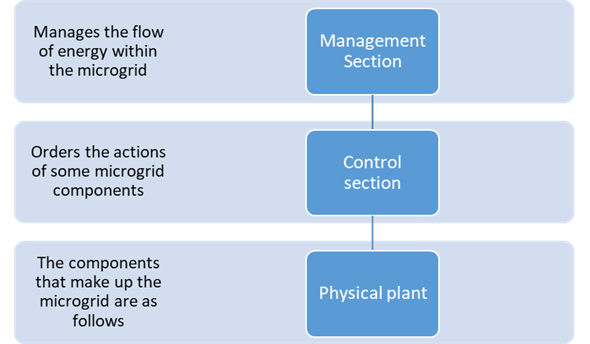
DOI:
https://doi.org/10.14483/23448393.19777Published:
2024-01-13Issue:
Vol. 29 No. 1 (2024): January-AprilSection:
Electrical, Electronic and Telecommunications EngineeringEnergy Management Systems for Microgrids: Evolution and Challenges within the Framework of the Energy Transition
Sistemas de gestión de energía para microrredes: evolución y desafíos en el marco de la transición energética
Keywords:
Microgrid, management system, input and output variables (en).Keywords:
microrred, sistema de gestión, variables de entrada y salida (es).Downloads
References
Z. Shuai et al., “Microgrid stability: Classification and a review,” Renew. Sustain. Energy Rev., vol. 58, pp. 167-179, 2016. https://doi.org/10.1016/j.rser.2015.12.201 DOI: https://doi.org/10.1016/j.rser.2015.12.201
O. O. Approach, K. Rahbar, S. Member, J. Xu, and R. Zhang, “Real-time energy storage management for renewable integration in microgrid: An off-line optimization approach,” IEEE Trans. Smart Grid, vol. 6, no. 1, pp. 124-134, 2015. https://doi.org/10.1109/TSG.2014.2359004 DOI: https://doi.org/10.1109/TSG.2014.2359004
C. L. Nge, I. U. Ranaweera, O. M. Midtgård, and L. Norum, “A real-time energy management system for smart grid integrated photovoltaic generation with battery storage,” Renew. Energy, vol. 130, pp. 774-785, 2019. https://doi.org/10.1016/j.renene.2018.06.073 DOI: https://doi.org/10.1016/j.renene.2018.06.073
W. El-Baz, P. Tzscheutschler, and U. Wagner, “Integration of energy markets in microgrids: A double-sided auction with device-oriented bidding strategies,” Appl. Energy, vol. 241, pp. 625-639, 2019. https://doi.org/10.1016/j.apenergy.2019.02.049 DOI: https://doi.org/10.1016/j.apenergy.2019.02.049
K. Markov and N. Rajaković, “Multi-energy microgrids with ecotourism purposes: The impact of the power market and the connection line,” Energy Convers. Manag., vol. 196, pp. 1105-1112, 2019. https://doi.org/10.1016/j.enconman.2019.05.048 DOI: https://doi.org/10.1016/j.enconman.2019.05.048
T. Ahmad and D. Zhang, “A critical review of comparative global historical energy consumption and future demand: The story told so far,” Energy Reports, vol. 6, pp. 1973-1991, 2020. https://doi.org/10.1016/j.egyr.2020.07.020 DOI: https://doi.org/10.1016/j.egyr.2020.07.020
L. Miller and R. Carriveau, “Energy demand curve variables – An overview of individual and systemic e ff ects,” Sustain. Energy Technol. Assessments, vol. 35, pp. 172-179, 2019. https://doi.org/10.1016/j.seta.2019.07.006 DOI: https://doi.org/10.1016/j.seta.2019.07.006
R. S. Jayashree and P. J. A. Kenis, “Micro fuel cells for portable applications,” AIChE Annu. Meet. Conf. Proc., no. 42, art. 3390, 2005.
