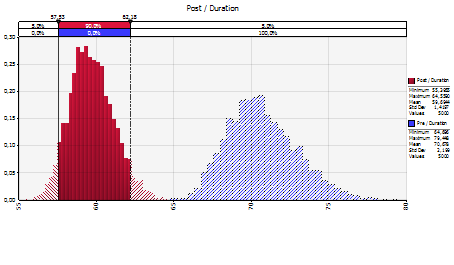
DOI:
https://doi.org/10.14483/23448393.21108Published:
2024-08-05Issue:
Vol. 29 No. 2 (2024): May-AugustSection:
Mechanical EngineeringMethodology for the Selection of Risk Response Actions while Considering Corporate Objectives in the Metalworking Industry
Metodología para la selección de acciones de respuesta a riesgos considerando los objetivos estratégicos en la industria metalmecánica
Keywords:
fuzzy logic, Monte Carlo simulation, project risk management, risk response actions (en).Keywords:
lógica difusa, simulación Monte Carlo, gestión de riesgos de proyectos, acciones de respuesta a riesgos (es).Downloads
Abstract (en)
Context: Projects in metalworking companies are affected by risk. Proper risk management depends on the responses provided to improve the project plan. However, multiple potential actions may result in constraints due to multiple factors. The purpose of this article is to propose a hybrid approach to solve the problem of selecting risk response actions while considering strategic objectives, fuzzy logic, and simulation.
Method: First, 334 risks were identified through a literature review and a discussion with experts. These were then filtered, resulting in 70 operational risks. Subsequently, the ten critical risks were prioritized using the risk matrix. Then, using Monte Carlo simulation and correlation analysis, the activities most affected by the risks were identified. Finally, potential response actions were designed for each case, and fuzzy logic and quality function deployment were applied to evaluate them.
Results: The selected responses were framed within the strategic objectives, i.e., customer satisfaction, business profitability, and implementation of new technologies. This, while considering some corporate attributes that the actions had to meet finishing the project on time, having low costs, and meeting the scope. The selected actions had a better profile than others seeking to minimize time or costs.
Conclusions: EPCC projects are complex and often suffer from gaps in scope, time, and cost. Risk analysis and the selection of responses in the planning phase help to improve performance. This study developed a risk response plan for a project executed in Brazil. Risks were identified, classified, and mitigated using simulations, resulting in an 11-day reduction in the project’s estimated duration.
Abstract (es)
Contexto: Los proyectos en empresas metalmecánicas se ven afectados por el riesgo. Una correcta gestión de riesgos depende de las respuestas que se brinden para mejorar el plan del proyecto. Sin embargo, múltiples acciones potenciales pueden resultar en restricciones por múltiples factores. El propósito de este artículo es proponer un enfoque híbrido para resolver el problema de seleccionar acciones de respuesta a riesgos considerando objetivos estratégicos, lógica difusa y simulación.
Método: Primero, se identificaron 334 riesgos mediante una revisión de la literatura y una discusión con expertos. Estos fueron filtrados, lo que resultó en 70 riesgos operacionales. Posteriormente, se priorizaron los 10 riesgos críticos utilizando la matriz de riesgos. Luego, mediante simulación Monte Carlo y análisis de correlación, se identificaron las actividades más afectadas por los riesgos. Finalmente, se diseñaron potenciales acciones de respuesta para cada caso, y se aplicó lógica difusa y despliegue de funciones de calidad para evaluarlas.
Resultados: Las respuestas seleccionadas se enmarcaron en los objetivos estratégicos, i.e., satisfacción del cliente, rentabilidad del negocio, e implementación de nuevas tecnologías. Esto, teniendo en cuenta algunos atributos corporativos que las acciones debían cumplir: finalizar a tiempo el proyecto, tener costos bajos y cumplir con el alcance. Las acciones seleccionadas tuvieron un mejor perfil que otras opciones que buscaban minimizar tiempo o costos.
Conclusiones: Los proyectos EPCC son complejos y a menudo sufren de desfases en alcance, tiempo y costo. El análisis de los riesgos y la selección de las respuestas en la fase de planificación ayudan a un mejor desempeño. Este estudio desarrolló un plan de respuesta a riesgos para un proyecto desarrollado en Brasil. Los riesgos fueron identificados, clasificados y mitigados mediante simulaciones, lo que resultó en una reducción de 11 días en la duración estimada del proyecto.
