To evaluate the performance of the proposed approach, leave-one-patient-out cross-validation was carried out with the hand tremor dataset. At each iteration, one patient was left out for testing, and the remaining ones (nine subjects in this case) were used for training. For these experiments, the correctly classified Parkinsonian patients were counted as true positives (TP), and the control patients were identified as true negatives (TN). Then, the following set of metrics was used to fully understand the performance of the approach in its different configurations: sensitivity
, accuracy
, precision
, and the F1 score
Evaluation and results
The proposed approach aims to achieve proper differentiation between control and PD patient groups regarding hidden tremor patterns in standard video sequences recorded in postural and resting hand configurations. For validation, the videos were projected in two different versions: raw and magnified sequences. The latter were obtained after applying a temporal filter that highlights key frequencies related to PD. Particularly, classical Euler magnification 12 was employed as a pre-processing stage.
A first validation was carried out with the aim of selecting the best temporal sequence length to discriminate between PD and control patients. Fig. 3 illustrates the model’s performance in terms of accuracy and the F1 score. To perform an ablation study, the video clips were downsampled to F = (8,12,16,24) frames per video. As expected, a low frame representation level led to poor spatiotemporal video patterns. On the other hand, through a medium frame representation 12, the model achieved the best results, given its compact tremor feature characterization. It is important to note that these videos were recorded within a range of 12-15 seconds and at a typical PD tremor frequency 13. Consequently, the proposed approach shows potential in distinguishing between control subjects and patients with mild tremors in the early stages of the disease. The differentiation capabilities of full video frames were limited due to redundancy between frames.
Figure 3: Frame variation for the best architecture configuration. The x-axis shows the frame variation, while the y-axis shows the accuracy values and the F1 score (blue and red lines, respectively)
Secondly, the spatiotemporal network’s contribution was analyzed while considering three and five 3D convolutional layers, varying the number of dense layers and their corresponding number of units. Fig. 4 illustrates the network’s performance concerning accuracy and the F1 score in standard and magnified video sequences for both configurations. As expected, the best model performance was achieved through the deepest configuration (five convolutional layers and two dense layers with 2048 units). This behavior reinforces the hypothesis that hidden hand tremors could be properly coded along the temporal dimension. Nevertheless, it is important to note that a deeper configuration alone is not enough: the 5C+Dense net configuration always reports the worst performance and is even surpassed by the standard 3C setup. In this vein, an additional dense layer offers a suitable spatiotemporal representation.
Figure 4: Model performance with respect to variations in the number of convolutional and dense layers. From left to right, model performance for the resting and postural configurations. From top to bottom, model performance concerning standard and magnified video sequences
Additionally, Table I shows a detailed metric evaluation of the best model configuration for each type of video sequence. As expected, the magnified postural configuration obtained the highest F1, sensitivity, and accuracy scores. In such cases, a major effort of the limbs increases the physical effort required to keep the arms in a fixed position, which contributes to a natural exaggeration of the tremor, which may even reveal patterns that cannot be observed in the resting configuration. Particularly, tremors are more constant in the postural configuration, given the muscular effort required to maintain a posture against gravity. Thus, amplifying these standard movements significantly improves the model’s performance.
Table I: Model performance across different video configurations
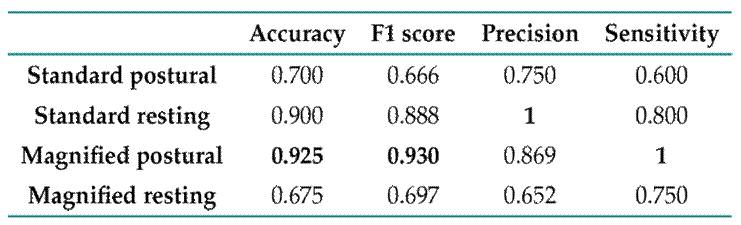
Interestingly enough, a perfect precision score was obtained under the resting hand configuration without magnification. This may be associated with the digital noise introduced by the synthetic exaggeration caused by optical magnification, i.e., control movements not associated with Parkinsonian patterns may be exaggerated, resulting in reduced precision and accuracy. Euler magnification is subject to a global constraint that may increase the dynamic noise of video sequences, even surpassing patients’ natural tremor. From a clinical perspective, it is important to use video materials that provide a clearer and less ambiguous signal. In this vein, standard resting videos are less prone to model misinterpretation than magnified ones. In light of the above, the proposed scheme yields promising results in distinguishing PD patients from control subjects, minimizing the false positive rate. It could be regarded as a potential clinical tool to support medical decisions while only requiring standard video sequences. Specifically, it could potentially discriminate between two types of tremors observed in clinical practice, i.e., postural and resting tremors. In this context, a differentiated diagnosis of tremors could support the development of more personalized treatments, thereby improving the patient’s quality of life 14.
Complementarily, Fig. 5 shows the training adjustment performed for the postural magnified configuration architecture. It can be observed that accuracy increases while losses decrease consistently for all folds until a stable configuration is reached. Also note that, in the first epochs, there are remarkable variations in the results and the stabilization of losses. Nonetheless, as the number of epochs increases, these variations are significantly reduced, showing a greater confidence in the results.