E. Bullich-massagué, F. Díaz-gonzález, M. Aragüés-peñalba, F. Girbau-llistuella, P. Olivella-rosell, and A. Sumper, “Microgrid clustering architectures,” Appl. Energy, vol. 212, pp. 340-361, 2018. https://doi.org/10.1016/j.apenergy.2017.12.048 DOI: https://doi.org/10.1016/j.apenergy.2017.12.048
J. Shen, C. Jiang, Y. Liu, and X. Wang, “A microgrid energy management system and risk management under an electricity market environment,” IEEE Access, vol. 4, pp. 2349-2356, 2016. https://doi.org/10.1109/ACCESS.2016.2555926 DOI: https://doi.org/10.1109/ACCESS.2016.2555926
D. Giaouris et al., “A systems approach for management of microgrids considering multiple energy carriers, stochastic loads, forecasting and demand side response,” Appl. Energy, vol. 226, pp. 546-559, 2018. https://doi.org/10.1016/j.apenergy.2018.05.113 DOI: https://doi.org/10.1016/j.apenergy.2018.05.113
D. Petreus, R. Etz, T. Patarau, and M. Cirstea, “An islanded microgrid energy management controller validated by using hardware-in-the-loop emulators,” Int. J. Electr. Power Energy Syst., vol. 106,. 346-357, 2019. https://doi.org/10.1016/j.ijepes.2018.10.020 DOI: https://doi.org/10.1016/j.ijepes.2018.10.020
W. Su and J. Wang, “Energy management systems in microgrid operations,” Electr. J., vol. 25, no. 8, pp. 45-60, 2012. https://doi.org/10.1016/j.tej.2012.09.010 DOI: https://doi.org/10.1016/j.tej.2012.09.010
L. A. Dao, A. Dehghani-Pilehvarani, A. Markou, and L. Ferrarini, “A hierarchical distributed predictive control approach for microgrids energy management,” Sustain. Cities Soc., vol. 48, art. 101536, 2019. https://doi.org/10.1016/j.scs.2019.101536 DOI: https://doi.org/10.1016/j.scs.2019.101536
T. Morstyn, B. Hredzak, and V. G. Agelidis, “Control strategies for microgrids with distributed energy storage systems: An overview,” IEEE Trans. Smart Grid, vol. 9, no. 4, pp. 3652-3666, 2018. https://doi.org/10.1109/TSG.2016.2637958 DOI: https://doi.org/10.1109/TSG.2016.2637958
M. F. Zia, E. Elbouchikhi, and M. Benbouzid, “Microgrids energy management systems: A critical review on methods, solutions, and prospects,” Appl. Energy, vol. 222, pp. 1033-1055, 2018. https://doi.org/10.1016/j.apenergy.2018.04.103 DOI: https://doi.org/10.1016/j.apenergy.2018.04.103
T. Dragicevic, X. Lu, J. C. Vasquez, and J. M. Guerrero, “DC microgrids - Part I: A review of control strategies and stabilization techniques,” IEEE Trans. Power Electron., vol. 31, no. 7, pp. 4876-4891, 2016. https://doi.org/10.1109/TPEL.2015.2478859 DOI: https://doi.org/10.1109/TPEL.2015.2478859
J. Pascual, J. Barricarte, P. Sanchis, and L. Marroyo, “Energy management strategy for a renewable-based residential microgrid with generation and demand forecasting,” Appl. Energy, vol. 158, pp. 12-25, 2015. https://doi.org/10.1016/j.apenergy.2015.08.040 DOI: https://doi.org/10.1016/j.apenergy.2015.08.040
International Electrotechnical Commission, “IEC 61970-1:2005 | IEC Webstore | automation, cyber security, smart city, smart energy, smart grid,” pp 5-7 2005. https://webstore.iec.ch/publication/6208 (accessed Oct. 16, 2020).
C. C. S. Duan and T. C. B. Liu, “Smart energy management system for optimal microgrid economic operation,” IET Rene. Power Gen., vol. 5, no. 3, pp. 258-267, 2011. https://doi.org/10.1049/iet-rpg.2010.0052 DOI: https://doi.org/10.1049/iet-rpg.2010.0052
A. Ahmad Khan, M. Naeem, M. Iqbal, S. Qaisar, and A. Anpalagan, “A compendium of optimization objectives, constraints, tools and algorithms for energy management in microgrids,” Renew. Sustain. Energy Rev., vol. 58, pp. 1664-1683, 2016. https://doi.org/10.1016/j.rser.2015.12.259 DOI: https://doi.org/10.1016/j.rser.2015.12.259
M. Hu, J. W. Xiao, S. C. Cui, and Y. W. Wang, “Distributed real-time demand response for energy management scheduling in smart grid,” Int. J. Electr. Power Energy Syst., vol. 99, pp. 233-245, 2018. https://doi.org/10.1016/j.ijepes.2018.01.016 DOI: https://doi.org/10.1016/j.ijepes.2018.01.016
M. Elsied, A. Oukaour, T. Youssef, H. Gualous, and O. Mohammed, “An advanced real time energy management system for microgrids,” Energy, vol. 114, pp. 742-752, 2016. https://doi.org/10.1016/j.energy.2016.08.048 DOI: https://doi.org/10.1016/j.energy.2016.08.048
K. Say, M. John, and R. Dargaville, “Power to the people : Evolutionary market pressures from residential PV battery investments in Australia,” Energy Policy, vol. 134, art. 110977, 2019. https://doi.org/10.1016/j.enpol.2019.110977 DOI: https://doi.org/10.1016/j.enpol.2019.110977
T. R Nudell, M. Brignone, M. Robba, A. Bonfiglio, F. Delfino, and A. Annaswamy, “A dynamic market mechanism for combined heat and power microgrid energy management,” IFAC-PapersOnLine, vol. 50, no. 1, pp. 10033-10039, 2017. https://doi.org/10.1016/j.ifacol.2017.08.2040 DOI: https://doi.org/10.1016/j.ifacol.2017.08.2040
