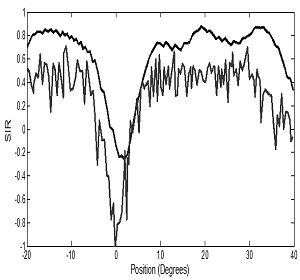
DOI:
https://doi.org/10.14483/udistrital.jour.reving.2016.2.a07Published:
2016-05-26Issue:
Vol. 21 No. 2 (2016): May - AugustSection:
Special Section: Best Extended Articles - WEA 2015Adaptive Beamforming for Moving Targets Using Genetic Algorithms
Formación de Haz Adaptativo para Objetos Móviles usando Algoritmos Genéticos
Keywords:
Smart Antenna, beamforming, moving targets, CDMA, genetic algorithms (en).Keywords:
Antena Inteligente, beamforming, CDMA, algoritmos genéticos (es).Downloads
References
A. Paulraj, R. Roy, and T. Kailath, “A subspace rotation approach to signal parameter estimation” Proceedings of the IEEE, vol. 74, no. 7, pp. 1044 – 1046, July 1986
Y. R. Ferreira, ”Métodos de Estimação de Ângulos DOA”, Tese de Mestrado, Universidade Federal de Goiás, Goiânia, 2005.
R. O. Schmidt, Multiple emitter location and signal parameter estimation. In: Proc. RADC Spectral Estimation Workshop. [S.l.: s.n.], 1979. p. 243.258.A. J.
A. J. Barabell, “Improving the resolution performance of eigenstruture-based direcion - finding algorihtms. In: Proc. of the IEEE Int'l. Conf. on Acoustic, Speech, and Signal Processing-83. [S.l.: s.n.], pp. 336 – 339, 1983.
P. Stoica and K. Sharman, “Novel eigenanalysis method for direction estimation” In: PROCEEDINGS, I. (Ed.). IEE Proceedings. [S.l.: s.n.], v. 137, n. 1,1990.
H. V. Leão e Silva “Redução da complexidade computacional do método de estimação de ângulos de incidência através da diferença entre os valores singulares da matriz de covariância espacial” Tese de Mestrado, Universidade Federal de Goiás, Goiânia, 2009.
S. B. Puttini, “Emprego de Antenas Adaptativas para Estimação de Dados em Ambiente CDMA”, Tese de Mestrado, Universida-de Federal de Brasília, Brasília, Março 2006.
V. V. de Faria, “Antenas Adaptativas para Sistemas de Comunicações sem Fio” Tese de Mestrado, Instituto Nacional de teleco-municações, Santa Rita do Sapucaí, 2003.
W. L. Stutzman e G. A. Thiele, “Antenna Theory and Desing”, John Wiley & Sons, Nova Iorque EUA, 1981.
C. Arévalo e J. Rojas, “Material Didáctico para el Estudio y Simulación de CDMA: Aplicación a Comunicaciones Móviles”, Escue-la Politécnica Nacional, Quito, 2012.
A. E. Zooghby, “Smart Antenna Engineering”, Artech House, Norwood EUA, 2005.
S. Haykin, “Modern Filters”, Macmillan Publ Company, Nova Iorque EUA,1989.
G. Blanchet & M. Charbit, “Digital Signal and Image Processing Using MATLAB”, ISTE Ltd, Londres UK, 2006.
J. C. Liberti e T.S. Rappaport, “Smart Antennas for Wireless Communications: IS-95 and Third Generation CDMA Applications”, Prentice Hall, Nova Jersey EUA, 1999.
M. Vitale, G. Vesetini, N.N. Ahmad & L. Hanzo,” Genetic Algorithm Assisted Adaptive Beamforming”, Vehicular Technology Conference, vol. 1, pp. 601 – 605, 2002.
A. S. Rocha, “Otimização Multiobjetivo e Multirestrição da Cobertura de Redes de Frequência Única”, Tese de Doutorado, Universidade Federal de Brasília, Brasília, Agosto 2013.
D. Burgos, R. Lemos, J. Kunzler & H. Silva, “Adaptive Beamforming for Moving Traget Using Genetics Algorithms and a CDMA Reference Signal”, IEEE COLCOM, pp. 1-5, 2015.
