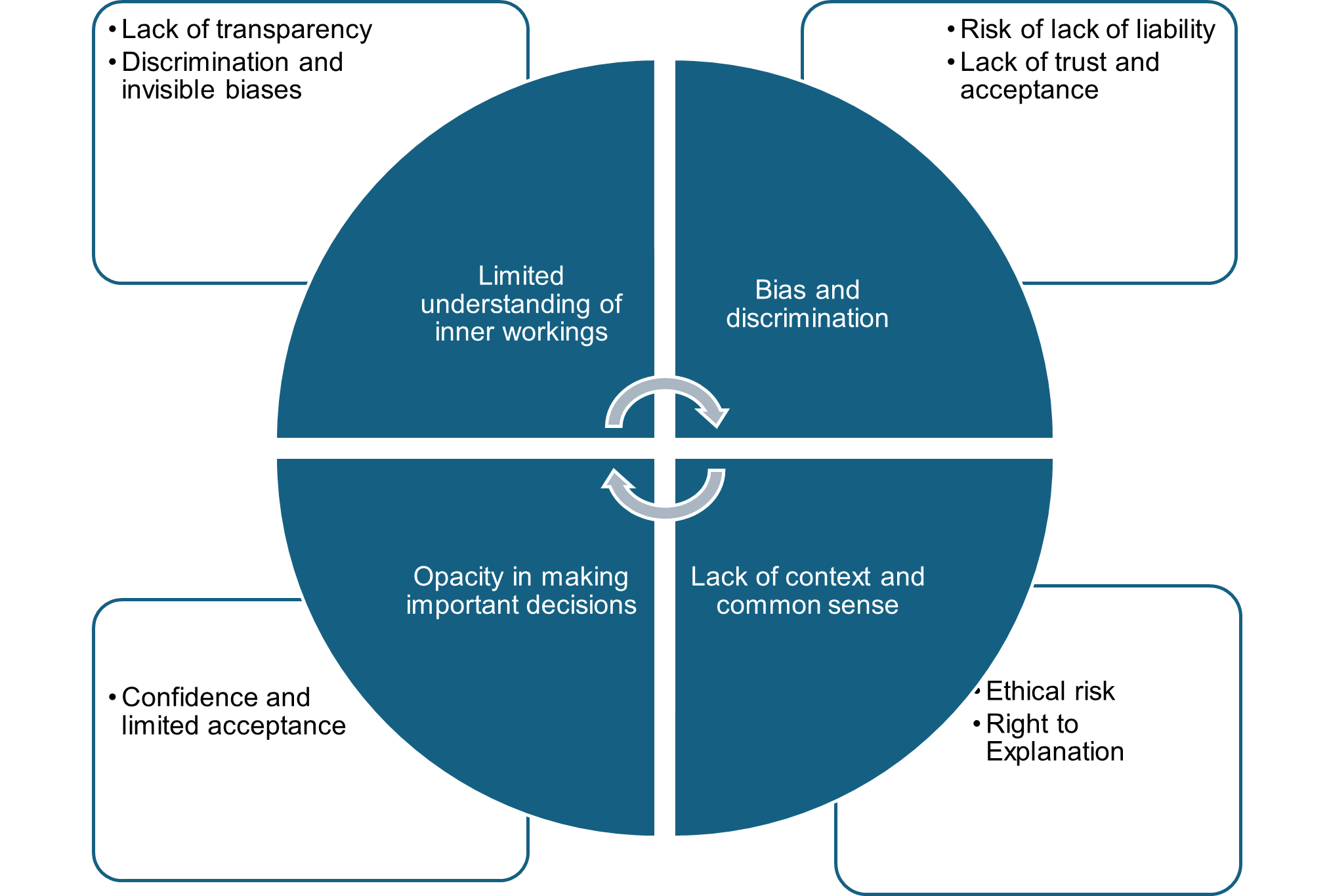
DOI:
https://doi.org/10.14483/23448393.21583Published:
2024-05-22Issue:
Vol. 29 No. 2 (2024): May-AugustSection:
Systems EngineeringInteligencia artificial explicable como principio ético
Explainable Artificial Intelligence as an Ethical Principle
Keywords:
Artificial intelligence, ethics, ethical principles, explainability, transparency, AI (en).Keywords:
inteligencia artificial, ética, principios éticos, explicabilidad, transparencia, IA (es).Downloads
Abstract (es)
Contexto: El avance de la inteligencia artificial (IA) ha traído numerosos beneficios en varios campos. Sin embargo, también plantea desafíos éticos que deben ser abordados. Uno de estos es la falta de explicabilidad en los sistemas de IA, i.e., la incapacidad de entender cómo la IA toma decisiones o genera resultados. Esto plantea preguntas sobre la transparencia y la responsabilidad de estas tecnologías. Esta falta de explicabilidad limita la comprensión de la manera en que los sistemas de IA llegan a ciertas conclusiones, lo que puede llevar a la desconfianza de los usuarios y afectar la adopción de tales tecnologías en sectores críticos (e.g., medicina o justicia). Además, existen dilemas éticos respecto a la responsabilidad y el sesgo en los algoritmos de IA.