S. Chalise, J. Sternhagen, T. M. Hansen, and R. Tonkoski, “Energy management of remote microgrids considering battery lifetime,” Electr. J., vol. 29, no. 6, pp. 1-10, 2016. https://doi.org/10.1016/j.tej.2016.07.003 DOI: https://doi.org/10.1016/j.tej.2016.07.003
J. Shen, C. Jiang, Y. Liu, and J. Qian, “Electric power components and systems a microgrid energy management system with demand response for providing grid peak shaving a microgrid energy management system with demand response for providing grid peak shaving,” Elec. Power Comp. Syst., vol. 44, no. 8, 2016. https://doi.org/10.1080/15325008.2016.1138344 DOI: https://doi.org/10.1080/15325008.2016.1138344
S. Sukumar, H. Mokhlis, S. Mekhilef, and K. Naidu, “Mix-mode energy management strategy and battery sizing for economic operation of grid-tied microgrid,” Energy, vol. 118, pp. 1322-1333, 2017. https://doi.org/10.1016/j.energy.2016.11.018 DOI: https://doi.org/10.1016/j.energy.2016.11.018
G. Comodi et al., “Multi-apartment residential microgrid with electrical and thermal storage devices: Experimental analysis and simulation of energy management strategies,” Appl. Energy, vol. 137, pp. 854-866, 2015. https://doi.org/10.1016/j.apenergy.2014.07.068 DOI: https://doi.org/10.1016/j.apenergy.2014.07.068
M. H. Amrollahi, S. Mohammad, and T. Bathaee, “Techno-economic optimization of hybrid photovoltaic / wind generation together with energy storage system in a stand-alone micro-grid subjected to demand response,” Appl. Energy, vol. 202, pp. 66-77, 2017. https://doi.org/10.1016/j.apenergy.2017.05.116 DOI: https://doi.org/10.1016/j.apenergy.2017.05.116
M. Střelec and J. Berka, "Microgrid energy management based on approximate dynamic programming," in IEEE PES ISGT Europe 2013, Lyngby, Denmark, 2013, pp. 1-5. https://doi.org/10.1109/ISGTEurope.2013.6695439 DOI: https://doi.org/10.1109/ISGTEurope.2013.6695439
S. Golshannavaz, S. Afsharnia, and P. Siano, “A comprehensive stochastic energy management system in reconfigurable microgrids,” Int. J. Energy, Res., vol. 40, no. 11, pp. 1518-1531, 2016. https://doi.org/10.1002/er.3536 DOI: https://doi.org/10.1002/er.3536
J. Radosavljevi, M. Jevti, and D. Klimenta, “Engineering optimization energy and operation management of a microgrid using particle swarm optimization,” Energy Opt., vol. 48, no. 5, pp. 811-813, 2015. https://doi.org/10.1080/0305215X.2015.1057135 DOI: https://doi.org/10.1080/0305215X.2015.1057135
M. Marzband, E. Yousefnejad, A. Sumper, and J. L. Domínguez-García, "Real time experimental implementation of optimum energy management system in stand-alone Microgrid by using multi-layer ant colony optimization," Int. J. Electr. Power Energy Syst., vol. 75, pp. 265-274, 2016. https://doi.org/10.1016/j.ijepes.2015.09.010 DOI: https://doi.org/10.1016/j.ijepes.2015.09.010
M. Marzband, M. Ghadimi, A. Sumper, and J. L. Domínguez-garcía, “Experimental validation of a real-time energy management system using multi-period gravitational search algorithm for microgrids in islanded mode,” Appl. Energy, vol. 128, pp. 164-174, 2014. https://doi.org/10.1016/j.apenergy.2014.04.056 DOI: https://doi.org/10.1016/j.apenergy.2014.04.056
D. Arcos-Aviles, J. Pascual, F. Guinjoan, L. Marroyo, P. Sanchis, and M. P. Marietta, “Low complexity energy management strategy for grid profile smoothing of a residential grid-connected microgrid using generation and demand forecasting,” Appl. Energy, vol. 205, pp. 69-84, 2017. https://doi.org/10.1016/j.apenergy.2017.07.123 DOI: https://doi.org/10.1016/j.apenergy.2017.07.123
Y.-K. Chen, Y.-C. Wu, C.-C. Song, and Y.-S. Chen, "Design and implementation of energy management system with fuzzy control for DC microgrid systems," IEEE Trans. Power Elect., vol. 28, no. 4, pp. 1563-1570, April 2013. https://doi.org/10.1109/TPEL.2012.2210446 DOI: https://doi.org/10.1109/TPEL.2012.2210446
T. Bogaraj and J. Kanakaraj, “Intelligent energy management control for independent microgrid,” Sadhana - Acad. Proc. Eng. Sci., vol. 41, no. 7, pp. 755-769, 2016. https://doi.org/10.1007/s12046-016-0515-6 DOI: https://doi.org/10.1007/s12046-016-0515-6
A. Anvari-moghaddam, A. Rahimi-kian, M. S. Mirian, and J. M. Guerrero, “A multi-agent based energy management solution for integrated buildings and microgrid system,” Appl. Energy, vol. 203, pp. 41-56, 2017. https://doi.org/10.1016/j.apenergy.2017.06.007 DOI: https://doi.org/10.1016/j.apenergy.2017.06.007
C. Corchero and M. Cruz-zambrano, “Optimal energy management for a residential microgrid including a vehicle-to-grid system,” IEEE Trans. Smart Grid, vol. 5, no. 4, pp. 2163-2172, 2014. https://doi.org/10.1109/TSG.2014.2318836 DOI: https://doi.org/10.1109/TSG.2014.2318836