References
S. Mittal and N. Gorowara, “Knowledge Integration in engineering, procurement and construction projects: A conceptual study,” Psychol. Educ. J., vol. 58, no. 1, pp. 5733–5738, 2021. https://doi.org/10.17762/PAE.V58I1.2209
H. Erol, I. Dikmen, G. Atasoy, M. Talat Birgonul, and M. T. Birgonul, “An analytic network process model for risk quantification of mega construction projects,” Expert Syst Appl, vol. 191, art. 116215, 2022. https://doi.org/10.1016/J.ESWA.2021.116215
E. Oliveira and C. Santos, “Application of a risk management methodology in industrial projects: A case study in the metalworking sector,” in Educ. Excellence Innov. Manag. Vision 2020 App., 2019, pp. 5647–5662, Online]. Available: https://repositorium.sdum.uminho.pt/bitstream/1822/61217/1/Application%20of%20a%20Risk%20Management%20Methodology%20in%20Industrial%20projects.pdf.
A. Birjandi and S. M. Mousavi, “Fuzzy resource-constrained project scheduling with multiple routes: A heuristic solution,” Autom. Constr., vol. 100, pp. 84–102, Apr. 2019. https://doi.org/10.1016/j.autcon.2018.11.029
J. Silva et al., “Improvement of planning and time control in the project management of a metalworking industry - Case study,” Procedia Comput. Sci., vol. 196, no. 2021, pp. 288–295, 2021. https://doi.org/10.1016/j.procs.2021.12.016
PricewaterhouseCoopers, “En la ruta de la Competitividad. Principales hallazgos de la 1ra Encuesta Nacional de Madurez en Gerencia de Proyectos,” PwC, Bogotá, Colombia, 2011.
S. Changali, A. Mohammad, and M. Van Niewlan, “The construction productivity imperative,” 2015. [Online]. Available: https://www.mckinsey.com/~/media/mckinsey/business functions/operations/our insights/the construction productivity imperative/the construction productivity imperative.pdf
PMI, A guide to the Project Management Body of Knowledge (PMBOK Guide), 6th, 2017.
L. Bai, Q. Xie, J. Lin, S. Liu, C. Wang, and L. Wang, “Dynamic selection of risk response strategies with resource allocation for construction project portfolios,” Comput. Ind. Eng., vol. 191, p. 110116, May 2024. https://doi.org/10.1016/j.cie.2024.110116
Y. Zhang and F. Zuo, “Selection of risk response actions considering risk dependency,” Kybernetes, vol. 45, no. 10, pp. 1652–1667, Nov. 2016. https://doi.org/10.1108/K-05-2016-0096
APM, Association for Project Management Body of Knowledge, 6th., 2012.
OGC, Managing successful projects with PRINCE2, 6th ed., 2017.
M. R. Gleim, H. McCullough, N. Sreen, and L. G. Pant, “Is doing right all that matters in sustainability marketing? The role of fit in sustainable marketing strategies,” J. Retail. Consum. Serv., vol. 70, 2023, art. 103124. https://doi.org/10.1016/j.jretconser.2022.103124
W. Stevenson, Operations Management, 14th ed., New York, NY, USA: McGraw-Hill Education, 2021.
R. F. Jacobs and R. B. Chase, Administracion de operaciones: produccion y cadena de suministros, 15th ed., New York, NY, USA: McGraw-Hill Education, 2019. https://book4you.org/book/11172205/6d75a9
PMI, "The standard for portfolio management," 2017. [Online]. Available: https://www.pmi.org/pmbok-guide-standards/foundational/standard-for-portfolio-management
L. E. Dounavi, E. Dermitzakis, G. Chatzistelios, and K. Kirytopoulos, “Project management for corporate events: A set of tools to manage risk and increase quality outcomes,” Sustainability, vol. 14, no. 4, pp. 1–37, 2022. https://doi.org/10.3390/SU14042009
M. Hamid, A. M. Abdelalim, M. Abdel, H. Hassanen, and A. M. Abdelalim, “Risk identification and assessment of mega industrial projects in Egypt,” Int. J. Manag. Commer. Innov., vol. 10, no. 1, pp. 187–199, 2022. https://doi.org/10.5281/zenodo.6579176
S. Bakri et al., “Identification of factors influencing time and cost risks in highway construction projects,” Int. J. Sustain. Constr. Eng. Technol., vol. 12, no. 3, pp. 280–288, 2021. https://doi.org/10.30880/ijscet.2021.12.03.027
T. Yuan, P. Xiang, H. Li, and L. Zhang, “Identification of the main risks for international rail construction projects based on the effects of cost-estimating risks,” J. Clean. Prod., vol. 274, p. 122904, 2020. https://doi.org/10.1016/j.jclepro.2020.122904
A. Nurdiana, M. Agung Wibowo, Y. Fundra Kurnianto, M. A. Wibowo, and Y. F. Kurnianto, “The identification of risk factors of delay on the road construction project in indonesia,” in Int. Conf. Maritime Archipelago (ICoMA 2018), 2019, pp. 384–387. https://doi.org/10.2991/ICOMA-18.2019.82
M. H. Kotb and M. M. Ghattas, “Risk identification barriers in construction projects in MENA,” PMI World J., 2018. https://doi.org/10.13140/RG.2.2.20614.83525.