Figure 5: Training for the magnified postural architectures through k-fold cross-validation. This plot shows the mean accuracy and losses values achieved during each epoch, together with their variance (vertical lines).
As a second phase in the validation and evaluation of the proposed methodology, explainability maps were calculated to interpret and support the predictions. Notably, Grad-CAM offers weighted
volumetric visualization across different video regions, with a strong influence on the decision. Fig. 6 shows the Grad-CAM algorithm employed for the same magnified sequences of control and PD patients. Our proposal suggests the palms of the hands as the main regions to achieve control predictions. Even more interesting is the fact that, for the PD patients, the model seems to focus on the boundary regions of the hands, which are prone to slight movements. These regions are associated with a greater perception of tremors (mainly the fingertips). It can thus be assumed that convolutional bank filters computed from 3D convolutional kernels pay attention to the subtle motion of the hand and the arm. In fact, the prediction weighted with such activation shows an association with tremors. In clinical practice, there is a phenomenon called pill-rolling, wherein the patient’s tremor is predominantly observed in the thumb and forefinger 15. Notably, with an early diagnosis of this condition, the symptoms of PD can be alleviated through medication and physical therapy, helping the patient to regain independence. In this vein, the proposed approach exhibits a robust behavior in codifying hidden hand tremors in regions that are most sensitive to the patient’s movement in both resting and postural configurations.
Figure 6: Heat maps obtained using the Grad-CAM algorithm. From left to right, PD and control subjects. Columns a, b, and c show the input and the response maps of the third and fourth convolutional layers, respectively
Conclusions and future work
Nowadays, PD diagnosis strongly depends on tremor observations based on medical expertise. These observations only consider strong changes in motion, which hinders the monitoring of PD progression given the high variance in the amplitude and frequency associated with the motion of a particular patient 16. To overcome this issue, this work presented a deep volumetric strategy that represents postural and tremor configurations through video sequences, with the purpose of identifying tremors associated with PD. The strategy included a convolutional architecture that extracts spatiotemporal patterns correlated with tremors and propagates them through different layers until PD and control subjects are distinguished from each other. In addition, explainability maps were computed by backpropagating output gradients into convolutionally learned spatiotemporal maps.
This approach was tested with five patients and five control subjects, for a total of 80 video sequences, showing promising results for predicting PD from video. The two studied configurations were seen to be complementary, demonstrating the importance of using both in clinical practice. Magnified postural patterns can contribute to a greater classification sensitivity, and standard resting videos can increase precision. However, some prediction errors were observed, which may be associated with the high variability in the intensity and frequency of the patients’ tremors. Particularly, in patients with early signs of the disease, the tremors were less pronounced and less consistent throughout the
video, especially in resting configurations. In such cases, processing the video samples and adequately classifying the patients concerning the control population become challenging tasks. Despite these limitations, postural effort was seen as appropriate for indicating abnormal patterns associated with PD. Moreover, processing raw videos of the resting configuration tests was more effective than using optical Eulerian magnification. The proposed 3D convolutional network is able to capture localized tremors without any optical exaggeration, providing an accurate and useful representation for the classification model. This is in line with the preference for standard video configurations in the context of medical diagnostics, where precision and reliability are critical.
The results of the proposed approach are very descriptive, highlighting its potential to support observational analysis by enabling the identification of spatiotemporal regions with stronger associations to tremors. Therefore, the explainability maps of the control subjects show a focus on the palm as opposed to those of the patients, which reveal a focus, for instance, on the thumb and the fingertips. The results suggest a potential link between the pill-rolling PD tremor (a tremor of the thumb and the index finger) and the explainability maps. Our approach was validated by studying a reduced number of subjects, given the difficulties in acquiring data from more patients. The main issue concerning data is the ability to quantify populations with comparable demographic characteristics in order to develop approaches that allow representing and classifying disease patterns.
In light of the above, we propose further validation with larger datasets and patients stratified according to the stages of the disease. This may be useful to identify new motion patterns that contribute to the precision of clinical predictions in relation to PD progression or the effectiveness of a particular treatment. Furthermore, the inclusion of new cardinal symptoms might improve the representation and the tool’s impact on diagnostic support
Acknowledgements
Acknowledgements
This work was supported by Vicerrectoría de Investigación y Extensión (VIE) of Universidad Industrial de Santander through the project titled Quantification of prostate lesions by comparing multiand biparametric MRI sequences via artificial intelligence tools, with code 3946.
References
Licencia
Derechos de autor 2024 Jessica Pedraza Cadena, John Edinson Archila Valderrama, Franklin Sierra-Jerez, Alejandra Moreno Tarazona, Fabio Martínez Carrillo

Esta obra está bajo una licencia internacional Creative Commons Atribución-NoComercial-CompartirIgual 4.0.
A partir de la edición del V23N3 del año 2018 hacia adelante, se cambia la Licencia Creative Commons “Atribución—No Comercial – Sin Obra Derivada” a la siguiente:
Atribución - No Comercial – Compartir igual: esta licencia permite a otros distribuir, remezclar, retocar, y crear a partir de tu obra de modo no comercial, siempre y cuando te den crédito y licencien sus nuevas creaciones bajo las mismas condiciones.