Método: Considerando lo anterior, existe una brecha de investigación relacionada con estudiar la importancia de la IA explicable desde un punto de vista ético. La pregunta de investigación es ¿cuál es el impacto ético de la falta de explicabilidad en los sistemas de IA y cómo puede abordarse? El objetivo de este trabajo es entender las implicaciones éticas de este problema y proponer métodos para abordarlo.
Resultados: Nuestros hallazgos revelan que la falta de explicabilidad en los sistemas de IA puede tener consecuencias negativas en términos de confianza y responsabilidad. Los usuarios pueden frustrarse por no entender cómo se toma una decisión determinada, lo que puede llevarlos a desconfiar de la tecnología. Además, la falta de explicabilidad dificulta la identificación y la corrección de sesgos en los algoritmos de IA, lo que puede perpetuar injusticias y discriminación.
Conclusiones: La principal conclusión de esta investigación es que la IA debe ser éticamente explicable para asegurar la transparencia y la responsabilidad. Es necesario desarrollar herramientas y metodologías que permitan entender cómo funcionan los sistemas de IA y cómo toman decisiones. También es importante fomentar la colaboración multidisciplinaria entre expertos en IA, ética y derechos humanos para abordar este desafío de manera integral.
Abstract (en)
Context: The advancement of artificial intelligence (AI) has brought numerous benefits in various fields. However, it also poses ethical challenges that must be addressed. One of these is the lack of explainability in AI systems, i.e., the inability to understand how AI makes decisions or generates results. This raises questions about the transparency and accountability of these technologies. This lack of explainability hinders the understanding of how AI systems reach conclusions, which can lead to user distrust and affect the adoption of such technologies in critical sectors (e.g., medicine or justice). In addition, there are ethical dilemmas regarding responsibility and bias in AI algorithms.
Method: Considering the above, there is a research gap related to studying the importance of explainable AI from an ethical point of view. The research question is what is the ethical impact of the lack of explainability in AI systems and how can it be addressed? The aim of this work is to understand the ethical implications of this issue and to propose methods for addressing it.
Results: Our findings reveal that the lack of explainability in AI systems can have negative consequences in terms of trust and accountability. Users can become frustrated by not understanding how a certain decision is made, potentially leading to mistrust of the technology. In addition, the lack of explainability makes it difficult to identify and correct biases in AI algorithms, which can perpetuate injustices and discrimination.
Conclusions: The main conclusion of this research is that AI must be ethically explainable in order to ensure transparency and accountability. It is necessary to develop tools and methodologies that allow understanding how AI systems work and how they make decisions. It is also important to foster multidisciplinary collaboration between experts in AI, ethics, and human rights to address this challenge comprehensively.
References
F. Doshi-Velez and B. Kim, “Towards a rigorous science of interpretable machine learning,” arXiv preprint, 2017. arXiv:1702.08608.
M. Huang, V. K. Singh, and A. Mittal, Explainable AI: interpreting, explaining, and visualizing deep learning. Berlin, Germany: Springer, 2020.
A. Hanif, X. Zhang, and S. Wood, “A survey on explainable artificial intelligence techniques and challenges,” in IEEE 25th Int. Ent. Dist. Object Computing Workshop (EDOCW), 2021, pp. 81-89. https://doi.org/10.1109/EDOCW52865.2021.00036
M. Coeckelbergh, “Artificial intelligence, responsibility attribution, and a relational justification of explainability,” Sci. Eng. Ethics, vol. 26, no. 4, pp. 2051-2068, 2020. https://doi.org/10.1007/s11948-019-00146-8
T. Izumo and Y. H. Weng, “Coarse ethics: How to ethically assess explainable artificial intelligence,” AI Ethics, vol. 2, no. S1, pp. 1-13, 2021. https://doi.org/10.1007/s43681-021-00091-y
A. Das and P. Rad, “Opportunities and challenges in explainable artificial intelligence (XAI): A survey,” arXiv preprint, 2020. arXiv:2006.11371.
G. Adamson, “Ethics and the explainable artificial intelligence (XAI) movement,” TechRxiv, Preprint, 2022. https://doi.org/10.36227/techrxiv.20439192.v1
A. Barredo Arrieta, N. Díaz-Rodríguez, J. Del Ser, A. Bennetot, S. Tabik, A. Barbado, and F. Herrera, “Explainable artificial intelligence (XAI): Concepts, taxonomies, opportunities and challenges toward responsible AI,” Inf. Fus., vol. 58, pp. 82-115, 2019. https://doi.org/10.1016/j.inffus.2019.12.012
A. Weller and E. Almeida, “Principles of transparency, explainability, and interpretability in machine learning,” Cogn. Technol. Work, vol. 3, pp. 1-14, 2020.
