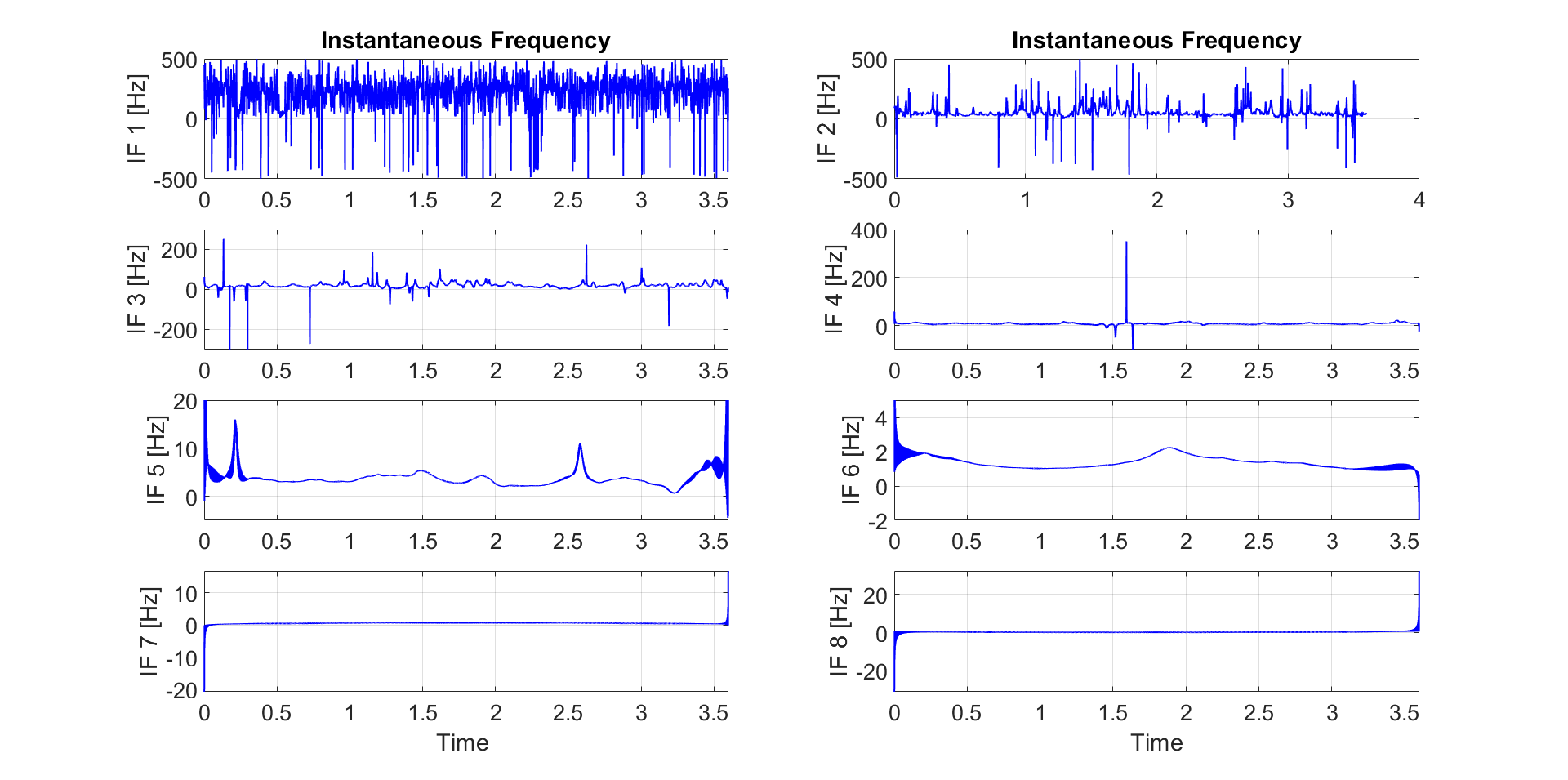
DOI:
https://doi.org/10.14483/23448393.21311Published:
2024-07-17Issue:
Vol. 29 No. 2 (2024): May-AugustSection:
Computational IntelligenceA Comparative Analysis between FFT, EMD, and EEMD for Epilepsy Detection
Análisis comparativo entre FFT, EMD y EEMD para la detección de epilepsia
Keywords:
electroencephalogram, Empirical Mode Decomposition, epilepsy, Instantaneous Frequency, Intrinsec Mode Functions, methodology, non-lineal, non-stationary, oscillation modes, seizures (en).Keywords:
convulsiones, Descomposicion Empírica de Modos, electroencefalograma, epilepsia, Frecuencias Instantáneas, Funciones de Modo Intrínseco, metodología, modos de oscilación, no-estacionaria, no-lineal (es).Downloads
Abstract (en)
Context: Epilepsy is a neurological disease that affects more than 50 million people worldwide, causing recurrent seizures, with a significant impact on patients' quality of life due to abnormally synchronized neuronal activity.
