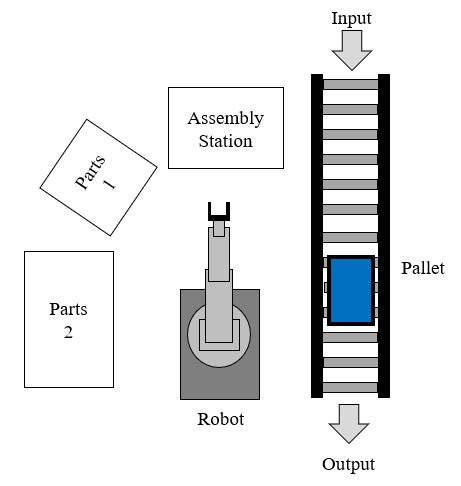
DOI:
https://doi.org/10.14483/23448393.20895Published:
2024-08-04Issue:
Vol. 29 No. 2 (2024): May-AugustSection:
Education in EngineeringScheduling in a Simple Assembly Robotics Cell to Minimize Earliness and Tardiness
Programación de trabajos en una celda robótica de ensamble simple para minimizar los adelantos y las tardanzas
Keywords:
robotic assembly cell, tardiness, scheduling (en).Keywords:
celdas de ensamble robóticas, tardanza, programación (es).Downloads
Abstract (en)
Background: Robotics Assembly Cells (RAC) have been designed to meet the flexibility requirements demanded by today's globalized market. The objective is to manufacture a vast variety of products at a low cost, which requires equipment with a high level of flexibility, such as robots. The need to schedule a great variety of jobs in an RAC is a very relevant issue, as efficiency and productivity depend on the sequence in which jobs are scheduled. Studies around this matter have developed models with analytical and heuristic approaches, as well as simulation methods and genetic algorithms, seeking to improve performance measures based mainly on time, utilization, and costs.
Method: The purpose of this article is to formulate an exact mathematical model using mixed-integer linear programming (MILP) to optimize small scheduling problems. The objective is to minimize the measure of performance related to the tardiness and earliness of jobs. This optimization aims to mitigate the effects of delays in product deliveries, queue times, and work-in-process inventory in subsequent processes. Doing so facilitates adherence to agreed-upon delivery deadlines and prevents bottlenecks in the assembly cell.
Results: The proposed mathematical model generates optimal solutions to the job scheduling problem in the assembly cell, which serves as a case study. This addresses the need to minimize tardiness to meet delivery deadlines or minimize earliness while avoiding an increase in work-in-process inventories. The model ensures that optimal scheduling decisions are made to optimize both delivery performance and inventory levels.
Conclusions: Due to the NP-hard complexity of the scheduling problem under study, the proposed mathematical model demonstrates computational efficiency in solving scheduling problems with fewer than 20 jobs. The model is designed to handle such smaller-scale problems within a reasonable computational time frame, considering the inherent complexity of the scheduling problem.
Abstract (es)
Contexto: Las celdas robóticas de ensamble RAC han sido diseñadas para cumplir con los requerimientos de flexibilidad que exige el mercado globalizado actual. El objetivo es fabricar una alta variedad de productos a bajos costos, por lo cual se requiere de equipos con un alto nivel de flexibilidad como los robots. La necesidad de programar una amplia variedad de trabajos en una RAC es un problema muy relevante, dado que la eficiencia y la productividad dependen de la secuencia en la cual se programan los trabajos. Los estudios alrededor de este asunto han desarrollado modelos con enfoques analíticos y heurísticos, así como métodos de simulación y algoritmos genéticos, que buscan mejorar medidas de desempeño basadas principalmente en el tiempo, el grado de utilización y el costo.
Método: El propósito de este artículo es formular un modelo matemático exacto mediante programación lineal entera mixta (MILP) para optimizar problemas pequeños de programación. El objetivo es minimizar las medidas de desempeño de tardanza y adelanto de los trabajos. Esta optimización busca mitigar los efectos de las demoras en las entregas de los productos, los tiempos de cola y los inventarios de producto en proceso en instancias posteriores. Esto permite cumplir con los plazos de entrega pactados y evita bloqueos en la celda de ensamble.