S. Maulana and F. D. Ariyanti, “Application of lean project management method in environmental drainage development case study: x area Bekasi City,” IOP Conf. Ser. Mater. Sci. Eng., vol. 1096, no. 1, art. 12085, 2021. https://doi.org/10.1088/1757-899X/1096/1/012085
J. Chilumo et al., “Risk management practices on performance of building construction projects,” J. Entrep. Proj. Manag., vol. 4, no. 6, art. 202, 2020. https://stratfordjournals.org/journals/index.php/journal-of-entrepreneurship-proj/article/view/659
J. Crispim, L. H. Silva, and N. Rego, “Project risk management practices: The organizational maturity influence,” Int. J. Manag. Proj. Bus., vol. 12, no. 1, pp. 187-210, 2018. https://doi.org/10.1108/IJMPB-10-2017-0122
E. Prihartanto and M. D. Bakri, “Identification the highest risk of performance based contract in Bojonegoro-Padangan road projects,” in Reg. Conf. Civil Eng. RCCE, Surabaya, Indonesia, 2017. http://dx.doi.org/10.12962/j23546026.y2017i6.3243
D. K. Sudarsana, “A concept model to scale the impact of safety risk in a construction project using a semi quantitative method,” Civ. Eng. Archit., vol. 9, no. 1, pp. 263–269, 2021. https://doi.org/10.13189/cea.2021.090122
Ayuningtyas, D. and Dita Rarasati, A, “Work acceleration strategy development on design-build project to improve risk based quality performance,” Glob. J. Sci. Eng., vol. 02, pp. 10–15, 2020. https://doi.org/10.37516/global.j.sci.eng.2020.007
L. Wu, H. Bai, C. Yuan, and C. Xu, “FANPCE technique for risk assessment on subway station construction,” J. Civ. Eng. Manag., vol. 25, no. 6, pp. 599–616, 2019. https://doi.org/10.3846/JCEM.2019.10373
B. Barghi, S. S. sikari, and S. Shadrokh sikari, “Qualitative and quantitative project risk assessment using a hybrid PMBOK model developed under uncertainty conditions,” Heliyon, vol. 6, no. 1, art. 3097, Jan. 2020. https://doi.org/10.1016/J.HELIYON.2019.E03097
M. Kaut, H. Vaagen, and S. W. Wallace, “The combined impact of stochastic and correlated activity durations and design uncertainty on project plans,” Int. J. Prod. Econ., vol. 233, art. 108015, 2020. https://doi.org/10.1016/J.IJPE.2020.108015
M. Eckhart, B. Brenner, A. Ekelhart, and E. Weippl, “Quantitative security risk assessment for industrial control systems: Research opportunities and challenges,” J. Internet Serv. Inf. Secur. (JISIS)2, vol. 09, no. 03, pp. 52–73, 2019. https://doi.org/10.22667/JISIS.2019.08.31.052.