R.L. Haupt, “Phase-only adaptive nulling with a GA”, IEEE Transactions on Antennas and Propagation., vol. 45, pp.1009- 1015, 1997.
D. Burgos, R. Lemos, J. Kunzler & H. Silva, “Adaptive Beamforming for Moving Traget Using Genetics Algorithms”, IEEE WEA, pp. 1-5, 2015.
J. Hung & A. Chang, “Combining genetic algorithm and iterative MUSIC searching DOA estimation for the CDMA system”, Expert Systems with Applications, pp. 1895-1902, 2011.
How to Cite
APA
ACM
ACS
ABNT
Chicago
Harvard
IEEE
MLA
Turabian
Vancouver
Download Citation
Visitas
Dimensions
PlumX
Downloads
DOI: http://dx.doi.org/10.14483/udistrital.jour.reving.2016.2.a07
Adaptive Beamforming for Moving Targets Using Genetic Algorithms
Formación de Haz Adaptativo para Objetos Móviles usando Algoritmos Genéticos
Diego Burgos
Universidade Federal de Goiás, d.burgos88@hotmail.com
Rodrigo Lemos
Universidade Federal de Goiás, lemos@ufg.br
Hugo Silva
Instituto Federal de Educ. Ciência e Tecnol. de Goiás hugovinicius@ifg.edu.br
Jonas Kunzler
Universidade Federal de Goiás, k.jonsaugusto@ufg.br
Edna Flôres
UniversidadeFederal de Uberlândia. edna@ufu.br
Received: 24-11-2015. Modified: 14-03-2016. Accepted: 06-04-2016.
Abstract
Context: This works investigates the use of Genetic Algorithm (GA) for beamforming on a Code Division Multiple Access (CDMA) environment under different Signal-to-Noise Ratios (SNR), assuming a reference signal is known.
Method: The GA is a method inspired in evolutionary principles to optimize an objective function by choosing the best candidates of a population. The population is randomly generated to ensure high diversity and get a global optimization. On the other hand, the Least Means squares (LMS) algorithm is an adaptive algorithm with guaranteed convergence as long as a reference signal is known.
Results: The GA converged faster than the LMS in all tested scenarios. Besides, GA achieved best results in pointing the beam for uncorrelated static sources. Additionally, proper tuning of GA parameters allowed fast convergence and improved tracking of moving targets.
Conclusions: The simulation results confirm that the GA is able to obtain a convergent and accurate tool for beamforming and tracking of moving targets, given a reference signal. Hence, GA turns to be promising in replacing LMS on Smart Antenna Systems for increasing channel capacity.
Keywords: Smart Antenna, beamforming, moving targets, CDMA, genetic algorithms.
Acknowledgements: Organization of American States, Universidade Federal de Goiás.
Resumen
Contexto: En este trabajo se investiga el uso de un Algoritmo Genético (GA) para la conformación del haz de un arreglo de antenas en ambientes de Acceso Múltiple por División de Código (CDMA) bajo diferentes relaciones Se˜nal a Ruido, asumiendo que la se˜nal de referencia es conocida.
Método: El Algoritmo Genético es un método inspirado en principios evolutivos, usado para optimizar una función objetivo seleccionando los mejores candidatos de una población. La población es generada aleatoriamente para asegurar alta diversidad y conseguir una optimación global. Por otro lado, el algoritmo LMS es un algoritmo que garantiza la convergencia siempre y cuando la se˜nal de referencia sea conocida.
Resultados: El GA converge más rápidamente en que el algoritmo LMS en todos los escenarios probados. Además, el GA consiguió mejores resultados apuntando el haz para fuentes estáticas descorrelacionadas. Adicionalmente, una apropiada selección de los parámetros del GA permite una mayor velocidad de convergencia y un mejorado rastreamiento de fuentes en movimiento.
Conclusiones: Los resultados de las simulaciones confirman que el GA es una herramienta capaz de obtener una convergencia y precisión en la conformación del haz y el rastreamiento de fuentes en movimiento dada una se˜nal de referencia. Por lo tanto, el GA resulta prometedor para sustituir el algoritmo LMS en sistemas de antenas inteligentes y aumentar la capacidad del canal.
Palabras clave: Antena Inteligente, beamforming, CDMA, algoritmos genéticos.