A. Jobin, M. Ienca, and E. Vayena, “The global landscape of AI ethics guidelines,” Nat. Mach. Intell., vol. 1, no. 9, pp. 389-399, 2019. https://doi.org/10.1038/s42256-019-0088-2
L. H. Gilpin, D. Bau, B. Z. Yuan, A. Bajwa, M. Specter, and L. Kagal, “Explaining explanations: An overview of interpretability of machine learning,” in IEEE 5th Int. Conf. Data Sci. Adv. Analytics, 2018, pp. 80-89. https://doi.org/10.1109/DSAA.2018.00018
M. T. Ribeiro, S. Singh, and C. Guestrin, "Why should I trust you? Explaining the predictions of any classifier,” in 22nd ACM SIGKDD Int. Conf. Knowledge Discovery Data Mining, 2016, pp. 1135-1144. https://doi.org/10.1145/2939672.2939778
A. Weller and H. Aljalbout, “Explainable artificial intelligence: Understanding, visualizing and interpreting deep learning models,” JAIR, vol. 68, pp. 853-863, 2020.
Z. Lipton, “The mythos of model interpretability,” arXiv preprint, 2018. arXiv:1606.03490.
R. Guidotti, A. Monreale, S. Ruggieri, F. Turini, F. Giannotti, and D. Pedreschi, “A survey of methods for explaining black box models,” ACM Comput. Surv., vol. 51, no. 5, 1-42, 2018. https://doi.org/10.1145/3236009
A. Weller and S. V. Albrecht, “Challenges for transparency,” in Proceedings of the AAAI/ACM Conf. AI Ethics Soc., 2019, pp. 351-357.
H. Nissenbaum, Privacy in context: Technology, policy, and the integrity of social life. Stanford, CA, USA: Stanford University Press, 2009. https://doi.org/10.1515/9780804772891
B. D. Mittelstadt, P. Allo, M. Taddeo, S. Wachter, and L. Floridi, “The ethics of algorithms: Mapping the debate,” BD&S, vol. 3, no. 2, e2053951716679679, 2016. https://doi.org/10.1177/2053951716679679
J. Burrell, “How the machine 'thinks': Understanding opacity in machine learning algorithms,” BD&S, vol. 3, no. 1, e2053951715622512, 2016. https://doi.org/10.1177/2053951715622512
A. D. Selbst and S. Barocas, “The intuitive appeal of explainable machines,” Ford. Law Rev., vol. 87, e1085, 2018. https://doi.org/10.2139/ssrn.3126971
A. Weller and L. Floridi, “AIEthics Manifesto,” Min. Mach., vol. 29, no. 3, pp. 371-413, 2019.
V. Dignum, “Responsible artificial intelligence: How to develop and use AI in a responsible way,” ITU J. (Geneva), vol. 1, no. 6, pp. 1-8, 2021.
L. Floridi and M. Taddeo, “What is data ethics?” Phil. Trans. R. Soc. A, vol. 376, no. 2128, e20180083, 2018.
Z. Lipton, “The mythos of model interpretability,” arXiv preprint, 2016. arXiv:1606.03490.
C. Molnar, Interpretable machine learning, 2019. [Online]. Available: https://christophm.github.io/interpretable-ml-book/
Unión Europea, Reglamento general de protección de datos (GDPR), 2016. [Online]. Available: https://eur-
lex.europa.eu/eli/reg/2016/679/
European Commission, Ethics guidelines for trustworthy AI, 2019. [Online]. Available: https://ec.europa.eu/digital-single-
market/en/news/ethics-guidelines-trustworthy-ai
A. Brynjolfsson and A. McAfee, The second machine age: Work, progress, and prosperity in a time of brilliant technologies. New York, NY, USA: WW Norton & Company, 2014.
B. Green and S. Hassan, “Explaining explainability: A roadmap of challenges and opportunities of machine learning interpretability,” in 24th ACM SIGKDD Int. Conf. Knowledge Discovery Data Mining, 2019, pp. 2952-2953.
L. Floridi and J. W. Sanders, “On the morality of artificial agents,” Min. Mach., vol. 14, no. 3, pp. 349-379, 2004. https://doi.org/10.1023/B:MIND.0000035461.63578.9d
A. B. Arrieta, V. Dignum, R. Ghaeini, A. López, V. Murdock, M. Osborne, and A. Rathke, "Transparent AI: An overview," Art. Inte., vol. 290, pp. 1-43, 2020.
L. Liao, A. Anantharaman, and K. Pei, “On explaining individual predictions of machine learning models: An application to credit scoring,” arXiv preprint, 2018. arXiv:1810.04076.
How to Cite
APA
ACM
ACS
ABNT
Chicago
Harvard
IEEE
MLA
Turabian
Vancouver
Download Citation
License
Copyright (c) 2024 Mario González Arencibia, Hugo Ordoñez-Erazo, Juan-Sebastián González-Sanabria

This work is licensed under a Creative Commons Attribution-NonCommercial-ShareAlike 4.0 International License.
From the edition of the V23N3 of year 2018 forward, the Creative Commons License "Attribution-Non-Commercial - No Derivative Works " is changed to the following:
Attribution - Non-Commercial - Share the same: this license allows others to distribute, remix, retouch, and create from your work in a non-commercial way, as long as they give you credit and license their new creations under the same conditions.