Resultados: El modelo matemático propuesto genera soluciones óptimas al problema de programación de trabajos en la celda de ensamble propuesta, lo que sirve como caso de estudio. Esto aborda la necesidad de minimizar las tardanzas para cumplir con los plazos de entrega o de minimizar los adelantos y evitar un aumento en los inventarios de producto en proceso. El modelo garantiza que se tomen decisiones óptimas de programación tanto para mejorar los tiempos de entrega como los niveles de inventario.
Conclusiones: Debido a la complejidad NP-difícil del problema de programación estudiado, el modelo matemático propuesto demuestra eficiencia computacional en la resolución de problemas de programación con menos de 20 trabajos. El modelo está diseñado para manejar tales problemas de menor escala dentro de un marco de tiempo computacional razonable, considerando la complejidad inherente del problema de programación.
References
M. P. Groover, "Flexible manufacturing systems," in Automation, Production Systems, and Computer-Integrated Manufacturing, Pallavaram, Chennai, India: Pearson Education India, 2016, vol. 4, ch. 16, pp. 460-462.
J. Browne, "Classification of flexible manufacturing systems," The FMS Mag., vol. 2, no. 2, pp. 114-117, 1984. [Online]. Available: https://www.academia.edu/502998/Classification_of_flexible_manufacturing_systems?auto=citations&from=cover_page
H. Gultekin, "Scheduling in flexible robotics manufacturing cells," PhD thesis, Dept. Ind. Eng., Bilkent Universitesi, 2006.
K. K. Abd, "Development of an intelligent methodology for scheduling RFAC," in Intelligent Scheduling of Robotic Flexible Assembly Cells, Berlin, Germany: Springer, 2016, pp. 31-47. https://doi.org/10.1007/978-3-319-26296-3_3
T. Sawik, "Simultaneous loading, routing, and assembly plan selection in a flexible assembly system," Mathematical Comp. Model., vol. 28, no. 9, pp. 19-29, 1998. https://doi.org/10.1016/S0895-7177(98)00142-3
G. Levitin, J. Rubinovitz, and B. Shnits, "A genetic algorithm for robotic assembly line balancing," Eur. J. Operational Res., vol. 168, no. 3, pp. 811-825, 2006. https://doi.org/10.1016/j.ejor.2004.07.030.
K. Abd, K. Abhary, and R. Marian, "Intelligent modeling of scheduling robotic flexible assembly cells using fuzzy logic," 2012. [Online]. Available: https://www.researchgate.net/publication/262398812_
M. Pinedo, "Advanced single machine models," in Scheduling; Theory, Algorithms, and Systems, 4th ed., Berlin, Germany: Springer, 2012, ch. 3, pp. 70-77.
K. K. Abd, Intelligent scheduling of robotic flexible assembly cells. Berlin, Germany, Springer, 2015.
A. Jain and H. Elmaraghy, "Production scheduling/rescheduling in flexible manufacturing," Int. J. Prod. Res., vol. 35, no. 1, pp. 281-309, 1997. https://doi.org/10.1080/002075497196082
J. Blazewicz, "Scheduling computer and manufacturing processes," J. Operational Res. Soc., vol. 48, no. 6, art. 659, 1997. https://doi.org/10.1057/palgrave.jors.2600793
R. Ramasesh, "Dynamic job shop scheduling: A survey of simulation research," Omega, vol. 18, no. 1, pp. 43-57, 1990. https://doi.org/10.1016/0305-0483(90)90017-4
C. Koulamas, "The single-machine total tardiness scheduling problem: Review and extensions," Eur. J. Operational Res., vol. 202, no. 1, pp. 1-7, 2010. https://doi.org/10.1016/j.ejor.2009.04.007
J. E. C. Arroyo, R. dos Santos Ottoni, and A. de Paiva Oliveira, "Multi-objective variable neighborhood search algorithms for a single machine scheduling problem with distinct due windows," Electron. Notes Theor. Comput. Sci., vol. 281, pp. 5-19, 2011.