A. G. Tsikalakis and N. D. Hatziargyriou, "Centralized control for optimizing microgrids operation," IEEE Trans. Energy Conv., vol. 23, no. 1, pp. 241-248, March 2008. https://doi.org/10.1109/TEC.2007.914686 DOI: https://doi.org/10.1109/TEC.2007.914686
N. Anglani, G. Oriti, and M. Colombini, “Optimized energy management system to reduce fuel consumption in remote military microgrids,” IEEE Trans. Ind. App., vol. 53, no. 6, pp. 5777-5785, 2017. https://doi.org/10.1109/TIA.2017.2734045 DOI: https://doi.org/10.1109/TIA.2017.2734045
B. Heymann, J. F. Bonnans, P. Martinon, F. J. Silva, and F. L. G. Jiménez-estévez, “Continuous optimal control approaches to microgrid energy management,” Energy Syst., vol. 9, pp. 59-77, 2017. https://doi.org/10.1007/s12667-016-0228-2 DOI: https://doi.org/10.1007/s12667-016-0228-2
J. B. Almada, R. P. S. Leão, R. F. Sampaio, and G. C. Barroso, “A centralized and heuristic approach for energy management of an AC microgrid,” Renew. Sustain. Energy Rev., vol. 60, pp. 1396-1404, 2016. https://doi.org/10.1016/j.rser.2016.03.002 DOI: https://doi.org/10.1016/j.rser.2016.03.002
K. Hassan Youssef, “Optimal management of unbalanced smart microgrids for scheduled and unscheduled multiple transitions between grid-connected and islanded modes,” Electr. Power Syst. Res., vol. 141, pp. 104-113, 2016. https://doi.org/10.1016/j.epsr.2016.07.015 DOI: https://doi.org/10.1016/j.epsr.2016.07.015
A. Choudar, D. Boukhetala, S. Barkat, and J. Brucker, “A local energy management of a hybrid PV -storage based distributed generation for microgrids,” Energy Convers. Manag., vol. 90, pp. 21-33, 2015. https://doi.org/10.1016/j.enconman.2014.10.067 DOI: https://doi.org/10.1016/j.enconman.2014.10.067
P. P. Vergara, J. C. López, L. C. P. Silva, and M. J. Rider, “Security-constrained optimal energy management system for three-phase residential microgrids,” Electr. Power Syst. Res., vol. 146, pp. 371-382, 2017. https://doi.org/10.1016/j.epsr.2017.02.012 DOI: https://doi.org/10.1016/j.epsr.2017.02.012
H. Kanchev, D. Lu, F. Colas, V. Lazarov, B. Francois, and S. Member, “Energy management and operational planning of a microgrid with a PV-based active generator for smart grid applications,” IEEE Trans. Ind. Electronics, vol. 58, no. 10, pp. 4583-4592, 2011. DOI: https://doi.org/10.1109/TIE.2011.2119451
M. Sechilariu, B. Wang, and F. Locment, “Building-integrated microgrid: Advanced local energy management for forthcoming smart power grid communication,” Energy Build., vol. 59, pp. 236-243, 2013. https://doi.org/10.1016/j.enbuild.2012.12.039 DOI: https://doi.org/10.1016/j.enbuild.2012.12.039
A. Askarzadeh, “A memory-based genetic algorithm for optimization of power generation in a microgrid,” IEEE Trans. Sust. Energy, vol. 9, no. 3, pp. 1081-1089, 2017. https://doi.org/10.1109/TSTE.2017.2765483 DOI: https://doi.org/10.1109/TSTE.2017.2765483
N. Tiwari and L. Srivastava, "Generation scheduling and micro-grid energy management using differential evolution algorithm," 2016 Int. Conf. Circuit, Power Comp. Tech. (ICCPCT), Nagercoil, India, 2016, pp. 1-7. https://doi.org/10.1109/ICCPCT.2016.7530218 DOI: https://doi.org/10.1109/ICCPCT.2016.7530218
T. Niknam, F. Golestaneh, and A. Malekpour, “Probabilistic energy and operation management of a microgrid containing wind / photovoltaic / fuel cell generation and energy storage devices based on point estimate method and self-adaptive gravitational search algorithm,” Energy, vol. 43, no. 1, pp. 427-437, 2012. https://doi.org/10.1016/j.energy.2012.03.064 DOI: https://doi.org/10.1016/j.energy.2012.03.064
M. Motevasel and T. Niknam, “Multi-objective energy management of CHP (combined heat and power)-based micro-grid,” Energy, vol. 51, pp. 123-136, 2013. https://doi.org/10.1016/j.energy.2012.11.035 DOI: https://doi.org/10.1016/j.energy.2012.11.035
M. Motevasel and A. R. Seifi, “Expert energy management of a micro-grid considering wind energy uncertainty,” Energy Convers. Manag., vol. 83, pp. 58-72, 2014. https://doi.org/10.1016/j.enconman.2014.03.022 DOI: https://doi.org/10.1016/j.enconman.2014.03.022
G. Kyriakarakos, A. I. Dounis, K. G. Arvanitis, and G. Papadakis, “A fuzzy logic energy management system for polygeneration microgrids,” Renew. Energy, vol. 41, pp. 315-327, 2012. https://doi.org/10.1016/j.renene.2011.11.019 DOI: https://doi.org/10.1016/j.renene.2011.11.019
D. Tenfen and E. C. Finardi, “A mixed integer linear programming model for the energy management problem of microgrids,” Electr. Power Syst. Res., vol. 122, pp. 19-28, 2015. https://doi.org/10.1016/j.epsr.2014.12.019 DOI: https://doi.org/10.1016/j.epsr.2014.12.019