Agradecimientos: Organización de los Estados Americanos, Universidad Federal de Goiás.
1. Introduction
Smart Antennas are antenna arrays (sensors) scattered on a specific geometry that present as output a combi-nation of the signals induced in its many elements. Actually, those sensors are omnidirectional antennas that allow changing their group radiation diagram through adaptive methods, pointing the main lobe to the main target, while positioning zeros towards the interfering sources. This way, using those arrays allows increasing the Signal-to-Interference-plus-Noise Ratio (SINR) and, consequently, the channel capacity to support the increasing demand for multimedia services in mobile cell phone networks.
Adaptive filters using least mean squares (LMS) [7] [8] have been successful on the beamforming task under several noise and multipath interference scenarios. They adjust a spatial filter on the array output in order to minimize the error between a reference signal and the filtered signal. Consequently, the main beam turns to be iteratively directed towards the target signal. This strategy is particularly interesting for tracking moving targets, due to its adaptability. However, under more severe conditions of signal reception, LMS fails to converge to suit-able solutions and points the main lobe to wrong directions.
Genetic Algorithms have proven to be useful in global optimization tasks, like base-station planning in coverage maximization [16] and Direction of Arrival estimation [15]. So, in this paper we propose using Genetic Algorithms instead of LMS to direct the main beam of a linear antenna array towards a mobile source, synchronizing a space filter according to a reference signal. This reference is built under the assumption that DS-CDMA spreading code is known, as stated by Puttini [7].
We remark that this is an extended version of a short paper recently published in the Workshop on Engineering Applications -WEA 2015- that was held in Bogotá, September 2015 [19].
2. Signal Model
According to [17], a Uniform Linear Array (ULA), composed of M half-wavelength spaced elements, suffers the incidence of K plane waves on directions θk related to the signals xk(t), k = 1,…,K [7]. The induced voltages on the array elements, sampled at times t = 1,…,N form a snapshot matrix, described as:
where A=[a(φ1)a(φ2) … a(φk)] is an M x K Vandermonde matrix, a(φk)=[1e-jφk … e-j(M-1)φk ]T are the M x 1 steering vectors, φk = 2πd.sen(θk/λ) are the electric angles corresponding to θk and nM(t) represents the additive white Gaussian noise samples on the mth element of the array. This can be written in matrix notation as:
The array output y =[y(1) … y(N)] is given by a linear combination of the induced voltages on the sensors, weighted by a vector w=[w1 … wM]T of a spatial filter coefficients:
where zm = [zm(1) … zm(N)] is the time sampled vector of the voltages on the mth sensor.
Considering a direct sequence code division multiple access system (DS-CDMA), signal xk=[xk(1)… xk(N)] from the kth source results from spreading the spectrum of the information bits bk ∈;{-1,1} associated to this source:
where ck ∈;{-1,1} is a spreading sequence containing a pseudo-noise code, and ⦿ stands for elementwise product. Considering we know the spreading sequence ck at the receiver and that it is synchronized with the target signal, we can recover bk by making:
since orthonormality between ci and ck is considered.
In practice, each xi has a different phase shift and orthonormality is not guaranteed such that interfering signals corrupt the binary message. So, spatial filtering can be used to mitigate interfering signals at angles of arrival that are different from that of the target signal and improve the Bit-Error-Rate (BER).
In this work we adopted a 64 chipsWalsh matrix [10] [11]. Then, considering fs samples per chip and B message bits during the observation interval, the amount of samples on a snapshot is N = 64 x fs x B.
3. Least Mean Squares Algorithm
The LMS algorithm iteratively updates the complex weights of a spatial filter on the array output by summing an estimate of the cross correlation between the received signal and the estimation error from the previous iteration [7], [17]. First, LMS initializes the weighting vector as ŵ0 = [1 1 … 1] such that the array is pointed towards θ = 0˚. Then, for each iteration i = 1, 2, …, it computes the filter output:
From the error between the output and a reference signal:
the algorithm updates the weighting vector:
Then, it returns to (6) and repeats the process until convergence is achieved. The positive factor, related to convergence speed and accuracy, shall be less than 1/tr(Rzz), where Rzz is the autocorrelation matrix of the voltages on the array sensors [7], [10], [17], [19].