Method: This article discusses three methods used for signal analysis in patients diagnosed with epilepsy. Conventional signal decomposition methods, such as the fast Fourier transform, widely used in signal analysis based on time series techniques, have some issues when analyzing nonlinear and non-stationary signals, in addition to difficulties in detecting low-order frequencies.
Results: To overcome these limitations, alternatives such as empirical mode decomposition and one of its variants, called ensemble empirical mode decomposition, have been developed. These techniques allow observing different oscillation modes through intrinsic mode functions and instantaneous frequencies.
Conclusions: In this study, the results obtained through the aforementioned techniques were compared, revealing the impact of nonlinear methods on the reconstruction of brain activity.
Abstract (es)
Contexto: La epilepsia es una enfermedad neurológica que afecta a más de 50 millones de personas en todo el mundo, provocando convulsiones recurrentes, con un impacto significativo en la calidad de vida de los pacientes debido a actividad neuronal anormalmente sincronizada.
Métodos: Este artículo analiza tres métodos empleados para el análisis de señales en pacientes diagnosticados con epilepsia. Los métodos de descomposición de señales convencionales, como la transformada rápida de Fourier, ampliamente utilizada en el análisis de señales basado en técnicas de series de tiempo, presentan algunos problemas al analizar señales no lineales y no estacionarias, así como dificultades para detectar frecuencias de bajo orden.
Resultados: Para superar estas limitaciones, se han desarrollado alternativas como la descomposición empírica de modos y una de sus variantes, llamada descomposición modal empírica de conjunto. Estas técnicas permiten observar diferentes modos de oscilación mediante las funciones de modo intrínseco y las frecuencias instantáneas.
Conclusiones: En este estudio se compararon los resultados obtenidos mediante las técnicas mencionadas, revelando el impacto de los métodos no lineales en la reconstrucción de la actividad cerebral.
References
R. S. Fisher et al., "Ilae official report: a practical clinical definition of epilepsy," Epilepsia, vol. 55, no. 4, pp. 475-482, 2014. https://doi.org/10.1111/epi.12550
A. T. Tzallas et al., "Automated epileptic seizure detection methods: a review study," Epilepsy-Histol. Electroencephalogr. Psychol. Asp., pp. 2027-2036, 2012. https://doi.org/10.5772/31597
K. M. Fiest et al., "Prevalence and incidence of epilepsy: a systematic review and meta-analysis of international studies," Neurology, vol. 88, no. 3, pp. 296-303, 2017. https://doi.org/10.1212/WNL.0000000000003509
S. Roy, I. Kiral-Kornek, and S. Harrer, "A deep recurrent neural network for abnormal eeg identification," in Proc. 17th Conf. Artif. Intell. Med (AIME), Poznan, Poland, Jun 26-29, 2019, pp. 47-56. https://doi.org/10.1007/978-3-030-21642-9_8
L.-D. Guerrero, L. D. Romero, and M. Bueno-Lopez, "A review of epileptic seizure detection using eeg signals analysis in the time and frequency domain," in 2021 IEEE 21st Int. Conf. Commun. Technol. (ICCT), 2021, pp. 1363-1367. https://doi.org/10.1109/ICCT52962.2021.9657835
O. Karabiber Cura, S. Kocaaslan Atli, H. S. Ture, and A. Akan, "Epileptic seizure classifications using empirical mode decomposition and its derivative," Biomed. eng., vol. 19, pp. 1-22, 2020. https://doi.org/10.1186/s12938-020-0754-y