M. Abedini, M. H. Moradi, and S. M. Hosseinian, “Optimal management of microgrids including renewable energy scources using GPSO-GM algorithm,” Renew. Energy, vol. 90, pp. 430-439, 2016. https://doi.org/10.1016/j.renene.2016.01.014 DOI: https://doi.org/10.1016/j.renene.2016.01.014
G. K. Venayagamoorthy and R. Sharma, “Dynamic energy management system for a smart microgrid,” IEEE Trans. Neural Networks Learning Syst., vol. 27, no. 8, pp. 1-14, 2016. http://dx.doi.org/10.1109/TNNLS.2016.2514358 DOI: https://doi.org/10.1109/TNNLS.2016.2514358
E. De Santis, A. Rizzi, and A. Sadeghian, “Hierarchical genetic optimization of a fuzzy logic system for energy flows management in microgrids,” Appl. Soft Comput. J., vol. 60, pp. 135-149, 2017. https://doi.org/10.1016/j.asoc.2017.05.059 DOI: https://doi.org/10.1016/j.asoc.2017.05.059
C. S. Karavas, G. Kyriakarakos, K. G. Arvanitis, and G. Papadakis, “A multi-agent decentralized energy management system based on distributed intelligence for the design and control of autonomous polygeneration microgrids,” Energy Convers. Manag., vol. 103, pp. 166-179, 2015. https://doi.org/10.1016/j.enconman.2015.06.021 DOI: https://doi.org/10.1016/j.enconman.2015.06.021
H. S. V. S. K. Nunna, A. M. Saklani, A. Sesetti, S. Battula, S. Doolla, and D. Srinivasan, “Multi-agent based demand response management system for combined operation of smart microgrids,” Sustain. Energy, Grids Networks, vol. 6, pp. 25-34, 2016. https://doi.org/10.1016/j.segan.2016.01.002 DOI: https://doi.org/10.1016/j.segan.2016.01.002
L. Ma, N. Liu, J. Zhang, and W. Tushar, “Energy management for joint operation of CHP and PV prosumers inside a grid-connected microgrid: A game theoretic approach,” IEEE Tran. Ind. Informatics, vol. 12, no. 5, 2016. https://doi.org/10.1109/TII.2016.2578184 DOI: https://doi.org/10.1109/TII.2016.2578184
G. E. Asimakopoulou, A. L. Dimeas, and N. D. Hatziargyriou, "Leader-follower strategies for energy management of multi-microgrids," IEEE Trans. Smart Grid, vol. 4, no. 4, pp. 1909-1916, Dec. 2013. https://doi.org/10.1109/TSG.2013.2256941 DOI: https://doi.org/10.1109/TSG.2013.2256941
H. S. V. S. Kumar Nunna and S. Doolla, "Energy management in microgrids using demand response and distributed storage – A multiagent approach," IEEE Trans. Power Delivery, vol. 28, no. 2, pp. 939-947, April 2013. https://doi.org/10.1109/TPWRD.2013.2239665 DOI: https://doi.org/10.1109/TPWRD.2013.2239665
L. Kumar, S. R. K, A. Verma, B. K. Panigrahi, and R. Kumar, “An operation window constrained strategic energy management (OWCSEM ) of micro grid with electric vehicle and distributed resources,” IET Gen. Trans. Dist., vol. 11, no. 3, 615-626. https://doi.org/10.1049/iet-gtd.2016.0654 DOI: https://doi.org/10.1049/iet-gtd.2016.0654
How to Cite
APA
ACM
ACS
ABNT
Chicago
Harvard
IEEE
MLA
Turabian
Vancouver
Download Citation
Recibido: 8 de agosto de 2022; Aceptado: 10 de mayo de 2023
Abstract
Context:
Microgrids have been gaining space and credibility in terms of research and real applications. Tech- nological maturity and new regulations have allowed these types of systems to position themselves as a real alternative to increase the coverage of the energy service and improve its quality. One of the biggest challenges of microgrids is the management of resources and their synchronization with conventional grids. In order to overcome the inconvenience of synchronizing and managing the components of a microgrid, research on management systems has been conducted, which usually consist of a set of modules and control strategies that manage the available resources. However, these studies have not reached unanimity on the best method to perform these tasks, which is why it is necessary to perform a systematic collection of information and clearly define the state of research in energy systems management for this type of network.
Method:
Based on the above, a systematic mapping was carried out in this article, wherein a significant number of papers that have contributed to this area were compiled. Taxonomies were generated based on the nature of the variables collected. These variables correspond to the data or information that enters and/or leaves the microgrid management system, such as meteorological variables, power, priority loads, intelligent loads, economic, operating states, and binary outputs.
Conclusions
: It was observed that, despite the advances in studying different techniques and strategies microgird control and management, other factors that may affect performance have not been covered in a relevant way, such as the nature of variables and microgrid topology, among others.