N.-T. Nguyen, Q.-T. Huynh, and T.-H.-G. Vu, “A Bayesian critical path method for managing common risks in software project scheduling,” in Ninth Int. Sym. Info. Comm. Tech. - SoICT 2018, 2018, pp. 382–388. https://doi.org/10.1145/3287921.3287962
X. Xu, J. Wang, C. Z. Li, W. Huang, and N. Xia, “Schedule risk analysis of infrastructure projects: A hybrid dynamic approach,” Autom. Constr., vol. 95, pp. 20–34, 2018. https://doi.org/10.1016/j.autcon.2018.07.026
M. Alipour-Bashary, M. Ravanshadnia, H. Abbasianjahromi, and E. Asnaashari, “Building demolition risk assessment by applying a hybrid fuzzy FTA and fuzzy CRITIC-TOPSIS framework,” Int. J. Build. Pathol. Adapt., vol. 40, no. 1, pp. 134–159, 2022. https://doi.org/10.1108/IJBPA-08-2020-0063
A. B. Ashkezari, M. Zokaee, A. Aghsami, F. Jolai, and M. Yazdani, “Selecting an appropriate configuration in a construction project using a hybrid multiple attribute decision making and failure analysis methods,” Buildings, vol. 12, no. 5, art. 0643. 2022. https://doi.org/10.3390/buildings12050643
S. Pehlivan and A. E. Öztemir, "Integrated risk of progress-based costs and schedule delays in construction projects," Eng. Manag. J., vol. 30, no. 2. 2018, pp. 108–116. https://doi.org/10.1080/10429247.2018.1439636
B. Yan, J. Wu, and F. Wang, “CVaR-based risk assessment and control of the agricultural supply chain,” Manag. Decis., vol. 57, no. 7, pp. 1496–1510, Jul. 2018. https://doi.org/10.1108/MD-11-2016-0808/FULL/XML
E. Cheraghi, M. Khalilzadeh, S. Shojaei, and S. Zohrehvandi, “A mathematical model to select the risk response strategies of the construction projects: Case study of Saba Tower,” Procedia Comput. Sci., vol. 121, pp. 609–616, 2017. https://doi.org/10.1016/j.procs.2017.11.080
R. Soofifard and M. Bafruei, “An optimal model for project risk response portfolio selection (P2RPS) (Case study: Research institute of petroleum industry),” Iran. J. Manag. Stud., vol. 9, no. 4, pp. 741–765, 2016. https://doi.org/10.22059/ijms.2017.59374
Y. Zhang, “Selecting risk response strategies considering project risk interdependence,” Int. J. Proj. Manag., vol. 34, no. 5, pp. 819–830, 2016. https://doi.org/10.1016/j.ijproman.2016.03.001
Z.-P. Fan, Y.-H. Li, and Y. Zhang, “Generating project risk response strategies based on CBR: A case study,” Expert Syst. Appl., vol. 42, no. 6, pp. 2870–2883, 2015. https://doi.org/10.1016/j.eswa.2014.11.034
F. Marmier, I. Filipas Deniaud, D. Gourc, I. F. Deniaud, and D. Gourc, “Strategic decision-making in NPD projects according to risk: Application to satellites design projects,” Comput. Ind., vol. 65, no. 8, pp. 1107–1114, Oct. 2014. https://doi.org/10.1016/J.COMPIND.2014.06.001
S. Jordan, L. Jørgensen, and H. Mitterhofer, “Performing risk and the project: Risk maps as mediating instruments,” Manag. Account. Res., vol. 24, no. 2, pp. 156–174, Jun. 2013. https://doi.org/10.1016/J.MAR.2013.04.009
S. Goh and H. Abdul-rahman, “The Identification and management of major risks in the Malaysian construction industry,” J. Constr. Dev. Ctries., vol. 18, no. 1, pp. 19–32, 2013. [Online]. Available: https://core.ac.uk/download/pdf/199244814.pdf
A. Pla, B. López, P. Gay, and C. Pous, “eXiT*CBR.v2: Distributed case-based reasoning tool for medical prognosis,” Decis. Support Syst., vol. 54, no. 3, pp. 1499–1510, Feb. 2013. https://doi.org/10.1016/J.DSS.2012.12.033
L.-C. Ma, “Screening alternatives graphically by an extended case-based distance approach,” Omega, vol. 40, no. 1, pp. 96–103, Jan. 2012. https://doi.org/10.1016/J.OMEGA.2011.03.010
E. Kujawski and D. Angelis, “Monitoring risk response actions for effective project risk management,” Syst. Eng., vol. 13, no. 4, pp. 353–368, 2010. https://doi.org/10.1002/sys.20154
S. M. Seyedhoseini, S. Noori, and M. A. Hatefi, “An integrated methodology for assessment and selection of the project risk response actions,” Risk Anal., vol. 29, no. 5, pp. 752-753, 2009. https://doi.org/10.1111/j.1539-6924.2008.01187.x
A. Öztaş and Ö. Ökmen, “Judgmental risk analysis process development in construction projects,” Build. Environ., vol. 40, no. 9, pp. 1244–1254, 2005. https://doi.org/10.1016/j.buildenv.2004.10.013
M. Vanhoucke, Integrated Project Management Sourcebook, vol. 2, CHam, Germany: Springer International Publishing, 2016. https://doi.org/10.1007/978-3-319-27373-0
I. Wallace, “Schedule risk and contingency using @ RISK and probabilistic analysis,” 2010. [Online]. Available: https://www.palisade.com/downloads/pdf/Wallace_Schedule_Risk.pdf
J. Song, A. Martens, and M. Vanhoucke, “Using schedule risk analysis with resource constraints for project control,” Eur. J. Oper. Res., vol. 288, pp. 736–752, 2020. https://doi.org/10.1016/j.ejor.2020.06.015
A. Öztaş and Ö. Ökmen, “Construction project network evaluation with correlated schedule risk analysis model,” J. Constr. Eng. Manag., no. 1, pp. 49–63, 2008. https://doi.org/10.1061/(ASCE)0733-9364(2008)134:1(49)
A. Grando, V. Beldevere, S. Raffaele, and G. Stabilini, Production, Operations and Supply Chain Management,1st ed., Milan, Italy: Bocconi University Press, 2021.