P. A. Munoz, E. Giraldo, M. B. Lopez, and M. Molinas, "Automatic selection of frequency bands for electroencephalographic source localization," in Proc. 2019 9th Int. IEEE/EMBS Conf. on Neural Eng. (NER), 2019, pp. 1179-1182. https://doi.org/10.1109/NER.2019.8716979
J. Engel Jr, "Evolution of concepts in epilepsy surgery," Epileptic Disorders, vol. 21, no. 5, pp. 391-409, 2019. https://doi.org/10.1684/epd.2019.1091
E. Ceballos Dominguez, M. Subathra, N. Sairamya, and S. Thomas George, "Detection of focal epilepsy in brain maps through a novel pattern recognition technique," Neur. Comput. and Appl., vol. 32, pp. 10 143-10 157, 2020. https://doi.org/10.1007/s00521-019-04544-8
N. van Klink et al., "Simultaneous meg and eeg to detect ripples in people with focal epilepsy," Clin. Neuroph., vol. 130, no. 7, pp. 1175-1183, 2019. https://doi.org/10.1016/j.clinph.2019.01.027
G. Zazzaro, S. Cuomo, A. Martone, R. V. Montaquila, G. Toraldo, and L. Pavone, "Eeg signal analysis for epileptic seizures detection by applying data mining techniques," IoT, vol. 14, p. 100048, 2021. https://doi.org/10.1016/j.iot.2019.03.002
S. Kulaseharan, A. Aminpour, M. Ebrahimi, and E. Widjaja, "Identifying lesions in paediatric epilepsy using morphometric and textural analysis of magnetic resonance images," NeuroImage: Clinical, vol. 21, p. 101663, 2019. https://doi.org/10.1016/j.nicl.2019.101663
L. A. Moctezuma and M. Molinas, "Eeg channel-selection method for epileptic-seizure classification based on multi-objective optimization," Front. Neurosci., vol. 14, p. 593, 2020. https://doi.org/10.3389/fnins.2020.00593
F. McLoughlin, A. Duffy, and M. Conlon, "Evaluation of time series techniques to characterise domestic electricity demand," Energy, vol. 50, pp. 120-130, 2013. https://doi.org/10.1016/j.energy.2012.11.048
E. Cano, R. Salcedo, and G. Soto, "Analisis de principios y aplicaciones de la transformada wavelet," Universidad Nacional de Catamarca, 2010.
J. Olbrys and M. Mursztyn, "Measuring stock market resiliency with discrete fourier transform for high frequency data," Physica A, vol. 513, pp. 248-256, 2019. https://doi.org/10.1016/j.physa.2018.09.028
M. Sanabria-Villamizar, M. Bueno-Lopez, J. C. Hernandez, and D. Vera, "Characterization of household-consumption load profiles in the time and frequency domain," Int. J. Electr. Power Energy Syst., vol. 137, p. 107756, 2022. https://doi.org/10.1016/j.ijepes.2021.107756
R. Sharma, R. B. Pachori, and S. Gautam, "Empirical mode decomposition based classification of focal and non-focal eeg signals," in Proc. 2014 Int. Conf. Med. Biometrics, 2014, pp. 135-140. https://doi.org/10.1109/ICMB.2014.31
M. A. Colominas, G. Schlotthauer, P. Flandrin, and M. E. Torres, "Descomposicion empirica en modos por conjuntos completa con ruido adaptativo y aplicaciones biomedicas," in Proc. XVIII Cong Arg. Bioing. y VII Jorn. Ing. Clinica, Mar del Plata, Argentina, 2011.
Y. Lei, J. Lin, Z. He, and M. J. Zuo, "A review on empirical mode decomposition in fault diagnosis of rotating machinery," Mech. Syst. Signal Process, vol. 35, no. 1-2, pp. 108-126, 2013. https://doi.org/10.1016/j.ymssp.2012.09.015
O. B. Fosso and M. Molinas, "Emd mode mixing separation of signals with close spectral proximity in smart grids," in Proc. 2018 IEEE PES innovat. smart grid technolog. conf. (ISGT-Europe), 2018, pp. 1-6. https://doi.org/10.1109/ISGTEurope.2018.8571816
M. Bueno-Lopez, M. Molinas, and G. Kulia, "Understanding instantaneous frequency detection: A discussion of hilbert-huang transform versus wavelet transform," in Proc. Int. Work-Conf. Time Ser. Anal.-ITISE, vol. 1, 2017, pp. 474-486.