Keywords:
microgrid, management system, input and output variables.Resumen
Contexto:
Las microrredes eléctricas han venido ganando espacio y credibilidad a nivel de investigación y aplicaciones reales. La madurez tecnológica y las nuevas regulaciones han permitido que este tipo de sistemas se posicionen como una alternativa real para aumentar la cobertura del servicio de energía y mejorar su calidad. Uno de los mayores retos de las microrredes es la gestión de los recursos y su sincronización con la red convencional. Con el fin de superar el inconveniente de sincronizar y gestionar los componentes de la microrred, se ha investigado sobre sistemas de gestión, los cuales normalmente consisten en un conjunto de módulos y estrategias de control que administran los recursos disponibles. Sin embargo, estas investigaciones no han llegado a una unanimidad sobre el mejor método para realizar estas tareas, por lo cual se hace necesario realizar una recopilación sistemática de información y definir claramente el estado de la investigación en gestión de sistemas de energía para este tipo de redes.
Método:
Con base en lo anterior, en este artículo se realizó un mapeo sistemático, donde se recopiló un importante número de artículos que han aportado a este campo. Se generaron taxonomías basadas en la naturaleza de las variables que se recopilaron. Dichas variables corresponden a los datos o información que entran y/o salen del sistema de gestión de la microrred, tales como variables meteorológicas, potencia, cargas prioritarias, cargas inteligentes, económicas, estados de operación y salidas binarias.
Conclusiones
: Se observa que, a pesar de los avances en el estudio de las diferentes técnicas y estrategias de control y gestión de microrredes, no se han cubierto de forma relevante otros factores que pueden afectar al rendimiento, como la naturaleza de las variables y la topología de la microrred, entre otros.
Palabras clave:
microrred, sistema de gestión, variables de entrada y salida.Introduction
Since the emergence of microgrids, the incidence of renewable energies in the energy landscape has increased 1. A microgrid consists of the interconnection of loads of different nature, communication systems, metering, control, and distributed energy sources. This system can operate in standalone mode and connect with other microgrids or the main power grid 1), (2. A microgrid has the following sections: a physical plant where the distributed energy components are located (generation and storage elements, load groups, flexible loads, smart loads, and protection and connection elements); a control section, which governs the components of the physical plant and may be centralized (the management system controls the microgrid as a single entity) or decentralized (the control of each component is independent); and a management section, where the flow of energy depends on data from inside and outside the microgrid, according to specific objectives 3 (Fig. 1). Microgrids have become more relevant due to changes in regulatory aspects 4 and the cost reduction of different components 5. These characteristics have also led to increased research aimed at solving various problems associated with these systems 6, particularly focusing on the development of a good energy management system (EMS) 7).
Figure 1: Microgrid sections
The first publications on microgrids date back to the early 2000s 8, which could be counted by the dozen. Nowadays, publications amount to approximately 1.500 articles per year 9. A wide variety of factors affect microgrids, such as generation, energy demand, storage status, and the cost of energy, without omitting any regulations that apply. These factors must be synchronized and managed in order to ensure the most efficient operation 10), (11. To this effect, a microgrid must have a suitable local generation system that allows it to operate in any scenario (desired and undesired) 12.
Different approaches have studied the problems associated with energy resource management 13, 14. A variety of research works focus on microgrid control methods and configurations 15, management system functionalities 16, architectures and the components to be controlled 17, and input and output variables. Different objectives and factors influence microgrid energy flow 18. However, these works have not reached unanimity on these aspects. Therefore, a systematic compilation of these works is necessary, in addition to a clear definition of the state of research on EMS for this type of grid.
This paper presents a descriptive review of the factors involved in the management system of a microgrid, such as input and output variables, topology configurations, and the control method and strategy. This review seeks to be a reference tool that will be available for all those interested in working on microgrid energy management to find a compendium of the main components in this type of system.
The structure of this paper is as follows: Section 2 presents the concept of a microgrid management system; Section 3 explains the variables involved in a microgrid management system; Section 4 presents them; and Section 5 provides some conclusions based on the collected information.
Microgrid management systems
In IEC 61970, the International Electrotechnical Commission defines a management system in electrical environments as ”a computer program comprising a software platform that provides basic support services and a set of applications providing the functionality necessary for the efficient operation of electrical generation and transmission facilities to ensure adequate security of power supply at minimum cost" 19. A microgrid management system generally consists of modules such as energy resource distribution and load forecasting systems, human-machine interfaces, and supervisory control and data acquisition (SCADA) systems, which ensure the efficient implementation of decision-making strategies by sending orders to generation, storage, and load unit components 20. The management system performs various functions, such as monitoring, analyzing, and forecasting power generation from distributed energy resources, load consumption, energy market prices, auxiliary markets, and meteorological factors. These functions help the management system improve the microgrid’s performance while compensating for its limitations 21.
Management systems implement one of two control strategies: centralized and decentralized. In the centralized one, a central controller accumulates information from the energy resources distribution and load forecasting modules, such as meteorological and energy data and user consumption patterns, among others. These data enter the SCADA module, which determines the energy programming of the microgrid and sends orders to the local controller, which is in charge of executing them in the different components of the microgrid 15. In contrast, in the decentralized control strategy, the central microgrid controller sends and receives all information from the local controllers of the microgrid components in real time. Each local controller proposes a current and future order to the central controller of the microgrid, and the central controller determines the most beneficial scheduling and delivers it to the local controllers. The latter may disagree with the current operation and may continue negotiating until global and local objectives are achieved 22.