S. French, Sequencing and scheduling. An Introduction to the Mathematics of the Job-Shop. Hemel Hempstead, UK: Ellis Horwood, 1982.
R. L. Graham, E. L. Lawler, J. K. Lenstra, and A. R. Kan, "Optimization and approximation in deterministic sequencing and scheduling: A survey," Ann. Discrete Math., vol. 5, pp. 287-326, 1979. https://doi.org/10.1016/S0167-5060(08)70356-X
H. Emmons, "One-machine sequencing to minimize certain functions of job tardiness," Oper. Res., vol. 17, no. 4, pp. 701-715, 1969. https://doi.org/10.1287/opre.17.4.701
E. L. Lawler, "A “pseudopolynomial” algorithm for sequencing jobs to minimize total tardiness," Ann. Disc. Math., vol. 1, pp. 331-342, 1977. https://doi.org/10.1016/S0167-5060(08)70742-8
S. K. Gupta and J. Kyparisis, "Single machine scheduling research," Omega, vol. 15, no. 3, pp. 207-227, 1987. https://doi.org/10.1016/0305-0483(87)90071-5
C. N. Potts and L. N. Van Wassenhove, "A decomposition algorithm for the single machine total tardiness problem," Oper. Res. Lett., vol. 1, no. 5, pp. 177-181, 1982. https://doi.org/10.1016/0167-6377(82)90035-9
C.-Y. Lee and J. Y. Choi, "A genetic algorithm for job sequencing problems with distinct due dates and general early-tardy penalty weights," Comp. Oper. Res., vol. 22, no. 8, pp. 857-869, 1995. https://doi.org/10.1016/0305-0548(94)00073-H
B. Yuce et al., "Hybrid genetic bees algorithm applied to single machine scheduling with earliness and tardiness penalties," Comp. Ind. Eng., vol. 113, pp. 842-858, 2017. https://doi.org/10.1016/j.cie.2017.07.018
J. Rocholl and L. Mönch, "Hybrid algorithms for the earliness–tardiness single-machine multiple orders per job scheduling problem with a common due date," RAIRO-Oper. Res., vol. 52, no. 4-5, pp. 1329-1350, 2018. https://doi.org/10.1051/ro/2018029
H. Kellerer, K. Rustogi, and V. A. Strusevich, "A fast FPTAS for single machine scheduling problem of minimizing total weighted earliness and tardiness about a large common due date," Omega, vol. 90, art. 101992, 2020. https://doi.org/10.1016/j.omega.2018.11.001
O. A. Arık, "Single machine earliness/tardiness scheduling problem with grey processing times and the grey common due date," Grey Syst. Theory Appl., vol. 11, no. 1, pp. 95-109, 2021. https://doi.org/10.1108/GS-01-2020-0010
W. Wang, "Single-machine due-date assignment scheduling with generalized earliness-tardiness penalties including proportional setup times," J. Appl. Math. Comput., vol. 68, no. 2, pp. 1013-1031, 2022. https://doi.org/10.1007/s12190-021-01555-4
How to Cite
APA
ACM
ACS
ABNT
Chicago
Harvard
IEEE
MLA
Turabian
Vancouver
Download Citation
License
Copyright (c) 2024 John Andres Muñoz Guevara, Jairo Alberto Villegas-Florez, Jhannier Jhoan Jaramillo Tabima

This work is licensed under a Creative Commons Attribution-NonCommercial-ShareAlike 4.0 International License.
From the edition of the V23N3 of year 2018 forward, the Creative Commons License "Attribution-Non-Commercial - No Derivative Works " is changed to the following:
Attribution - Non-Commercial - Share the same: this license allows others to distribute, remix, retouch, and create from your work in a non-commercial way, as long as they give you credit and license their new creations under the same conditions.