4. Genetic Algorithm
Genetic Algorithms (GA) have proven to be useful in designing conventional, static beamforming networks, like in positioning radio-base stations for maximizing a coverage area [16]. It is also quite useful as an adaptive algorithm for smart antennas [15]. A GA relies on the Darwinian principle of natural selection and evolution [15] to optimize a solution to a given problem. In the beamforming context, a set of S potential solutions (individuals), ŵi, i = 1, … , S, is randomly generated and iteratively combined with the aid of genetic operations like crossover and mutation.
Generally, each weight wi,m of the antenna array is represented by a string of bits, such that half of the bits corresponds to the real part and the other half to the imaginary part of the array weight concerned [18]. Instead of doing so, we propose using complex weights wi,m = Re(wi,m)+jIm(wi,m), where both Re(wi,m) and Im(wi,m) are uniformly distributed in [-1,1]. Then, each individual is represented by an M x 1 complex weight vector ŵi, = [wi,1 …wi,M]T .
In this work, genetic operations must be adapted to work on complex chromosomes. This was readily done for crossover using the Radcliff blending method, such that:
where is fixed throughout the process. Also, mutation is accomplished by a straightforward procedure in which a chromosome is randomly selected and then replaced by a randomly chosen complex value. On the other hand, the mutation process adopted consists in randomly altering a chromosome's gene according to (10).
Tournament and elitism selection were employed to pick out the individuals that minimize the error between the corresponding array output and a reference signal, according to (6) and (7).
The proposed GA stops as soon as the error falls below a convergence tolerance, and the best individual is chosen as the optimal solution.
5. Results
In order to assess the beamforming performance, we started by calculating the pointing root mean square error (RMSE). A low RMSE is fundamental to the operation of smart antennas on environments with high background noise and many interfering sources. Initially the algorithms are tested with uncorrelated and correlated static sources to evaluate the performance and the convergence speed, and determine the applicability for moving sources. We employed a large initial population size aiming to better cover the space of solutions. However, the evolution process was performed only on a subset formed by the P2 best individuals of this population in order to speed up the algorithm. The tests were run on Windows 8, 8G RAM and Intel I7 processor. For the Genetic Algorithm, we used Elitism = 4.0; Crossing Probability = 95%; Mutation Probability = 1%; Radcliff Crossing with β = 0.3; initial populations with P1 = 1000 individuals; populations between generations with P2 = 200 individuals and G = 100 generations. Those parameters were kept the same during all the simulations due to great results in previous experiments.
5.1. Uncorrelated Static Sources
Figure 1 presents the RMSE for three static uncorrelated sources, being the target source located at 10˚ and the interfering sources at 40˚ and 60˚. A thousand experiments were performed for each SNR ranging from -35 up to 10 dB. Figure 1 shows that the LMS algorithm was capable of achieving null error rates above SNR = -9dB, while the GA performs visibly better, providing unitary error even at -12 dB. Consequently, both algorithms were able of precisely pointing to the target source position under relatively high noise conditions. However, GA revealed to be clearly more robust to the noise effects.
Figure 2 presents the histogram of the convergence times of both methods for static uncorrelated sources. The convergence times were analyzed based on the average times of convergence achieved by each algorithm for each SNR. After a thousand experiments for every SNR in the range -40dB to 10dB, the histogram evidences that GA was faster with average time of 0.251 seconds against 0.820 seconds for LMS. Also, the variance was smaller for GA than LMS.
An analysis of the computational complexity shows that, since both LMS and GA require complex matrix products and additions, the orders of their computational costs in the worst case are respectively:
Expressions (11) and (12) show that the computational cost of LMS grows cubically with the number of snapshots, while GA's cost grows cubically with the number of sensors. Since the number of sensors in the array is much less than the number of snapshots, GA performs less machine operations and converges faster than LMS as shown in Figure 2.
5.2. Correlated Static Sources
Figure 3 presents the performance for three completely correlated static sources, being the target located at 10˚ and the interfering sources at 40˚ and 60˚. Again, a thousand experiments were performed for each SNR ranging from 35 up to 10 dB. In this case, since all signals arrive with the same power, the array pointed the beam to the source with the lowest delay, Instead of achieving null error. The LMS algorithm produced unstable errors rates even at high SNR. On the other hand, GA performed better with error rates lower than those of LMS.