With the integration of renewable energy resources, storage systems, electric vehicles, and demand response, the objectives of microgrid management systems have diversified, e.g., scheduling distributed energy resources and loads, minimizing system losses and outages, controlling the intermittency and volatility of renewable energy resources, and achieving economical, sustainable, and reliable operation for the microgrid 16.
Variables involved in a microgrid management system
Unlike traditional power systems, in a microgrid, it is possible to evaluate multiple variables thanks to the installation of smart meters and a better knowledge of the system’s operation. These variables can be inputs or outputs of the management system.
Management system inputs
Different works focused on implementing EMS 23)-(26 show the diverse use of input variables and different alternatives in decision-making.
The input variables can be divided into three categories:
-
External variables
-
Internal variables
-
Economic variables
External variables
The external inputs of the microgrid are related to the factors that influence energy generation and are usually of a meteorological nature. They change with respect to the type of renewable energy used.
For photovoltaic energy, the relevant variables are
-
Solar irradiance
-
Environment temperature
-
Effective hours of sunlight
For wind energy, there is
-
Wind speed
-
Air density
-
Rotor area or surface area in contact with the air
The external variables can be subdivided into two new categories:
-
Meteorological
-
Generation system power
Meteorological
In 28)-(32, the authors use solar irradiance, temperature, and wind speed as external variables of a meteorological nature. Uncertainty prediction methods establish the variable values to obtain an approximation of the microgrid’s energy and ability to supply the demand of its loads. Although these references share these common aspects, they implement different architectures, generation systems, strategies, and control methods 16. Although there is a difference in generation sources, the treatment of information and the obtention methods are similar. A general review of this subject is presented in Table I.
Table I: External meteorological variables
The most relevant meteorological variables are solar irradiance, temperature, and wind speed. Fig. 2 shows the use percentage of these variables. Irradiance is the most frequently mentioned due to the technological advances for measuring it and the availability of official information in some countries. Methods based on artificial intelligence have led the field by predicting behavior and due to their ability to use large volumes of data to estimate the generation potential.
Figure 2: a) Relevant meteorological variables, b) management techniques relevant to meteorological variables
Generation system power
In several works 18),(40)-(46, the variables used by the management system involve the output power of the generation systems in order to determine the maximum power that can be supplied via historic data or by directly measuring the output power. Hence, they only consider the result of the microgrid’s energy generation (Table II).
Table II: External variables associated with generation system power
Figure 3: a) Relevant power generation system variables, b) relevant management techniques regarding the power variables of generation systems
Internal variables
The information handled by the internal variables is electrical. Within this category the following groups can be mentioned:
-
The state of charge and capacity of the storage system
-
The state of charge of an electric vehicle
-
The power output of the generation or prediction
-
The frequency of the generated power
-
Load demand, smart loads, and flexible loads
There are two subcategories:
-
Priority loads
-
Controllable loads
Priority loads
Within this category, 27), (28), (42), (43), (48), and 56 control variables that are inherent to the mi- crogrid. There are loads with a degree of priority, as the network is operated via a management system with a hierarchy of consumption. This system prioritizes the supply of loads with a higher value regard- ing decision-making (Table III).
Table III: Internal variables for priority loads
The most relevant variables used in this category are shown in Fig. 4.
Figure 4: a) Relevant variables of priority loads, b) relevant management techniques for priority load variables
Controllable loads
In 10), (48), (50)-(52, the implementation of controllable or intelligent loads that help to prevent the network from overloading or destabilizing also facilitates communication with the management system. This configuration allows the EMS to adjust the power supplied or the actions to be taken from a predetermined range of parameters. Thus, the loads can operate partially, preserving their primary functions (Table IV).
Table IV: Internal variables for controllable loads
Economic variables
The economic variables of the microgrid are mainly related to its operation and maintenance costs, e.g.,
-
The cost of operation (maintenance and start-up)
-
Energy purchase price
-
Energy sale Price
This financial information is usually obtained through energy sales exchanges 61 and has two sub-categories:
-
Energy value
-
Cost of generation
Figure 5: a) Relevant variables of controllable loads, b) relevant management techniques for controllable load variables
Energy value
As per 29),(30),(33),(46, and (?), this category has associated operating costs and values, with particular emphasis on the energy price. The energy value generated can be similar to the energy price for the time during which the microgrid operates autonomously. This results in a saving that justifies the resources spent, contributing to the viability of the microgrid’s implementation (Table V).
Table V: Internal variables for controllable loads
The most relevant variables used in this category are shown in Fig. 6.
Figure 6: a) Economic variables regarding energy value, b) relevant management techniques regarding energy value
Cost of generation
In 26), (30), (42, and 43, factors such as the fuel or generation cost, the operating costs, and the maintenance costs of the microgrid take on greater relevance. The price of energy supplied by the conventional power grid is not considered by these authors, as the microgrid operates in islanded mode (Table VI).
Table VI: Economic variables regarding the cost of generation
The most relevant variables used in this category are shown in Fig. 7.
Figure 7: a) Economic variables regarding the cost of generation, b) relevant management techniques for economic variables regarding the cost of generation
Management system outputs
A management system’s output is the instruction or orders that the system executes to fulfill an objective. These instructions influence the routing of the energy flow and the operation or shutdown of components.