G. L. Goldberger AL, Amaral LAN, "Physiobank, physiotoolkit, and physionet: Components of a new research resource for complex physiologic signals, 2000. https://doi.org/10.1161/01.CIR.101.23.e215
C. Gomez, P. Arbelaez, M. Navarrete, C. Alvarado-Rojas, M. Le Van Quyen, and M. Valderrama, "Automatic seizure detection based on imaged-eeg signals through fully convolutional networks," Sci. Rep., vol. 10, no. 1, pp. 1-13, 2020. https://doi.org/10.1038/s41598-020-78784-3
J. Malmivuo and R. Plonsey, "Bioelectromagn. EEG," Jan. 1995, pp. 247-264.
A. H. Shoeb and J. V. Guttag, "Application of machine learning to epileptic seizure detection," in Proc. ICML, 2010.
M. A. Rodriguez, J. F. Sotomonte, J. Cifuentes, and M. Bueno-Lopez, "A classification method for power-quality disturbances using hilbert-huang transform and lstm recurrent neural networks," J. Electr. Eng. Technol., vol. 16, pp. 249-266, 2021. https://doi.org/10.1007/s42835-020-00612-5
M. Uyar, S. Yildirim, and M. T. Gencoglu, "An expert system based on s-transform and neural network for automatic classification of power quality disturbances," Expert Syst. Appl., vol. 36, no. 3, pp. 5962-5975, 2009. https://doi.org/10.1016/j.eswa.2008.07.030
F. Series, "Fourier series & fourier transforms," BioMed. Eng., 2003.
M. Zabihi, S. Kiranyaz, A. B. Rad, A. K. Katsaggelos, M. Gabbouj, and T. Ince, "Analysis of high-dimensional phase space via poincare section for patient-specific seizure detection," IEEE Trans. Neural Syst. Rehabil. Eng., vol. 24, no. 3, pp. 386-398, 2015. https://doi.org/10.1109/TNSRE.2015.2505238
N. E. Huang, Hilbert-Huang transform and its appl. World Scientific, 2014, vol. 16. https://doi.org/10.1142/8804
N. E. Huang et al., "The empirical mode decomposition and the hilbert spectrum for nonlinear and non-stationary time series analysis," Proc. R. Soc. Lond. A math. Phys. Eng Sci, vol. 454, no. 1971, pp. 903-995, 1998. https://doi.org/10.1098/rspa.1998.0193
P. N. Jadhav, D. Shanamugan, A. Chourasia, A. R. Ghole, A. Acharyya, and G. Naik, "Automated detection and correction of eye blink and muscular artefacts in eeg signal for analysis of autism spectrum disorder," in Proc. 2014 36th Annu. Int. Conf. IEEE Eng. Med. Biol. Soc., 2014, pp. 1881-1884. https://doi.org/10.1109/EMBC.2014.6943977
M. Peel, G. Pegram, and T. McMahon, "Empirical mode decomposition: improvement and application," in Proc. Int. Congress Model. Simul., vol. 1. Citeseer, 2007, pp. 2996-3002.
M. Bueno-Lopez, M. Sanabria-Villamizar, M. Molinas, and E. Bernal-Alzate, "Oscillation analysis of low-voltage distribution systems with high penetration of photovoltaic generation," Electr. Eng., vol. 103, no. 2, pp. 1141-1154, 2021. https://doi.org/10.1007/s00202-020-01152-x
C. Rehtanz, Y. Li, Y. Cao, and D. Yang, "Interconnected power systems: Wide-area dynamic monitoring and control applications," 2016.
Z. Wu and N. E. Huang, "Ensemble empirical mode decomposition: a noise-assisted data analysis method," Adv. Adapt. Data Anal., vol. 1, no. 01, pp. 1-41, 2009. https://doi.org/10.1142/S1793536909000047
A. R. Messina, V. Vittal, G. T. Heydt, and T. J. Browne, "Nonstationary approaches to trend identification and denoising of measured power system oscillations," IEEE Trans. Power Syst., vol. 24, no. 4, pp. 1798-1807, 2009. https://doi.org/10.1109/TPWRS.2009.2030419
How to Cite
APA
ACM
ACS
ABNT
Chicago
Harvard
IEEE
MLA
Turabian
Vancouver
Download Citation
License
Copyright (c) 2024 Leandro Dorado-Romero, Maximiliano Bueno-López, Jenny Alexandra Cifuentes

This work is licensed under a Creative Commons Attribution-NonCommercial-ShareAlike 4.0 International License.
From the edition of the V23N3 of year 2018 forward, the Creative Commons License "Attribution-Non-Commercial - No Derivative Works " is changed to the following:
Attribution - Non-Commercial - Share the same: this license allows others to distribute, remix, retouch, and create from your work in a non-commercial way, as long as they give you credit and license their new creations under the same conditions.