Figure 4 shows that, also for correlated sources, GA was faster with an average time of 0.248 seconds against 0.820 seconds for LMS. For every SNR in the range -40 dB to 10 dB, a thousand simulations were performed, resulting in a total of 51 thousand experiments.
The amount of machine operations of both algorithms is not affected by correlation of the sources, so that the GA remains faster than the LMS in this case, as shown in Figure 4.
5.3. Moving Target Sources
In order to assess the performance of LMS and GA in tracking moving targets, Figures 5 and 6 depict the displacement of a target source over time at the constant velocity of 0.2 degrees/second from -20˚ to 40˚, at SNR = 10 dB. Four static interference sources are simulated at -60˚, -30˚, 0˚ and 45˚. Doppler effect was taken into account on the moving source frequencies, but the frequency shift is virtually zero, so we can assume it is negligible.
LMS clearly pursued the target but, most of the time, its slow convergence rate prevented LMS from reaching the target. The resulting Mean Square Error (MSE) between the target track and the LMS estimated angles was 10.455 degrees. On the other hand, the faster convergence of GA allowed tracking the target closely, but sometimes pointed a little ahead of it, sometimes a little behind. Particularly, GA had more difficulties in pointing to the target when it crossed the direction of an interfering source. Even less stable, GA achieved a MSE of 5.077 degrees, almost the half of LMS fitting.
However, besides pointing the array on the right direction, beamforming is supposed to mitigate the interfering sources. Then, before giving the final word on the beamforming performance of GA, we measured the output Signal to Interference Ratio (SIR) of both algorithms during the tracking of the target source. Figure 7 shows that LMS was more accurate than GA in filtering out the target from the interfering sources. Therefore, GA was not so good in mitigating interfering sources as LMS.
Considering the relevance of such a system for real time communications, we investigated the beamforming efficiency for tracking a moving target among multiple moving interfering sources. Figures 8 and 9 shows the displacement of a target source and four interfering sources over time at a constant velocity of 0.2 degrees/second, the target source moves from -20˚ to 40˚, at SNR=10 dB. The interfering sources start displacements at -60˚, -30˚, 0˚ and 45˚ with random initial directions.
LMS pursued the target with a more stable behavior such that the Mean Square Error (MSE) between the real positions of the target source and the LMS estimated angles resulted in 11.3535 degrees. Furthermore, the low convergence speed prevented LMS from reaching the target. On the other hand, due to its faster convergence, GA was able to more closely track the target, resulting on a MSE of 2.3281 degrees, despite having a less stable behavior. Both algorithms had difficulties in pointing to the target when it crossed the path of an interfering source, but the LMS had more problems in these circumstances.
Another important measure of performance is the capacity of mitigating the interfering sources. Then, to assess the beamforming performance of the GA, we measured the output Signal to Interference Ratio (SIR) of both algorithms during the tracking of the target source [17].
Besides tracking the target, both algorithms were able to keep a positive SIR on the reception, except when the target crossed the path of an interfering source. However, Figure 10 shows that the low convergence speed of LMS algorithm also made it less efficient in mitigating the interfering sources. GA was more accurate in tracking the target out of interfering sources, despite presenting a less stable behavior.
6. Conclusion
This paper discussed the applications of Genetic Algorithms (GA) on beamforming of static uncorrelated and correlated sources, especially for moving targets on CDMA systems considering the spreading code of the signal of interest is known. LMS algorithm reached zero error rates at Signalto- Noise Ratios as low as -9 dB for static uncorrelated sources. For completely correlated sources LMS showed unstable performance, while GA showed better results in all cases of static sources achieving error rates lower than LMS. Additionally, in average, GA converged almost three times faster than LMS. This allowed GA to perform a close tracking while LMS makes fewer estimates, making tracking more difficult.
For future work, other algorithms will be tested and compared with the LMS and the GA algorithms in terms of accuracy, convergence speed and computing cost for different simulation environments. Also, it is considered implement the GA in real environments, in situations where the objective function change abruptly.