The outputs of the management system can be divided into two categories:
A. Preset operating status
B. Binary decisions
A. Preset operating status
28), (41)-(43, and 48 propose, as outputs of their management systems, pre-defined scenarios that are activated when the microgrid parameters reach previously defined values. These scenarios focus on directing the energy flow to meet a specific objective, maintaining the microgrid in islanded mode, feeding the storage components, or reducing the demand of the loads, among others (Table VII)
Table VII: Preset operating status
The most relevant variables used in this category are shown in Fig. 8.
Figure 8: a) Output variables for the preset operating status, b) relevant management techniques regarding the output variables for the preset operating status
B. Binary decisions
In 26), (27), (30, and 43, the management systems’ outputs correspond to the connection or disconnection of microgrid elements, as well as to the flow of energy (Table VIII).
Table VIII: Binary decisions
The most relevant variables used in this category are shown in Fig. 9.
Figure 9: a) Binary output variables, b) relevant management techniques for binary output variables
Analysis
This systematic review shows the importance of microgrid components and their influence on the data to be considered by the management system. The importance is primarily evident in the type of generation of the microgrid, where solar irradiance is the most relevant input of the external category. The meteorological subsection, which pertains to more than 40 % of the papers studied, shows a more effective implementation in microgrids that have photovoltaic generation sources. The same trend is visible in the category of external variables related to generation power, where photovoltaic power prediction is the most recurrent, with 31 % of the papers studied. This also applies to the category of external variables, with wind speed being the second most relevant meteorological variable (31 %). Similarly, for generation power, wind energy prediction variable is present in 19 % of the papers studied, which contrasts with the implementation of microgrids that use fossil fuels as generation sources, (5 %). This can be better seen in Fig. 10a. These results show a clear trend regarding the implementation of renewable energies in microgrids.
Figure 10: a) Microgrid generation in the reviewed papers, b) microgrid control strategies in the reviewed papers
Although some microgrid components influence the data used by the EMS, there is no significant impact. The methods for collecting and processing data are so varied that they do not reflect a significant statistical difference in the inputs and outputs of the management system. On the other hand, a more effective implementation of centralized control strategies is noticeable, which is present in 83 % of the papers studied (Fig. 10b). A meticulous analysis of the papers’ year of publication shows a trend towards decentralized control strategies. This may be due to the fact that these strategies are more flexible and ensure a better fulfillment of the objectives, although the computational effort and resources required are more significant 16. The control techniques implemented by the management systems of the studied papers are so varied that they do not represent a significant statistical difference, as seen in Fig. 11a.
The inputs follow the needs of the management system modules and the specific components of the microgrid. This need is evident since more than 90 % of the papers studied implement some type of storage system, mainly batteries, whose state of charge and storage capacity are used for monitoring. The other variables in this category depend more on the type of loads that the microgrid must supply. The economic variables depend primarily on the microgrid architecture, as they are directly related to the connection to the main grid or other microgrids. If the microgrid is islanded, the economic variables focus on the costs of operation and the fuel prices, given that diesel generators or similar resources are used to guarantee a constant flow of energy. The connection with the primary grid entails a focus on the energy sales price and the operating costs. This type of architecture is the most used in the analyzed papers (78 %) (Fig. 11b).
Figure 11: a) Microgrid control strategies in the reviewed papers, b) microgrid architecture in the reviewed papers
The output variables do not show any direct relationship with the EMS modules studied when compared to the input variables. The outputs depend more on how the author wants decision-making to take place, e.g., by having binary decisions involving the connection or disconnection of microgrid components. Decisions on the generation and autonomy of the microgrid are the most implemented when compared to those aimed at managing the demand.
Conclusions
This review article started with a brief description of the management systems applied to microgrids, in order to determine the functions they should perform. With these definitions, the data to be collected and its associated variables were determined, which would be the input for a correct EMS performance. With these variables as a basis, an analysis of their properties was conducted. The variables that shared properties were grouped into categories while considering the functions for which they provide information. In light of the above, this article proposes a taxonomy for the input and output variables of a microgrid management system.
Progress has been made in microgrid management using different control and management techniques and strategies, evaluating their pros and cons. However, it is relevant to consider other factors that may affect performance, such as the nature of the variables and the topology of the microgrid, among others.
It is important to know the variables that serve as the inputs and outputs of management systems in order to ensure a good microgrid performance. In this vein, this paper helps those interested in delving into the implementation of these systems. The proposed taxonomy could serve as a guide to perform the conceptual and basic engineering of projects in this sector.
Given that most of the papers studied used simulation environments, it is also necessary to test management systems in real-world scenarios to evaluate their effects and performance
References
License
Copyright (c) 2023 Carlos Santiago Vidal-Martinez, Maximiliano Bueno-López, Juan Fernando Flórez-Marulanda, Álvaro Rene Restrepo

This work is licensed under a Creative Commons Attribution-NonCommercial-ShareAlike 4.0 International License.
From the edition of the V23N3 of year 2018 forward, the Creative Commons License "Attribution-Non-Commercial - No Derivative Works " is changed to the following:
Attribution - Non-Commercial - Share the same: this license allows others to distribute, remix, retouch, and create from your work in a non-commercial way, as long as they give you credit and license their new creations under the same conditions.