H. Jafarzadeh, P. Akbari, and B. Abedin, “A methodology for project portfolio selection under criteria prioritisation, uncertainty and projects interdependency – Combination of fuzzy QFD and DEA,” Expert Syst. Appl., vol. 110, pp. 237–249, Nov. 2018. https://doi.org/10.1016/J.ESWA.2018.05.028
J. Osorio, M. Peña, and D. Arias, “Priorización de despachos en empresas de manufactura usando QFD difuso,” Rev. Ing. Univ. Medellín, vol. 17, pp. 173–186, 2018. https://doi.org/10.22395/rium.v17n33a9
J. Osorio, D. Manotas, and J. García, “Operational risk assessment in 3PL for maritime transportation,” Res. Comput. Sci., vol. 132, pp. 63–69, 2017. https://doi.org/10.13053/rcs-132-1-6
S. S. Agarwal and M. L. Kansal, “Risk based initial cost assessment while planning a hydropower project,” Energy Strateg. Rev., vol. 31, art. 100517, 2020. https://doi.org/10.1016/j.esr.2020.100517
León, R,. Scaco, E. and Galiano, N. “Identificación de factores de riesgo operativo en el sector metalmecánico manufacturero,” Rev. Espacios, vol. 40, no. 20, p. 23, 2019. [Online]. Available: https://www.revistaespacios.com/a19v40n20/a19v40n20p23.pdf
J. Liu, F. Jin, Q. Xie, and M. Skitmore, “Improving risk assessment in financial feasibility of international engineering projects: A risk driver perspective,” Int. J. Proj. Manag., vol. 35, no. 2, pp. 204–211, 2017. https://doi.org/10.1016/j.ijproman.2016.11.004
M. J. Naude and N. Chiweshe, “A proposed operational risk management framework for small and medium enterprises,” South African J. Econ. Manag. Sci., vol. 20, no. 1, pp. 1–10, 2017. https://doi.org/10.4102/sajems.v20i1.1621
J. Osorio, D. Manotas, and L. Rivera, “Priorización de riesgos operacionales para un proveedor de tercera parte logística - 3PL,” Inf. Tecnol., vol. 28, no. 4, pp. 135–144, 2017. https://doi.org/10.4067/S0718-07642017000400016
J. R. Ríos, D. Manotas, and J. C. Osorio, “Operational supply chain risk identification and prioritization using the SCOR model,” Ing. Univ., vol. 23, no. 1, pp. 1–20, 2019. https://doi.org/10.11144/Javeriana.iyu23-1.oscr
F. Romero. (2017), "El riesgo operativo y su influencia en el crecimiento empresarial del sector metalmecánica - Puente Piedra (lado sur)" M.S. thesis, Facultad de Ciencias Empresariales, Universidad Cesar Vallejo, Lima, Perú, 2017.
F. Sánchez, S. Steria, E. Bonjour, J.-P. P. J.-P. P. Micaelli, and D. Monticolo, “An approach based on bayesian network for improving project management maturity: An application to reduce cost overrun risks in engineering projects,” Comput. Ind., vol. 119, art. 103227, 2020. https://doi.org/10.1016/j.compind.2020.103227
How to Cite
APA
ACM
ACS
ABNT
Chicago
Harvard
IEEE
MLA
Turabian
Vancouver
Download Citation
License
Copyright (c) 2024 Álvaro Julio Cuadros-López, Alexander Bustos-Useche, Leonardo Bustos-Useche

This work is licensed under a Creative Commons Attribution-NonCommercial-ShareAlike 4.0 International License.
From the edition of the V23N3 of year 2018 forward, the Creative Commons License "Attribution-Non-Commercial - No Derivative Works " is changed to the following:
Attribution - Non-Commercial - Share the same: this license allows others to distribute, remix, retouch, and create from your work in a non-commercial way, as long as they give you credit and license their new creations under the same conditions.