References
[1] A. Paulraj, R. Roy, and T. Kailath, "A subspace rotation approach to signal parameter estimation" Proceedings of the IEEE, vol. 74, no. 7, pp. 1044 - 1046, July 1986.
[2] Y. R. Ferreira, "Métodos de Estimaçaõ de Ángulos DOA", Tese de Mestrado, Universidade Federal de Goiás, Goânia, 2005.
[3] R. O. Schmidt, Multiple emitter location and signal parameter estimation. In: Proc. RADC Spectral Estimation Workshop. [S.l.: s.n.], 1979. p. 243.258.A. J.
[4] A. J. Barabell, "Improving the resolution performance of eigenstruture-based direcion - finding algorihtms. In: Proc. of the IEEE Int'l. Conf. on Acoustic, Speech, and Signal Processing-83. [S.l.: s.n.], pp. 336 - 339, 1983.
[5] P. Stoica and K. Sharman, "Novel eigenanalysis method for direction estimation" In: PROCEEDINGS, I. (Ed.). IEE Proceedings. [S.l.: s.n.], v. 137, n. 1,1990.
[6] H. V. Leão e Silva "Redução da complexidade computacional do método de estimação de ângulos de incidência através da diferença entre os valores singulares da matriz de covarância espacial" Tese de Mestrado, Universidade Federal de Goiás, Goânia, 2009.
[7] S. B. Puttini, "Emprego de Antenas Adaptativas para Estimação de Dados em Ambiente CDMA", Tese de Mestrado, Universidade Federal de Brasília, Brasília, Março 2006.
[8] V. V. de Faria, "Antenas Adaptativas para Sistemas de Comunicaç ões sem Fio" Tese de Mestrado, Instituto Nacionalde telecomunicações, Santa Rita do Sapucaí, 2003.
[9] W. L. Stutzman e G. A. Thiele, "Antenna Theory and Desing", John Wiley & Sons, Nova Iorque EUA, 1981.
[10] C. Arévalo e J. Rojas, "Material Didáctico para el Estudio y Simulación de CDMA: Aplicación a Comunicaciones Móviles", Escuela Politécnica Nacional, Quito, 2012.
[11] Zooghby, "Smart Antenna Engineering", Artech House, Norwood EUA, 2005.
[12] Haykin, "Modern Filters", Macmillan Publ Company, Nova Iorque EUA,1989.
[13] G. Blanchet & M. Charbit, "Digital Signal and Image Processing Using MATLAB", ISTE Ltd, Londres UK, 2006.
[14] J. C. Liberti e T.S. Rappaport, "Smart Antennas for Wireless Communications: IS-95 and Third Generation CDMA Applications", Prentice Hall, Nova Jersey EUA, 1999.
[15] M. Vitale, G. Vesetini, N.N. Ahmad & L. Hanzo," Genetic Algorithm Assisted Adaptive Beamforming", Vehicular Technology Conference, vol. 1, pp. 601 - 605, 2002.
[16] A. S. Rocha, "Otimizaçaõ Multiobjetivo e Multirestriçaõ da Cobertura de Redes de Frequência Única", Tese de Doutorado, Universidade Federal de Brasília, Brasília, Agosto 2013.
[17] D. Burgos, R. Lemos, J. Kunzler & H. Silva, "Adaptive Beamforming for Moving Traget Using Genetics Algorithms and a CDMA Reference Signal", IEEE COLCOM, pp. 1-5, 2015.
[18] R.L. Haupt, "Phase-only adaptive nulling with a GA", IEEE Transactions on Antennas and Propagation, vol. 45, pp.1009- 1015, 1997.
[19] D. Burgos, R. Lemos, J. Kunzler & H. Silva, "Adaptive Beamforming for Moving Traget Using Genetics Algorithms", IEEE WEA, pp. 1-5, 2015.
[20] J. Hung & A. Chang, "Combining genetic algorithm and iterative MUSIC searching DOA estimation for the CDMA system", Expert Systems with Applications, pp. 1895-1902, 2011.
License
From the edition of the V23N3 of year 2018 forward, the Creative Commons License "Attribution-Non-Commercial - No Derivative Works " is changed to the following:
Attribution - Non-Commercial - Share the same: this license allows others to distribute, remix, retouch, and create from your work in a non-commercial way, as long as they give you credit and license their new creations under the same conditions.